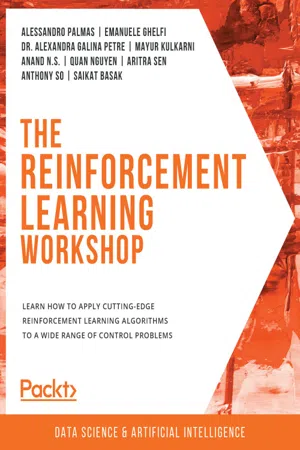
The Reinforcement Learning Workshop
Alessandro Palmas, Emanuele Ghelfi, Dr. Alexandra Galina Petre, Mayur Kulkarni, Anand N.S., Quan Nguyen, Aritra Sen, Anthony So, Saikat Basak
- 822 Seiten
- English
- ePUB (handyfreundlich)
- Über iOS und Android verfügbar
The Reinforcement Learning Workshop
Alessandro Palmas, Emanuele Ghelfi, Dr. Alexandra Galina Petre, Mayur Kulkarni, Anand N.S., Quan Nguyen, Aritra Sen, Anthony So, Saikat Basak
Über dieses Buch
Start with the basics of reinforcement learning and explore deep learning concepts such as deep Q-learning, deep recurrent Q-networks, and policy-based methods with this practical guide
Key Features
- Use TensorFlow to write reinforcement learning agents for performing challenging tasks
- Learn how to solve finite Markov decision problems
- Train models to understand popular video games like Breakout
Book Description
Various intelligent applications such as video games, inventory management software, warehouse robots, and translation tools use reinforcement learning (RL) to make decisions and perform actions that maximize the probability of the desired outcome. This book will help you to get to grips with the techniques and the algorithms for implementing RL in your machine learning models.
Starting with an introduction to RL, you'll be guided through different RL environments and frameworks. You'll learn how to implement your own custom environments and use OpenAI baselines to run RL algorithms. Once you've explored classic RL techniques such as Dynamic Programming, Monte Carlo, and TD Learning, you'll understand when to apply the different deep learning methods in RL and advance to deep Q-learning. The book will even help you understand the different stages of machine-based problem-solving by using DARQN on a popular video game Breakout. Finally, you'll find out when to use a policy-based method to tackle an RL problem.
By the end of The Reinforcement Learning Workshop, you'll be equipped with the knowledge and skills needed to solve challenging problems using reinforcement learning.
What you will learn
- Use OpenAI Gym as a framework to implement RL environments
- Find out how to define and implement reward function
- Explore Markov chain, Markov decision process, and the Bellman equation
- Distinguish between Dynamic Programming, Monte Carlo, and Temporal Difference Learning
- Understand the multi-armed bandit problem and explore various strategies to solve it
- Build a deep Q model network for playing the video game Breakout
Who this book is for
If you are a data scientist, machine learning enthusiast, or a Python developer who wants to learn basic to advanced deep reinforcement learning algorithms, this workshop is for you. A basic understanding of the Python language is necessary.
Häufig gestellte Fragen
Information
1. Introduction to Reinforcement Learning
Introduction
Learning Paradigms
Introduction to Learning Paradigms


Supervised versus Unsupervised versus RL
Inhaltsverzeichnis
- The Reinforcement Learning Workshop
- Preface
- 1. Introduction to Reinforcement Learning
- 2. Markov Decision Processes and Bellman Equations
- 3. Deep Learning in Practice with TensorFlow 2
- 4. Getting Started with OpenAI and TensorFlow for Reinforcement Learning
- 5. Dynamic Programming
- 6. Monte Carlo Methods
- 7. Temporal Difference Learning
- 8. The Multi-Armed Bandit Problem
- 9. What Is Deep Q-Learning?
- 10. Playing an Atari Game with Deep Recurrent Q-Networks
- 11. Policy-Based Methods for Reinforcement Learning
- 12. Evolutionary Strategies for RL
- Appendix