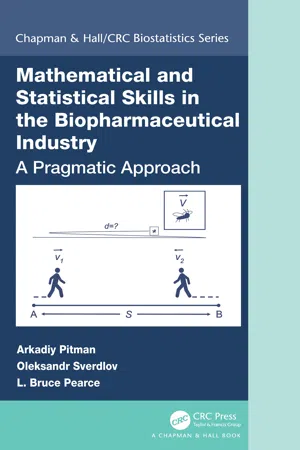
eBook - ePub
Mathematical and Statistical Skills in the Biopharmaceutical Industry
A Pragmatic Approach
Arkadiy Pitman, Oleksandr Sverdlov, L. Bruce Pearce
This is a test
Compartir libro
- 220 páginas
- English
- ePUB (apto para móviles)
- Disponible en iOS y Android
eBook - ePub
Mathematical and Statistical Skills in the Biopharmaceutical Industry
A Pragmatic Approach
Arkadiy Pitman, Oleksandr Sverdlov, L. Bruce Pearce
Detalles del libro
Vista previa del libro
Índice
Citas
Información del libro
Mathematical and Statistical Skills in the Biopharmaceutical Industry: A Pragmatic Approach describes a philosophy of efficient problem solving showcased using examples pertinent to the biostatistics function in clinical drug development. It was written to share a quintessence of the authors' experiences acquired during many years of relevant work in the biopharmaceutical industry. The book will be useful will be useful for biopharmaceutical industry statisticians at different seniority levels and for graduate students who consider a biostatistics-related career in this industry.
Features:
-
- Describes a system of principles for pragmatic problem solving in clinical drug development.
- Discusses differences in the work of a biostatistician in small pharma and big pharma.
- Explains the importance/relevance of statistical programming and data management for biostatistics and necessity for integration on various levels.
- Describes some useful statistical background that can be capitalized upon in the drug development enterprise.
- Explains some hot topics and current trends in biostatistics in simple, non-technical terms.
- Discusses incompleteness of any system of standard operating procedures, rules and regulations.
- Provides a classification of scoring systems and proposes a novel approach for evaluation of the safety outcome for a completed randomized clinical trial.
- Presents applications of the problem solving philosophy in a highly problematic transfusion field where many investigational compounds have failed.
- Discusses realistic planning of open-ended projects.
Preguntas frecuentes
¿Cómo cancelo mi suscripción?
¿Cómo descargo los libros?
Por el momento, todos nuestros libros ePub adaptables a dispositivos móviles se pueden descargar a través de la aplicación. La mayor parte de nuestros PDF también se puede descargar y ya estamos trabajando para que el resto también sea descargable. Obtén más información aquí.
¿En qué se diferencian los planes de precios?
Ambos planes te permiten acceder por completo a la biblioteca y a todas las funciones de Perlego. Las únicas diferencias son el precio y el período de suscripción: con el plan anual ahorrarás en torno a un 30 % en comparación con 12 meses de un plan mensual.
¿Qué es Perlego?
Somos un servicio de suscripción de libros de texto en línea que te permite acceder a toda una biblioteca en línea por menos de lo que cuesta un libro al mes. Con más de un millón de libros sobre más de 1000 categorías, ¡tenemos todo lo que necesitas! Obtén más información aquí.
¿Perlego ofrece la función de texto a voz?
Busca el símbolo de lectura en voz alta en tu próximo libro para ver si puedes escucharlo. La herramienta de lectura en voz alta lee el texto en voz alta por ti, resaltando el texto a medida que se lee. Puedes pausarla, acelerarla y ralentizarla. Obtén más información aquí.
¿Es Mathematical and Statistical Skills in the Biopharmaceutical Industry un PDF/ePUB en línea?
Sí, puedes acceder a Mathematical and Statistical Skills in the Biopharmaceutical Industry de Arkadiy Pitman, Oleksandr Sverdlov, L. Bruce Pearce en formato PDF o ePUB, así como a otros libros populares de Business y Industria farmaceutica, biotecnologica e sanitaria. Tenemos más de un millón de libros disponibles en nuestro catálogo para que explores.
Información
1
Background and Motivation
As the title suggests, this book will discuss a pragmatic approach to problem solving in modern clinical drug development. We shall emphasize some basic yet powerful principles that can help leverage the biostatistician’s value.
We start this chapter with a general discussion of the philosophy of problem solving (§1.1–1.2), followed by a discussion of mathematics and statistics as important tools for problem solving (§1.3). Furthermore, we give a brief discussion of the modern drug development process (§1.4) and revisit some issues in the application of statistics in evidence-based science (§1.5). A summary of what this book is all about is given in §1.6.
