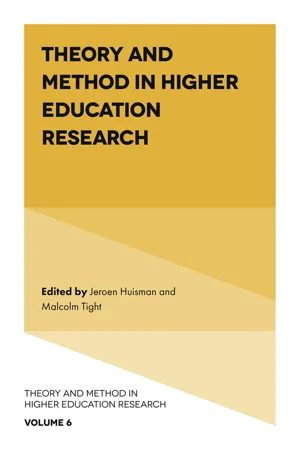
eBook - ePub
Theory and Method in Higher Education Research
This is a test
- 300 pages
- English
- ePUB (mobile friendly)
- Available on iOS & Android
eBook - ePub
Theory and Method in Higher Education Research
Book details
Book preview
Table of contents
Citations
About This Book
Higher education research is a developing field internationally, which is attracting more and more researchers from a great variety of disciplinary backgrounds within and beyond higher education institutions. As such, it is an arena within which a wide range of theories, methods and methodologies are being applied.
This volume of Theory and Method in Higher Education Research contains analyses and discussions of, amongst others, relational working, corpus linguistics, data dialogues, instrumental variables, participatory pedagogy, diverse participation, policy discourse, quality management and knowledge structures of research.
Frequently asked questions
At the moment all of our mobile-responsive ePub books are available to download via the app. Most of our PDFs are also available to download and we're working on making the final remaining ones downloadable now. Learn more here.
Both plans give you full access to the library and all of Perlego’s features. The only differences are the price and subscription period: With the annual plan you’ll save around 30% compared to 12 months on the monthly plan.
We are an online textbook subscription service, where you can get access to an entire online library for less than the price of a single book per month. With over 1 million books across 1000+ topics, we’ve got you covered! Learn more here.
Look out for the read-aloud symbol on your next book to see if you can listen to it. The read-aloud tool reads text aloud for you, highlighting the text as it is being read. You can pause it, speed it up and slow it down. Learn more here.
Yes, you can access Theory and Method in Higher Education Research by Jeroen Huisman, Malcolm Tight, Jeroen Huisman, Malcolm Tight in PDF and/or ePUB format, as well as other popular books in Pedagogía & Educación superior. We have over one million books available in our catalogue for you to explore.
Information
Topic
PedagogíaSubtopic
Educación superiorThe Use of Instrumental Variables in Higher Education Research
Abstract
Higher education researchers are often challenged by the difficulty of empirically validating causal links posited by theories or inferred from correlational observations. The instrumental variable (IV) estimation strategy is one approach that researchers can use to estimate the causal impact of various higher education–related interventions. In this chapter, we discuss how the body of quantitative research specifically devoted to higher education has made use of the IV estimation strategy: we describe how this estimation strategy was used to address causality concerns and provide examples of the types of IVs that were used in various subfields of higher education research. Our discussion is based on a systematic review of a corpus of econometric studies on higher education–related issues that spans the last 30 years. The chapter concludes with a critical discussion of the use of IVs in quantitative higher education research and a discussion of good practices when using an IV estimation strategy.
Keywords: Higher education; education research; quantitative methods; regression analysis; instrumental variables; endogeneity; econometrics
Introduction
A substantial body of research on higher education consists of studies using quantitative approaches: in the years 2000 and 2010, 42% of all journal articles published in 15 academic journals in this field had a predominantly quantitative methodology (Tight, 2013). Quantitative research in the social sciences is commonly challenged by the difficulty of empirically validating causal links posited by theories or inferred from correlational observations (Firebaugh, 2008). Higher education, which can be conceptualized as a complex set of educational interventions that are expected to bring about various individual and societal benefits, is no exception to this methodological challenge.
The difficulty of estimating the causal impact of various educational interventions is partly due to the fact that observational data are more readily available than experimental data, and researchers' inability to observe or measure theoretically important variables introduces a significant source of bias into impact estimates (Schneider, Carnoy, Kilpatrick, Schmidt, & Shavelson, 2007). The bias introduced by such omitted variables is also known as endogeneity bias (Xiqian & Borden, 2019) and can be described as a correlation between the independent variable and the error term in statistical models (Wooldridge, 2003). Several methodological approaches have been developed to address the problem of endogeneity, including fixed effects, instrumental variables (IVs), propensity score matching techniques, difference in differences designs, and regression discontinuity designs (Schneider et al., 2007).
In this chapter, we focus on the use of IVs in addressing the endogeneity problem in quantitative higher education research. The goal of the IV estimation strategy is to reduce bias in parameter estimates that might result from the presence of endogenous variable(s) in statistical models. Adopting an IV estimation strategy means including a variable in the model that can be used to estimate the extent to which the observed correlation between the independent variable(s) and the dependent variable is biased by endogeneity. Such a variable is called an instrument or IV. Including instrument(s) in a statistical model makes researchers' claims about causality stronger compared to correlational models.
