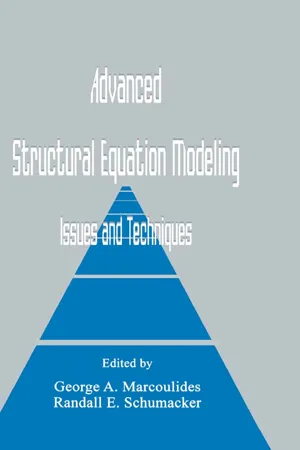
eBook - ePub
Advanced Structural Equation Modeling
Issues and Techniques
George A. Marcoulides,Randall E. Schumacker
This is a test
- 374 páginas
- English
- ePUB (apto para móviles)
- Disponible en iOS y Android
eBook - ePub
Advanced Structural Equation Modeling
Issues and Techniques
George A. Marcoulides,Randall E. Schumacker
Detalles del libro
Vista previa del libro
Índice
Citas
Información del libro
By focusing primarily on the application of structural equation modeling (SEM) techniques in example cases and situations, this book provides an understanding and working knowledge of advanced SEM techniques with a minimum of mathematical derivations. The book was written for a broad audience crossing many disciplines, assumes an understanding of graduate level multivariate statistics, including an introduction to SEM.
Preguntas frecuentes
¿Cómo cancelo mi suscripción?
¿Cómo descargo los libros?
Por el momento, todos nuestros libros ePub adaptables a dispositivos móviles se pueden descargar a través de la aplicación. La mayor parte de nuestros PDF también se puede descargar y ya estamos trabajando para que el resto también sea descargable. Obtén más información aquí.
¿En qué se diferencian los planes de precios?
Ambos planes te permiten acceder por completo a la biblioteca y a todas las funciones de Perlego. Las únicas diferencias son el precio y el período de suscripción: con el plan anual ahorrarás en torno a un 30 % en comparación con 12 meses de un plan mensual.
¿Qué es Perlego?
Somos un servicio de suscripción de libros de texto en línea que te permite acceder a toda una biblioteca en línea por menos de lo que cuesta un libro al mes. Con más de un millón de libros sobre más de 1000 categorías, ¡tenemos todo lo que necesitas! Obtén más información aquí.
¿Perlego ofrece la función de texto a voz?
Busca el símbolo de lectura en voz alta en tu próximo libro para ver si puedes escucharlo. La herramienta de lectura en voz alta lee el texto en voz alta por ti, resaltando el texto a medida que se lee. Puedes pausarla, acelerarla y ralentizarla. Obtén más información aquí.
¿Es Advanced Structural Equation Modeling un PDF/ePUB en línea?
Sí, puedes acceder a Advanced Structural Equation Modeling de George A. Marcoulides,Randall E. Schumacker en formato PDF o ePUB, así como a otros libros populares de Psicologia y Storia e teoria della psicologia. Tenemos más de un millón de libros disponibles en nuestro catálogo para que explores.
Información
CHAPTER ONE
Introduction
California State University, Fullerton
University of North Texas
Structural equation models (SEMs) are used by biologists, educational researchers, market researchers, medical researchers, psychologists, social scientists, and others who traditionally deal with nonexperimental and quasi-experimental data. One reason for the pervasive use in almost every scientific field of study is that SEMs provide researchers with a comprehensive method for the quantification and testing of theories. In fact, it has been suggested that the development of SEM is perhaps the most important and influential statistical revolution to have recently occurred in the scientific arena (Cliff, 1983).
SEM techniques are accepted today as a major component of applied multivariate analysis. The use of the term structural equation modeling is broadly defined to accomodate models that include latent variables, measurement errors in both dependent and independent variables, multiple indicators, reciprocal causation, simultaneity, and interdependence. As implemented in most commercial computer packages (e.g., Amos, EQS, LISREL, LISCOMP, Mx, SAS PROC-CALIS, STATISTICA-SEPATH), the method includes as special cases such procedures as confirmatory factor analysis, multiple regression, path analysis, models for time-dependent data, recursive and nonrecursive models for cross-sectional and longitutinal data, and covariance structure analysis.