1.1 Pragmatic approach to problem solving
“First, you select the problem you would like to solve, then you list at least ten reasons why this has not been solved. But in picking that problem be sure to analyze it carefully to see that it is worth the effort. It takes just as much effort to solve a useless problem as a useful one. Make sure the game is worth the candle”
Charles F. Kettering “Research is a State of Mind”
One may think that any problem solving is pragmatic by definition—after all, it is the hunt for a practical solution. If we include into the definition of a “practical solution” the decision making on whether you spend time on the problem along with reasoning for such a decision, the words “pragmatic approach” become completely unnecessary. It is interesting that the actual short-term practicality might be unrelated to real pragmatism. For example, the short-term practicality of modern mathematics is highly questionable, but the long-term pragmatism in development of fundamental science is undisputable. One of the greatest philosophers, Stanislaw Lem (who happened to be a famous science fiction writer as well), in his 1964 philosophical tractate Summa Technologiae coined the term “crazy tailor” while describing modern pure mathematics. According to Lem, mathematics is a tailor shop where clothes for any imaginable creatures are made in advance: when these creatures arrive, the clothes are already available.
Now, seriously: the gap between creation of tools and their first applications for pure mathematics has been increasing since the split of pure and applied mathematics, which is now estimated as 2-3 centuries. Simply put, current applications are based on theories developed before 1800, and it is expected that cutting edge theories which pure mathematicians are working on now will be needed only after 2200. Worse still, about 95% of the studied structures might not be used at all. We think that is the main reason why pure mathematicians are not considered to be “truly pragmatic” and most statisticians want to separate themselves from pure mathematicians.
There is an interesting psychological phenomenon: mathematicians are considered to be the best problem solvers but are deemed unpractical. If we rephrase the title from the epigraph, we can say that “Problem Solving is a State of Mind”, and mathematicians, mostly due to their training, are recognized as having it, thus making them superior in this aspect. Perhaps in order to balance this aspect, and to have an opportunity to deny their findings, others have developed a superiority complex on practicality. In fact, pure mathematicians are probably the most practical/pragmatic people in the world, since they constantly have to decide whether “the game is worth the candle” while working on a complex problem (take Grigori Perelman1 as an example). The only “impractical” quality of mathematicians (as well as any true problem solvers) is the pursuit of a problem just for moral satisfaction. Strictly speaking, though, the decision to continue work on a problem “just for fun” could be defended as pragmatical, especially if it is done during one’s leisure time. After all, it is great entertainment, plus you never know whether newly acquired knowledge and/or skills could be useful elsewhere.
1.2 Problem solving skills
“Expertise in one field does not carry over into other fields. But experts often think so. The narrower their field of knowledge the more likely they are to think so.”
Robert A. Heinlein “The Notebooks of Lazarus Long”
_______________
1 The one who proved the Poincaré conjecture (one of the seven Millenium Prize Problems stated by the Clay Mathematics Institute on May 24, 2000).
The quoted character (Lazarus Long) is perhaps one of the most pragmatic persons described in literature. He was created to demonstrate the idea that a combination of common sense, a good brain, proper education and pragmatism is the best recipe for successful problem solving in real life and actually could even serve as a decent substitute for morality and religion. The meaning of the epigraph is pretty clear: highly specialized professionals who limit themselves to narrow fields are useless for anything else.

FIGURE 1.1
Vital parts of a problem solving process
Vital parts of a problem solving process
One important direct consequence of the above quote can be formulated as follows: “Expertise is not transferable, but skills and approach are”. Indeed, real-life problem solving skills and a creative approach can be developed anywhere (in any field)—the bigger the number and diversity of these fields, the stronger the skills are, and the easier application in a new situation is.
The first author’s firm philosophy is that relevance of past experience depends much more on what you have carried over into today’s work rather than on where you have worked.
Let us take a closer look at what is meant by “problem solving”. Figure 1.1 displays elements (may not be all inclusive) which represent vital parts of any problem solving process. Most of the elements are self-explanatory and we shall just add a few clarifying notes. Detection is probably the hardest part. A lot of problems are not solved simply because they are not detected. Feasibility is two-fold, and it is in fact a part of detection: some problems are indeed non-solvable, while others are left unattended because they are considered non-solvable. Analysis (broken down by disciplines) implies that all problems are multidimensional; and yet a successful analysis does not necessarily guarantee a successful synthesis. Simplification (or principle of parsimony) is another critical piece. We have a good company here: Einstein’s take on Occham’s Razor—“As simple as possible, but not simpler.” Going “outside the box” and over-simplification are again two sides of the same coin. Formalization of the task is an art by itself; selection of tools is certainly essential and, in fact, new tools should generally be considered as a last resort (unavoidable evil). Planning and execution of the discovered solution can be a problem in itself; and finally, the effective presentation of results and ability to sell both expertise and solution are very crucial.
