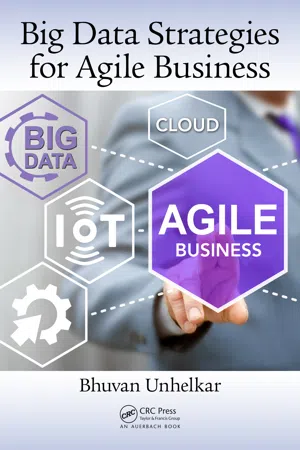
eBook - ePub
Big Data Strategies for Agile Business
Bhuvan Unhelkar
This is a test
- 503 Seiten
- English
- ePUB (handyfreundlich)
- Über iOS und Android verfügbar
eBook - ePub
Big Data Strategies for Agile Business
Bhuvan Unhelkar
Angaben zum Buch
Buchvorschau
Inhaltsverzeichnis
Quellenangaben
Über dieses Buch
Agile is a set of values, principles, techniques, and frameworks for the adaptable, incremental, and efficient delivery of work. Big Data is a rapidly growing field that encompasses crucial aspects of data such as its volume, velocity, variety, and veracity. This book outlines a strategic approach to Big Data that will render a business Agile. It discusses the important competencies required to streamline and focus on the analytics and presents a roadmap for implementing such analytics in business.
Häufig gestellte Fragen
Wie kann ich mein Abo kündigen?
Gehe einfach zum Kontobereich in den Einstellungen und klicke auf „Abo kündigen“ – ganz einfach. Nachdem du gekündigt hast, bleibt deine Mitgliedschaft für den verbleibenden Abozeitraum, den du bereits bezahlt hast, aktiv. Mehr Informationen hier.
(Wie) Kann ich Bücher herunterladen?
Derzeit stehen all unsere auf Mobilgeräte reagierenden ePub-Bücher zum Download über die App zur Verfügung. Die meisten unserer PDFs stehen ebenfalls zum Download bereit; wir arbeiten daran, auch die übrigen PDFs zum Download anzubieten, bei denen dies aktuell noch nicht möglich ist. Weitere Informationen hier.
Welcher Unterschied besteht bei den Preisen zwischen den Aboplänen?
Mit beiden Aboplänen erhältst du vollen Zugang zur Bibliothek und allen Funktionen von Perlego. Die einzigen Unterschiede bestehen im Preis und dem Abozeitraum: Mit dem Jahresabo sparst du auf 12 Monate gerechnet im Vergleich zum Monatsabo rund 30 %.
Was ist Perlego?
Wir sind ein Online-Abodienst für Lehrbücher, bei dem du für weniger als den Preis eines einzelnen Buches pro Monat Zugang zu einer ganzen Online-Bibliothek erhältst. Mit über 1 Million Büchern zu über 1.000 verschiedenen Themen haben wir bestimmt alles, was du brauchst! Weitere Informationen hier.
Unterstützt Perlego Text-zu-Sprache?
Achte auf das Symbol zum Vorlesen in deinem nächsten Buch, um zu sehen, ob du es dir auch anhören kannst. Bei diesem Tool wird dir Text laut vorgelesen, wobei der Text beim Vorlesen auch grafisch hervorgehoben wird. Du kannst das Vorlesen jederzeit anhalten, beschleunigen und verlangsamen. Weitere Informationen hier.
Ist Big Data Strategies for Agile Business als Online-PDF/ePub verfügbar?
Ja, du hast Zugang zu Big Data Strategies for Agile Business von Bhuvan Unhelkar im PDF- und/oder ePub-Format sowie zu anderen beliebten Büchern aus Commerce & Gestion de l'information. Aus unserem Katalog stehen dir über 1 Million Bücher zur Verfügung.
Information
Chapter 3
Data Science—Analytics, Context, and Strategies
Chapter Objectives