The purpose of this volume is to introduce the latest issues and developments in SEM techniques. The goal is to provide an understanding and working knowledge of advanced SEM techniques with a minimum of mathematical derivations. The strategy is to focus primarily on the application of SEM techniques in example cases and situations. We believe that by using this didactic approach, readers will better understand the underlying logic of advanced SEM techniques and, thereby, assess the suitability of the method for their own research. As such, the volume is targeted to a broad audience across multiple disciplines. It is assumed that the reader has mastered the equivalent of a graduate-level multivariate statistics course that included coverage of introductory SEM techniques.
The chapters in this volume provide a timely contribution to the literature on SEM. The theoretical developments and applications of SEMs are burgeoning, and so this volume could have easily become several volumes. Unfortunately, within the limitations of a single volume, only a limited number of topics could be addressed. Each chapter included in this volume was selected to address what we believe are currently the most important issues and developments in SEM. The topics selected include models for multitrait–multimethod (MTMM) matrix analysis, nonlinear structural equation models, multilevel models, cross-domain analyses of change over time, structural time series models, bootstrapping techniques in the analysis of mean and covariance structure, limited information estimators, dealing with incomplete data, problems with equivalent models, and an evaluation of incremental fit indices.
OVERVIEW OF THE VOLUME
Some 35 years ago, Campbell and Fiske (1959) presented the MTMM matrix as a device to assess convergent and divergent validity. Although the qualitative criteria that Campbell and Fiske proposed to judge convergent and divergent validity are quite well known, what is the best method to evaluate quantitatively these two validities is not yet known. In chapter 2, Wothke concentrates on three quantitative approaches to MTMM analysis: confirmatory factor analysis, covariance component analysis, and the direct product model. The three approaches are presented in formal notation and illustrated with worked examples. In addition, model specifications of the worked examples for the Amos (Arbuckle, 1995), LISREL (Jöreskog & Sörbom, 1993), and Mx (Neale, 1994) programs are provided in an appendix. It is shown that covariance component analysis and the direct product model can yield simultaneous estimates of method and trait components. However, there are many cases where these two approaches provide inadmissible solutions or very poor fit. In contrast, the confirmatory factor-analytic approach generally provides admissible solutions but does not yield reliable results when both correlated trait and method factors are included in a measurement design. The chapter concludes by emphasizing the importance of carefully conceptualized MTMM measurement designs.
Models with interaction and nonlinear effects are often encountered in the social and behavioral sciences. In SEM, estimating the interaction effects of latent variables is made possible by using nonlinear and product indicators. In chapter 3, Jöreskog and Yang investigate the interaction effects model proposed by Kenny and Judd (1984) as a general model with nonlinear relationships between latent variables. Using both articificial and empirical data from example cases that involve interactions among latent variables, the authors demonstrate how nonlinear models can be estimated. Model specifications and output using the LISREL program are provided throughout the chapter. The chapter concludes by providing some general guidelines researchers must consider before attempting to model nonlinear relationships among latent variables.
In chapter 4, McArdle and Hamagami present an overview of the multilevel modeling approach from a multiple group SEM perspective. Multilevel models (also referred to as random coefficient, variance components, or hierarchical linear models; Bryk & Raudenbush, 1992) have recently become popular because they permit researchers to deal with estimation problems in complex nested data collection designs. The authors examine the major models of multilevel analysis (i.e., the variance components model, the random coefficients model, the logit model, longitudinal growth models, and multilevel factor models) and attempt to relate these models to standard SEMs for multiple groups. Using examples of multilevel models from their own work on individual differences in human cognitive abilities, the authors illustrate some of the benefits and limitations of the multilevel approach.
