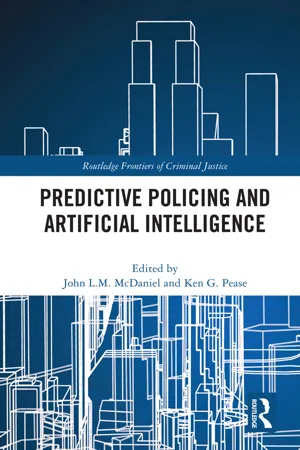
Predictive Policing and Artificial Intelligence
John McDaniel, Ken Pease
- 312 pages
- English
- ePUB (mobile friendly)
- Available on iOS & Android
Predictive Policing and Artificial Intelligence
John McDaniel, Ken Pease
About This Book
This edited text draws together the insights of numerous worldwide eminent academics to evaluate the condition of predictive policing and artificial intelligence (AI) as interlocked policy areas. Predictive and AI technologies are growing in prominence and at an unprecedented rate. Powerful digital crime mapping tools are being used to identify crime hotspots in real-time, as pattern-matching and search algorithms are sorting through huge police databases populated by growing volumes of data in an eff ort to identify people liable to experience (or commit) crime, places likely to host it, and variables associated with its solvability. Facial and vehicle recognition cameras are locating criminals as they move, while police services develop strategies informed by machine learning and other kinds of predictive analytics. Many of these innovations are features of modern policing in the UK, the US and Australia, among other jurisdictions.AI promises to reduce unnecessary labour, speed up various forms of police work, encourage police forces to more efficiently apportion their resources, and enable police officers to prevent crime and protect people from a variety of future harms. However, the promises of predictive and AI technologies and innovations do not always match reality. They often have significant weaknesses, come at a considerable cost and require challenging trade- off s to be made. Focusing on the UK, the US and Australia, this book explores themes of choice architecture, decision- making, human rights, accountability and the rule of law, as well as future uses of AI and predictive technologies in various policing contexts. The text contributes to ongoing debates on the benefits and biases of predictive algorithms, big data sets, machine learning systems, and broader policing strategies and challenges.Written in a clear and direct style, this book will appeal to students and scholars of policing, criminology, crime science, sociology, computer science, cognitive psychology and all those interested in the emergence of AI as a feature of contemporary policing.
Frequently asked questions
Information
Chapter 1
The future of AI in policing
Exploring the sociotechnical imaginaries
Introduction
1. Sociotechnical imaginaries
Our definition pulls together the normativity of the imagination with the materiality of networks … Unlike mere ideas and fashions, sociotechnical imaginaries are collective, durable, capable of being performed; yet they are also temporally situated and culturally particular. …[T]hese imaginaries are at once products of and instruments of the co-production of science, technology, and society in modernity.(2015a: 19)
2. The benefits and risks of AI for society
Definition of AI

The benefits of AI
New techniques of machine learning are spurring unprecedented developments in AI applications. Next-generation robotics promise to transform our manufacturing, infrastructure and agriculture sectors; advances in natural language processing are revolutionising the way clinicians interpret the results of diagnostic tests and treat patients; chatbots and automated assistants are ushering in a new world of communication, analytics and customer service; unmanned autonomous vehicles are changing our capacities for defence, security and emergency response; intelligent financial technologies are establishing a more accountable, transparent and risk-aware financial sector; and autonomous vehicles will revolutionise transport.(2019: 4)
The risks of AI
It is well known, for example, that smart facial recognition technologies have often been inaccurate and can replicate the underlying biases of the human-encoded data they rely upon; that AI relies on data that can and has been exploited for ethically dubious purposes, leading to social injustice and inequality; and that while the impact of AI is often described as ‘revolutionary’ and ‘impending’, there is no guarantee that AI technologies such as autonomous vehicles will have their intended effects, or even that their uptake in society will be inevitable or seamless.(2019: 4)
Technical limitations
ML systems will often break in strange ways, do not provide meaningful explanations, and struggle to transfer to a new domain.(Walsh et al., 2019: 15–16)
NLP still has limitations as demonstrated by the Winograd Schema Challenge, a test of machine intelligence. The Winograd Schema tasks computer programs with answering carefully tailored questions that require common sense reasoning to solve. The results from the first annual Winograd Schema Challenge ranged from the low 30th percentile in answering correctly to the high 50s, suggesting that further research is required to develop systems that can handle such tests. Notably, human subjects were asked the same questions and scored much higher, with an overall average of approximately 90 percent.(2019: 34–35)
Data-driven biases
Intrinsic bias is built-in in the development of the AI system or results from inputs causing permanent change in the system’s structure and rules of operation. … Extrinsic bias derives from a system’s inputs in a way that does not effect a permanent change in the system’s internal structure and rules of operation. The output of such systems might be inaccurate or unfair but the system remains ‘rational’ in that new evidence is capable of correcting the fault.(2019: 178–9)
Most of the advances in NLP over the past decade have been achieved with specific tasks and datasets, which are driven by ever larger datasets. However, NLP is only as good as the data set underlying it. If not appropriately trained, NLP models can accentuate b...
Table of contents
- Cover
- Half Title
- Series Information
- Title Page
- Copyright Page
- Dedication
- Contents
- List of illustrations
- Foreword
- List of contributors
- Introduction
- Part I Bias and Big Data
- Part II Police accountability and human rights
- Index