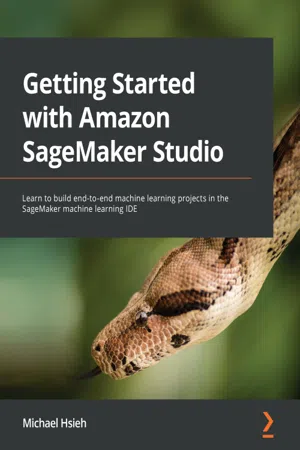
Getting Started with Amazon SageMaker Studio
Michael Hsieh
- 326 Seiten
- English
- ePUB (handyfreundlich)
- Über iOS und Android verfügbar
Getting Started with Amazon SageMaker Studio
Michael Hsieh
Über dieses Buch
Build production-grade machine learning models with Amazon SageMaker Studio, the first integrated development environment in the cloud, using real-life machine learning examples and codeKey Features• Understand the ML lifecycle in the cloud and its development on Amazon SageMaker Studio• Learn to apply SageMaker features in SageMaker Studio for ML use cases• Scale and operationalize the ML lifecycle effectively using SageMaker StudioBook DescriptionAmazon SageMaker Studio is the first integrated development environment (IDE) for machine learning (ML) and is designed to integrate ML workflows: data preparation, feature engineering, statistical bias detection, automated machine learning (AutoML), training, hosting, ML explainability, monitoring, and MLOps in one environment. In this book, you'll start by exploring the features available in Amazon SageMaker Studio to analyze data, develop ML models, and productionize models to meet your goals. As you progress, you will learn how these features work together to address common challenges when building ML models in production. After that, you'll understand how to effectively scale and operationalize the ML life cycle using SageMaker Studio. By the end of this book, you'll have learned ML best practices regarding Amazon SageMaker Studio, as well as being able to improve productivity in the ML development life cycle and build and deploy models easily for your ML use cases.What you will learn• Explore the ML development life cycle in the cloud• Understand SageMaker Studio features and the user interface• Build a dataset with clicks and host a feature store for ML• Train ML models with ease and scale• Create ML models and solutions with little code• Host ML models in the cloud with optimal cloud resources• Ensure optimal model performance with model monitoring• Apply governance and operational excellence to ML projectsWho this book is forThis book is for data scientists and machine learning engineers who are looking to become well-versed with Amazon SageMaker Studio and gain hands-on machine learning experience to handle every step in the ML lifecycle, including building data as well as training and hosting models. Although basic knowledge of machine learning and data science is necessary, no previous knowledge of SageMaker Studio and cloud experience is required.
Häufig gestellte Fragen
Information
Part 1 – Introduction to Machine Learning on Amazon SageMaker Studio
- Chapter 1, Machine Learning and Its Life Cycle in the Cloud
- Chapter 2, Introducing Amazon SageMaker Studio
Chapter 1: Machine Learning and Its Life Cycle in the Cloud
- Understanding ML and its life cycle
- Building ML in the cloud
- Exploring AWS essentials for ML
- Setting up AWS environment
Technical requirements
Understanding ML and its life cycle
An ML life cycle
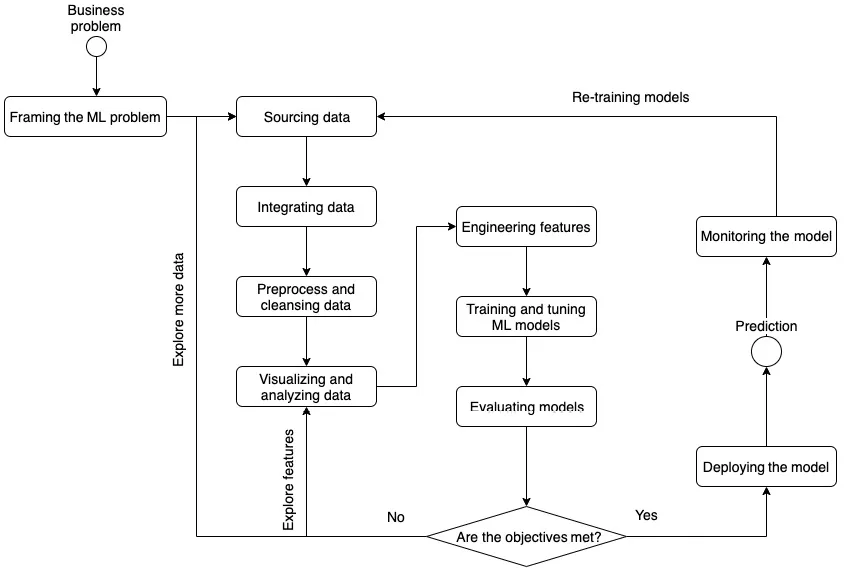
Problem framing
- "What are the key factors to the success of product sales?"
- "Who are the people that are most likely to purchase the product?"
- "What is the bottleneck in throughput in the assembly line?"
- "How do we know whether an item is defective? What differentiates a defective one from a normal one?"
- "How do we measure the conversion?"
- "What are the common characteristics of the consumers who have bought this product?"
Data exploration and engineering
Inhaltsverzeichnis
- Contributors
- Preface
- Part 1 – Introduction to Machine Learning on Amazon SageMaker Studio
- Chapter 1: Machine Learning and Its Life Cycle in the Cloud
- Chapter 2: Introducing Amazon SageMaker Studio
- Part 2 – End-to-End Machine Learning Life Cycle with SageMaker Studio
- Chapter 3: Data Preparation with SageMaker Data Wrangler
- Chapter 4: Building a Feature Repository with SageMaker Feature Store
- Chapter 5: Building and Training ML Models with SageMaker Studio IDE
- Chapter 6: Detecting ML Bias and Explaining Models with SageMaker Clarify
- Chapter 7: Hosting ML Models in the Cloud: Best Practices
- Chapter 8: Jumpstarting ML with SageMaker JumpStart and Autopilot
- Part 3 – The Production and Operation of Machine Learning with SageMaker Studio
- Chapter 9: Training ML Models at Scale in SageMaker Studio
- Chapter 10: Monitoring ML Models in Production with SageMaker Model Monitor
- Chapter 11: Operationalize ML Projects with SageMaker Projects, Pipelines, and Model Registry
- Other Books You May Enjoy