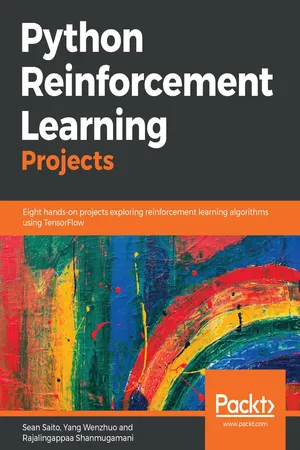
Python Reinforcement Learning Projects
Eight hands-on projects exploring reinforcement learning algorithms using TensorFlow
Sean Saito, Yang Wenzhuo, Rajalingappaa Shanmugamani
- 296 Seiten
- English
- ePUB (handyfreundlich)
- Über iOS und Android verfügbar
Python Reinforcement Learning Projects
Eight hands-on projects exploring reinforcement learning algorithms using TensorFlow
Sean Saito, Yang Wenzhuo, Rajalingappaa Shanmugamani
Über dieses Buch
Implement state-of-the-art deep reinforcement learning algorithms using Python and its powerful libraries
Key Features
- Implement Q-learning and Markov models with Python and OpenAI
- Explore the power of TensorFlow to build self-learning models
- Eight AI projects to gain confidence in building self-trained applications
Book Description
Reinforcement learning is one of the most exciting and rapidly growing fields in machine learning. This is due to the many novel algorithms developed and incredible results published in recent years.
In this book, you will learn about the core concepts of RL including Q-learning, policy gradients, Monte Carlo processes, and several deep reinforcement learning algorithms. As you make your way through the book, you'll work on projects with datasets of various modalities including image, text, and video. You will gain experience in several domains, including gaming, image processing, and physical simulations. You'll explore technologies such as TensorFlow and OpenAI Gym to implement deep learning reinforcement learning algorithms that also predict stock prices, generate natural language, and even build other neural networks.
By the end of this book, you will have hands-on experience with eight reinforcement learning projects, each addressing different topics and/or algorithms. We hope these practical exercises will provide you with better intuition and insight about the field of reinforcement learning and how to apply its algorithms to various problems in real life.
What you will learn
- Train and evaluate neural networks built using TensorFlow for RL
- Use RL algorithms in Python and TensorFlow to solve CartPole balancing
- Create deep reinforcement learning algorithms to play Atari games
- Deploy RL algorithms using OpenAI Universe
- Develop an agent to chat with humans
- Implement basic actor-critic algorithms for continuous control
- Apply advanced deep RL algorithms to games such as Minecraft
- Autogenerate an image classifier using RL
Who this book is for
Python Reinforcement Learning Projects is for data analysts, data scientists, and machine learning professionals, who have working knowledge of machine learning techniques and are looking to build better performing, automated, and optimized deep learning models. Individuals who want to work on self-learning model projects will also find this book useful.
Häufig gestellte Fragen
Information
Learning to Play Go
- Introduction to Go and relevant research in AI
- Overview of AlphaGo and AlphaGo Zero
- The Monte Carlo tree search algorithm
- Implementation of AlphaGo Zero
A brief introduction to Go
Go and other board games
Go and AI research
Inhaltsverzeichnis
- Title Page
- Copyright and Credits
- Packt Upsell
- Contributors
- Preface
- Up and Running with Reinforcement Learning
- Balancing CartPole
- Playing Atari Games
- Simulating Control Tasks
- Building Virtual Worlds in Minecraft
- Learning to Play Go
- Creating a Chatbot
- Generating a Deep Learning Image Classifier
- Predicting Future Stock Prices
- Looking Ahead
- Other Books You May Enjoy