In this chapter, we present a systematic review of how the IV estimation strategy was used in a body of econometric research on higher education–related issues. We provide an overview of the IV approach to estimating causal impact and an example from higher education research to illustrate this strategy. We systematically review a corpus of econometric studies published between 1990 and 2019. We report the endogenous dependent and independent variables used in the reviewed studies and provide a critical discussion of the IVs that were used to mitigate endogeneity in relation to these variables. Finally, a critical discussion of the use of IVs in higher education research is offered, highlighting good practices in using an IV estimation strategy.
Instrumental Variable Estimation Strategy in the Social Sciences
A visual representation of the IV estimation strategy can be found in Fig. 1, adapted from Firebaugh (2008). In this path diagram, the problem of endogeneity is represented by the relationships between variables X, Y, and C. C stands for confounders: all individual characteristics and structural differences which are correlated with both the intervention of interest (represented on the diagram by X) and the outcome of interest (represented by Y) and which cannot be measured directly within the current research design. In the presence of confounder(s), X and Y are endogenous. If there is a theoretical or empirical basis to suspect endogeneity, then the statistical correlation between X and Y (represented by β) cannot be interpreted as validation of the causal impact of the independent variable X on dependent variable Y. In such a situation, the presence of an instrument (represented on the diagram by Z) can help mitigate the endogeneity problem and strengthen causal claims (Firebaugh, 2008).

Fig. 1. Instrumental Variable Method for Estimating the Causal Impact of X on Y. Source: Adapted from Firebaugh (2008).
Two assumptions must be true for a variable to function as an instrument. The first assumption is that Z causes X. An IV estimation strategy should only be adopted if Z, X, and Y constitute a causal chain in which Z causes X and X in turn causes Y. The second assumption is that Z is randomly distributed in relation to Y. This means that there can be no direct causal relationship between Z and Y. There can only be an indirect relationship between Y and Z through Z's impact on X. As per the first assumption, α in Fig. 1 represents the causal impact of Z on X. Using an IV estimator means that we divide the correlation of Z and Y (αβ) with the direct impact of Z on X (α), which gives us β. If both assumptions regarding the instrument Z are met, we can interpret β as the causal impact of X on Y (Firebaugh, 2008).
An IV estimation strategy in practice involves fitting a two-stage least squares regression model (Angrist & Pischke, 2009). In the first-stage equation, the endogenous independent variable X is the outcome, and the IV Z is the predictor. Estimating α gives us the predicted values of X. In the second-stage equation, we estimate β by regressing the endogenous dependent variable (Y) not on the observed values of X, but on the predicted values of X that were calculated with the help of instrument Z in the first-stage equation.
IV Estimation Strategy in Higher Education Research: An Example
Parey and Waldinger (2011) set out to estimate the causal impact of study abroad participation on cross-border employment. They studied the population of German university graduates. The intervention was defined as having been enrolled at a university outside of Germany during the student's bachelor studies. The outcome of interest was whether the university graduate worked in a country other than Germany 12 months after completing their bachelor's degree program. Descriptive statistics of the analyzed sample indicated that the university graduates who studied abroad were employed outside of Germany at substantially higher rates (10.2%) than the university graduates who did not study abroad (2.7%).
Parey and Waldinger were concerned that there might be unobserved differences between university graduates who participated in study abroad and those who did not, which might partially explain the differences in the postgraduation mobility of these individuals. In other words, the authors feared that nonrandom assignment (i.e., selection or self-selection) into study abroad participation biased their results. An IV estimation strategy can be used when participation in an intervention is nonrandom, but the incentive to participate in the intervention is randomly assigned (Firebaugh, 2008). In such a scenario, a measure of the incentive can be used as an instrument.
The introduction of dedicated scholarships for international student mobility (such as the Erasmus scholarships in Germany and other European countries) provided an incentive for university students to study abroad by decreasing the direct costs associated with studying in another country. Therefore, Parey and Waldinger argued, students who attended universities where Erasmus scholarships were offered (or where more Erasmus scholarships were offered) might have been more likely to study abroad...
Table of contents
- Cover
- Series Editor
- Title
- Copyright
- Contents
- List of Contributors
- Editorial Introduction
- Theorising Practices of Relational Working across the Boundaries of Higher Education
- Uses of Corpus Linguistics in Higher Education Research: An Adjustable Lens
- Dialogues with Data: Generating Theoretical Insights from Research on Practice in Higher Education
- The Use of Instrumental Variables in Higher Education Research
- Participatory Pedagogy and Artful Inquiry: Partners in Researching the Student Experience
- Rolling Out the Mat: A Talanoa on Talanoa as a Higher Education Research Methodology
- Rethinking Diversity: Combining Sen and Bourdieu to Critically Unpack Higher Education Participation and Persistence
- Deleuzian Approaches to Researching Student Experience in Higher Education
- Investigating Policy Processes and Discourses in Higher Education: The Theoretical Complementarities of Bernstein's Pedagogic Device and Critical Discourse Studies
- Framing Theory for Higher Education Research
- Research into Quality Assurance and Quality Management in Higher Education
- Knowledge with Impact in Higher Education Research