By and large, the toolkit displayed in Figure 1.1 is used by any person who has been engaged in problem solving either at work or in everyday situations. While the goal of the current book is to demonstrate how the approach works in clinical drug development, some ideas can be successfully applied in other settings as well.
Let us now look at the requirements for a professional problem solver—a person who does it for living and who is called upon only when a serious problem is detected and all attempts to solve it from the inside have failed. The success drivers are the logic and the logistics. The important prerequisites include understanding the elements and structure of logical thinking, the ability to learn “from scratch” quickly and deeply in an unfamiliar field, and the ability to focus on what is most relevant (accurate assessment of scope, applicability, limitations, precision and practicality). After all, goal-oriented practicality, simplicity, comprehensiveness and acceptability frequently take precedence over the beauty of science.
In any case, either for an amateur or a professional, it is good to remember that problem solving is more than work; it is rather a passion or even a lifestyle. The major difference between casual and professional problemsolving is grounded in the organizational aspects that are necessary for a professional. Theoretically it is possible to be a genius problem solver without any political and organizational skills; however, in practice, such individuals usually have a very hard time in self-realization and, as a rule, remain unknown and unaccepted.
One of the major goals of this book is to discuss not only solutions to the problems, but also attempts for getting acceptance as well. Considering that drug development is far away from pure science, achieving acceptance is, in many cases, one of the most complicated parts of the solution.
1.3 Mathematics versus statistics
“A mathematician believes that 2 − 1 ≠ 0; a statistician is uncertain.”
Anonymous
Broadly speaking, science can be classified into three major categories: natural sciences (the study of natural phenomena), social sciences (the study of human behavior and society), and formal sciences (mathematics). The first two types are empirical sciences in which new knowledge is acquired through observation of certain phenomena. By contrast, in mathematics new results are obtained by proving certain conjectures (theorems) using formal logic, a system of axioms, and previously established results. In the context of empirical sciences, one can distinguish three types of knowledge, in the order of increasing complexity: 1) descriptions of phenomena in terms of observed characteristics of the events; 2) description of association among phenomena; and 3) descriptions of causal relationships among phenomena [88]. Many of scientific activities aim at establishing causal relationships between various phenomena, ideally using mathematical equations.
In natural sciences such as physics or chemistry, mathematics has been a very powerful tool to describe causal relationships and, therefore, enable prediction. The physicist Eugene Wigner (1902–1995) in his 1960 seminal paper [103] wrote:
“The miracle of the appropriateness of the language of mathematics for the formulation of the laws of physics is a wonderful gift which we neither understand nor deserve. We should be grateful for it and hope that it will remain valid in future research and that it will extend, for better or for worse, to our pleasure, even though perhaps also to our bafflement, to wide branches of learning.”
While many physical laws have been successfully described using mathematical models, such models are only approximations (albeit quite accurate) to reality. This is captured in Albert Einstein’s (1879–1955) famous quote:
“As far as the laws of mathematics refer to reality, they are not certain; as far as they are certain, they do not refer to reality.”
In biological and social sciences the formulation of cause-effect relationships is much less straightforward than in natural sciences. There is a substantial random variation among humans with respect to their physiology, environmental and lifestyle factors which makes it difficult, if not impossible, to draw definitive conclusions about observed phenomena. For instance, in epidemiology the goal is to determine how certain risk factors affect the incidence of a disease in a population. Does smoking cause lung cancer? If so, then why are there smokers who do not develop lung cancer in their lifetime? Clearly, there is a strong association between smoking and lung cancer, but the causal link is elusive. As soon as we get away from deterministic relationships, establishing “true” causality is practically impossible.
In 1965, the English statistician Sir Austin Bradford Hill (1897–1991) (who is also known as the designer of the first randomized clinical trial evaluating the use of streptomycin in treating tuberculosis in 1946) formulated nine criteria that can be used to provide epidemiological evidence of a causal relationship between risk factors and disease [56]. These criteria include: 1) strength of association (effect size), 2) consistency of the findings (reproducibility), 3) specificity, 4) temporality (the effect must be observed after the cause, possibly with delay), 5) biological gradient (greater exposure should magnify, or sometimes lessen, the incidence of the disease), 6) plausibility, 7) coherence, 8) experiment (“occasionally it is possible to appeal to experimental evidence”), and 9) analogy. While Bradford Hill’s criteria have been widely used in epidemiology, they are still subject to scientific debate.
The challenges of building mathematical models to describe phenomena in economics, social sciences, and even technology were also acknowledged by the originator of cybernetics Norbert Wiener (1894–1964). In his work “God & Golem, Inc.” [102], the famous researcher wrote:
“The success of mathematical physics led the social scientist to be jealous of its power without quite understanding the intellectual attitudes...