This chapter is mainly based on the second module of the Big Data Framework for Agile Business (BDFAB): data science—analytics, context, and technology. Specifically, as shown in Figure 2.1, this module draws attention to data, its various types and categories, and their utilization in analytics. Setting the context of a data point and the role of hex elementization in doing so is also discussed in this chapter. Furthermore, this chapter explains the crucial importance of granularity in data and analytics, and the setting of the OGL. Data science is presented as a discipline responsible for adopting and using Big Data in an iterative and incremental manner.
Data Science: Analytics, Context, and Strategies
Understanding the Importance of Data Science
Data science is a broad-ranging term that represents the technologies and analytics of Big Data. Additionally, though, data science can be understood as a discipline of utilizing technologies and analytics to convert data into actionable knowledge. Data science includes data mining, analytics (statistics), process modeling, machine learning (ML), parallel processing, and associated aspects of data management. The application of analytics to this data is the main step in arriving at insights. Therefore, data analytics remains at the core of data science. Data science, however, is closer to the business leadership and strategic decision making than data analytics. The evolution of data to actionable knowledge requires a specialist discipline that includes the study of data, its characteristics, its context in analytics, and eventually its value in business agility. These aforementioned activities require a wide coverage of various other disciplines within the organization and collaboration with many cross-functional teams. Therefore, the work of data science is interdisciplinary.
While analysis of data can focus on using the statistical expertise and management of data on the technical aspects, data science focuses on the strategic aspect of producing business value from data. This strategic aspect of data science requires domain knowledge of the industry where it is applied. For example, domain knowledge of the banking, finance, insurance, telecom, medical, and education industries is important in developing corresponding data strategies. Consider the following examples where data science provides value by combining the science of data with the domain knowledge:








Data science thus has wide-ranging applications in business decision making. Many internal organizational disciplines and functions provide input into data science. These are the disciplines of business strategies, project management, enterprise architecture, process modeling, solutions development, and quality assurance and testing. These disciplines complement those of data science. Figure 3.1 shows the effort involved in categorizing data, finding the correlation, undertaking analytics, and presenting the insights in an easy-to-use way for the end user.
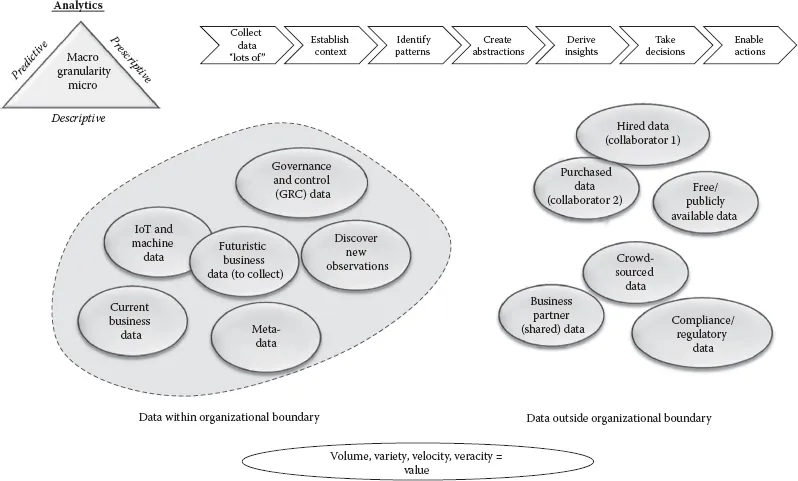
Figure 3.1Data analytics, data categories (pools), and a subprocess for data transformation.
Data science considers the following in order to provide business value:




Inhaltsverzeichnis
- Cover
- Half-Title
- Title
- Copyright
- Dedication
- Series
- Contents
- List of Figures
- List of Tables
- Foreword
- Preface
- Acknowledgements
- About the Author
- Domain Terms and Acronyms
- SECTION I INTRODUCTION TO BIG DATA STRATEGIES AND OUTLINE OF BIG DATA FRAMEWORK FOR AGILE BUSINESS (BDFAB)
- SECTION II ANALYTICS, PROCESSES, TECHNOLOGIES, ARCHITE CTURE, AND DATABASES WIT HIN THE BDFAB
- SECTION III QUALITY, GRC, PEOPLE AND THEIR UPSKILLING, AND AGILE BUSINESS WIT HIN THE BDFAB
- SECTION IV CASE STUDIES IN BANKING, HEALTH, AND EDUCATION
Zitierstile für Big Data Strategies for Agile Business
APA 6 Citation
Unhelkar, B. (2017). Big Data Strategies for Agile Business (1st ed.). CRC Press. Retrieved from https://www.perlego.com/book/1515920/big-data-strategies-for-agile-business-pdf (Original work published 2017)
Chicago Citation
Unhelkar, Bhuvan. (2017) 2017. Big Data Strategies for Agile Business. 1st ed. CRC Press. https://www.perlego.com/book/1515920/big-data-strategies-for-agile-business-pdf.
Harvard Citation
Unhelkar, B. (2017) Big Data Strategies for Agile Business. 1st edn. CRC Press. Available at: https://www.perlego.com/book/1515920/big-data-strategies-for-agile-business-pdf (Accessed: 14 October 2022).
MLA 7 Citation
Unhelkar, Bhuvan. Big Data Strategies for Agile Business. 1st ed. CRC Press, 2017. Web. 14 Oct. 2022.