In chapter 5, Willett and Sayer demonstrate how the methods of individual growth modeling and covariance structure analysis can be integrated to investigate interindividual differences in change over time. The individual growth-modeling approach uses a pair of hierarchical statistical models to represent two distinct models: (a) individual status as a function of time (the so-called “Level 1” model), and (b) interindividual differences in true change (the so-called “Level 2” model). In the covariance structure approach, these two models can be reformatted as the “measurement” and “structural” components of the general LISREL model with mean structures, and their parameter estimates can be determined. Using longitudinal data drawn from the National Child Development Study (a longitudinal study of all children born in Britain between March 3–9, 1958), Willett and Sayer explain and demonstrate how the new approach can be used to investigate the interrelationships among simultaneous individual changes in two domains (reading and mathematics achievement) over the entire school career, for both healthy children and children with chronic illness. Generalizations of the approach to more than two domains are also discussed, and sample LISREL programs (Jöreskog & Sörbom, 1993) are provided in an appendix.
Time series models attempt to describe the behavior of a process across multiple occasions (Harvey, 1981). To date, time series models have not received extensive usage in the social and behavioral sciences. The most cited reasons for this neglect have been the need to collect data across numerous occasions, and the mathematical complexity of the models (Schmitz, 1990). In chapter 6, Hershberger, Molenaar, and Corneal describe how various time series models can be analyzed using an SEM approach. For each time series model presented, the authors provide a basic description of the model, how the model is analyzed in SEM, and the results of fitting the model to an example data set. In addition, LISREL instructions and model specifications of the example data sets are provided in an appendix.
In chapter 7, Yung and Bentler focus on the application of bootstrapping techniques to covariance structure analysis, of which exploratory and confirmatory factor analysis are considered the leading cases. In general, bootstrapping techniques involve the drawing of random subsamples from an original sample and estimating various parameters from each subsample for the purpose of studying some characteristic of the distribution of these parameter estimates (Efron & Tibshirani, 1993). Using real data, the authors examine the application of bootstrap methods suitable for covariance structure analysis and determine whether the bootstrap “works” for the given situations. The authors also present two new applications of the bootstrap approach to mean and covariance structures.
Estimators for SEMs fall into two classes: full-information and limited-information estimators. Full-information estimators attempt to derive parameter estimates in a model by using all information in the sets of equations simultaneously (e.g., maximum likelihood, generalized least squares, weighted least squares). Limited-information estimators attempt to derive parameter estimates in a model, one equation at a time. Unfortunately, a critical drawback with full-information estimators is that any specification error in the set of equations can bias the estimates throughout the system. In contrast, the use of limited-information estimators results in the bias sometimes being isolated to either one equation or just part of the system. In chapter 8, Bollen presents a two-stage least squares (2SLS) limited-information estimator for structural equation models with latent variables. Using example data, Bollen explains and demonstrates how the proposed 2SLS estimator can be used in various SEMs, including those with heteroscedastic errors.
In chapter 9, Arbuckle discusses the application of maximum likelihood (ML) estimation as an alternative method for handling missing data. Incomplete or missing data are routinely encountered in structural equation modeling, and are even a byproduct from the use of certain designs (e.g., Balanced Incomplete Block, Spiraling Designs; Brown, 1994). To date, a considerable number of approaches for dealing with missing data have been proposed in the literature (Little & Rubin, 1989). The most common approaches are ad hoc adjustments to the data that attempt to remedy the problem either by removal or replacement of values. SEMs are built on the assumption that the covariance matrix follows a Wishart distribution (Brown, 1994; Jöreskog, 1969). As such, complete data are required for the probability density function estimation, and ad hoc adjustments must be made to the data set when there are missing values. Current practice in the handling of missing data for SEMs appears to be dominated by the methods sometimes known as “pairwise deletion” and “listwise deletion.” In Arbuckle’s chapter, two simulations are presented in which the performance of a full-information ML estimation method is compared to the performance of competing missing data techniques. The results demonstrate both the efficiency and the reduced bias of ML estimates. It appears that ML estimation with incomplete data should be the preferred method of treating missing data when the alternatives are pairwise deletion and listwise deletion.
