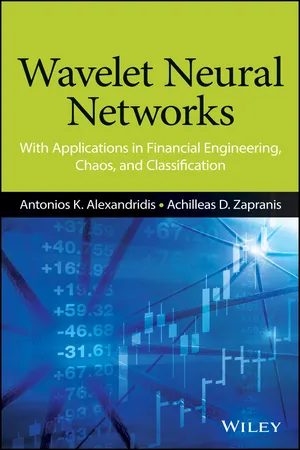
Wavelet Neural Networks
With Applications in Financial Engineering, Chaos, and Classification
Antonios K. Alexandridis, Achilleas D. Zapranis
- English
- ePUB (handyfreundlich)
- Über iOS und Android verfügbar
Wavelet Neural Networks
With Applications in Financial Engineering, Chaos, and Classification
Antonios K. Alexandridis, Achilleas D. Zapranis
Über dieses Buch
A step-by-step introduction to modeling, training, and forecasting using wavelet networks
Wavelet Neural Networks: With Applications in Financial Engineering, Chaos, and Classification presents the statistical model identification framework that is needed to successfully apply wavelet networks as well as extensive comparisons of alternate methods. Providing a concise and rigorous treatment for constructing optimal wavelet networks, the book links mathematical aspects of wavelet network construction to statistical modeling and forecasting applications in areas such as finance, chaos, and classification.
The authors ensure that readers obtain a complete understanding of model identification by providing in-depth coverage of both model selection and variable significance testing. Featuring an accessible approach with introductory coverage of the basic principles of wavelet analysis, Wavelet Neural Networks: With Applications in Financial Engineering, Chaos, and Classification also includes:
• Methods that can be easily implemented or adapted by researchers, academics, and professionals in identification and modeling for complex nonlinear systems and artificial intelligence
• Multiple examples and thoroughly explained procedures with numerous applications ranging from financial modeling and financial engineering, time series prediction and construction of confidence and prediction intervals, and classification and chaotic time series prediction
• An extensive introduction to neural networks that begins with regression models and builds to more complex frameworks
• Coverage of both the variable selection algorithm and the model selection algorithm for wavelet networks in addition to methods for constructing confidence and prediction intervals
Ideal as a textbook for MBA and graduate-level courses in applied neural network modeling, artificial intelligence, advanced data analysis, time series, and forecasting in financial engineering, the book is also useful as a supplement for courses in informatics, identification and modeling for complex nonlinear systems, and computational finance. In addition, the book serves as a valuable reference for researchers and practitioners in the fields of mathematical modeling, engineering, artificial intelligence, decision science, neural networks, and finance and economics.
Häufig gestellte Fragen
Information
1
Machine Learning and Financial Engineering
Financial Engineering
Inhaltsverzeichnis
- Cover
- Titlepage
- Copyright
- Dedication
- Preface
- Chapter 1: Machine Learning and Financial Engineering
- Chapter 2: Neural Networks
- Chapter 3: Wavelet Neural Networks
- Chapter 4: Model Selection: Selecting the Architecture of the Network
- Chapter 5: Variable Selection: Determining the Explanatory Variables
- Chapter 6: Model Adequacy: Determining a Network's Future Performance
- Chapter 7: Modeling Uncertainty: From Point Estimates to Prediction Intervals
- Chapter 8: Modeling Financial Temperature Derivatives
- Chapter 9: Modeling Financial Wind Derivatives
- Chapter 10: Predicting Chaotic Time Series
- Chapter 11: Classification of Breast Cancer Cases
- Index
- End User License Agreement