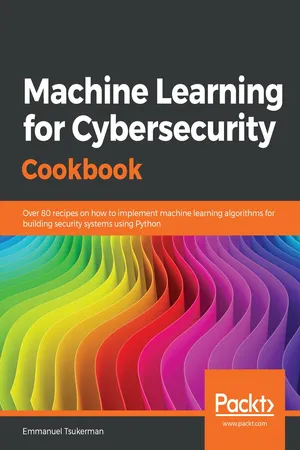
Machine Learning for Cybersecurity Cookbook
Over 80 recipes on how to implement machine learning algorithms for building security systems using Python
Emmanuel Tsukerman
- 346 Seiten
- English
- ePUB (handyfreundlich)
- Über iOS und Android verfügbar
Machine Learning for Cybersecurity Cookbook
Over 80 recipes on how to implement machine learning algorithms for building security systems using Python
Emmanuel Tsukerman
Über dieses Buch
Learn how to apply modern AI to create powerful cybersecurity solutions for malware, pentesting, social engineering, data privacy, and intrusion detection
Key Features
- Manage data of varying complexity to protect your system using the Python ecosystem
- Apply ML to pentesting, malware, data privacy, intrusion detection system(IDS) and social engineering
- Automate your daily workflow by addressing various security challenges using the recipes covered in the book
Book Description
Organizations today face a major threat in terms of cybersecurity, from malicious URLs to credential reuse, and having robust security systems can make all the difference. With this book, you'll learn how to use Python libraries such as TensorFlow and scikit-learn to implement the latest artificial intelligence (AI) techniques and handle challenges faced by cybersecurity researchers.
You'll begin by exploring various machine learning (ML) techniques and tips for setting up a secure lab environment. Next, you'll implement key ML algorithms such as clustering, gradient boosting, random forest, and XGBoost. The book will guide you through constructing classifiers and features for malware, which you'll train and test on real samples. As you progress, you'll build self-learning, reliant systems to handle cybersecurity tasks such as identifying malicious URLs, spam email detection, intrusion detection, network protection, and tracking user and process behavior. Later, you'll apply generative adversarial networks (GANs) and autoencoders to advanced security tasks. Finally, you'll delve into secure and private AI to protect the privacy rights of consumers using your ML models.
By the end of this book, you'll have the skills you need to tackle real-world problems faced in the cybersecurity domain using a recipe-based approach.
What you will learn
- Learn how to build malware classifiers to detect suspicious activities
- Apply ML to generate custom malware to pentest your security
- Use ML algorithms with complex datasets to implement cybersecurity concepts
- Create neural networks to identify fake videos and images
- Secure your organization from one of the most popular threats – insider threats
- Defend against zero-day threats by constructing an anomaly detection system
- Detect web vulnerabilities effectively by combining Metasploit and ML
- Understand how to train a model without exposing the training data
Who this book is for
This book is for cybersecurity professionals and security researchers who are looking to implement the latest machine learning techniques to boost computer security, and gain insights into securing an organization using red and blue team ML. This recipe-based book will also be useful for data scientists and machine learning developers who want to experiment with smart techniques in the cybersecurity domain. Working knowledge of Python programming and familiarity with cybersecurity fundamentals will help you get the most out of this book.
Häufig gestellte Fragen
Information
Automatic Intrusion Detection
- Spam filtering using machine learning
- Phishing URL detection
- Capturing network traffic
- Network behavior anomaly detection
- Botnet traffic detection
- Feature engineering for insider threat detection
- Employing anomaly detection for insider threats
- Detecting DDoS
- Credit card fraud detection
- Counterfeit bank note detection
- Ad blocking using machine learning
- Wireless indoor localization
Technical requirements
- Wireshark
- PyShark
- costcla
- scikit-learn
- pandas
- NumPy
Spam filtering using machine learning
Getting ready
pip install sklearn
How to do it...
- Unzip the spamassassin-public-corpus.7z dataset.
- Specify the path of your spam and ham directories:
import os
spam_emails_path = os.path.join("spamassassin-public-corpus", "spam")
ham_emails_path = os.path.join("spamassassin-public-corpus", "ham")
labeled_file_directories = [(spam_emails_path, 0), (ham_emails_path, 1)]
- Create labels for the two classes and read the emails into a corpus:
email_corpus = []
labels = []
for class_files, label in labeled_file_directories:
files = os.listdir(class_files)
for file in files:
file_path = os.path.join(class_files, file)
try:
with open(file_path, "r") as currentFile:
email_content = currentFile.read().replace("\n", "")
email_content = str(email_content)
email_corpus.append(email_content)
labels.append(label)
except:
pass
- Train-test split the dataset:
from sklearn.model_selection import train_test_split
X_train, X_test, y_train, y_test = train_test_split(
email_corpus, labels, test_size=0.2, random_state=11
)
- Train an NLP pipeline on the training data:
from sklearn.pipeline import Pipeline
from sklearn.feature_extraction.text import HashingVectorizer, TfidfTransformer
from sklearn import tree
nlp_followed_by_dt = Pipeline(
[
("vect", HashingVectorizer(input="content", ngram_range=(1, 3))),
("tfidf", TfidfTransformer(use_idf=True,)),
("dt", tree.DecisionTreeClassifier(class_weight="balanced")),
]
)
nlp_followed_by_dt.fit(X_train, y_train)
- Evaluate the classifier on the testing data:
from sklearn.metrics import accuracy_score, confusion_matrix
y_test_pred = nlp_followed_by_dt.predict(X_test)
print(accuracy_score(y_test, y_test_pred))
print(confusion_matrix(y_test, y_test_pred))
0.9761620977353993
[[291 7]
[ 13 528]]
How it works…
Phishing URL detection
Inhaltsverzeichnis
- Title Page
- Copyright and Credits
- About Packt
- Contributors
- Preface
- Machine Learning for Cybersecurity
- Machine Learning-Based Malware Detection
- Advanced Malware Detection
- Machine Learning for Social Engineering
- Penetration Testing Using Machine Learning
- Automatic Intrusion Detection
- Securing and Attacking Data with Machine Learning
- Secure and Private AI
- Appendix
- Other Books You May Enjoy