The problem of equivalent models has been known since the earliest developmental stages of SEM. Equivalent models are those that provide identical statistical fit to the data as the hypothesized model but may imply very different substantive interpretations of the data. In chapter 10, Williams, Bozdogan, and Aiman-Smith provide an overview of problems associated with testing equivalent models and propose the use of a novel information complexity approach to assess model fit for equivalent models. Using results of analyses from both simulated and real sample data, the authors demonstrate how to assess model fit among the equivalent models and how substantive conclusions about relationships in models can be impacted when selecting one model from among a set of equivalent models.
In order to assess the goodness of fit of SEMs, researchers generally rely on subjective indices of fit (Bentler, 1990). Although different types of indices have been proposed in the literature, no concensus has been reached as to which are the best (Tanaka, 1993). Currently, the most popular indices are from the family of incremental fit indices (Bollen & Long, 1993). The final chapter by Marsh, Balla, and Hau, examines seven incremental fit indices in relation to their mathematical and empirical properties in a large Monte Carlo study. These seven incremental fit indices are provided as standard output in most commercially available SEM computer packages. The authors recommend that the nonnormed fit index (or its normed counterpart the normed Tucker–Lewis index), and the relative noncentrality index (or its normed counterpart the comparative fit index) should be preferred when evaluating goodness of fit of SEMs. The normed fit index, the incremental fit index, and the relative fit index are not recommended because they are systematically biased by sample size and can inappropriately penalize model complexity.
REFERENCES
Arbuckle, J. L. (1995). Amos for Windows. Analysis of moment structures (Version 3.5). Chicago, IL: Smallwaters.
Bentler, P. M. (1990). Comparative fit indices in structural models. Psychological Bulletin, 107, 238–246.
Bollen, K. A., & Long, J. S. (1993). Introduction. In K. A. Bollen & J. S. Long (Eds.), Testing structural equation models. Newbury Park, CA: Sage.
Brown, R. L. (1994). Efficacy of the indirect approach for estimating structural equation models with missing data: A comparison of five methods. Structural Equation Modeling, 1, 287–316.
Bryk, A. S., & Raudenbush, S. W. (1992). Hierarchical linear models: Applications and data analysis methods. Newbury Park, CA: Sage.
Campbell, D. T., & Fiske, D. W. (1959). Convergent and discriminant validation by the multitrait-multimethod matrix. Psychological Bulletin, 56, 81–105.
Cliff, N. (1983). Some cautions concerning the application of causal modeling methods. Multivariate Behavioral Research, 18, 115–126.
Efron, B., & Tibshirani, R. J. (1993). An introduction to the bootstrap. New York: Chapman & Hall.
Harvey, A. C. (1981). Time-series models. Oxford: Philip Alan.
Jöreskog, K. G. (1969). A general approach to confirmatory maximum likelihood factor analysis. Psychometrika, 34, 183–202.
Jöreskog, K. G., & Sörbom, D. (1993). LISREL 8. Structural equation modeling with the SIMPLIS command language. Chicago, IL: Scientific Software International.
Kenny, D. A., & Judd, C. M. (1984). Estimating the nonlinear and interactive effects of latent variables. Psychological Bulletin, 96, 201–210.
Little, R. J. A., & Rubin, D. B. (1989). The analysis of social science data with missing values. Sociological Methods and Research, 18, 292–326.
Neale, M. C. (1994). Mx: Statistical modeling (2nd ed.). Richmond, VA: Author.
Schmitz, B. (1990). Univariate and multivariate time-series models: The analysis of intraindividual variability and interindividual relationships. In A. Von Eye (Ed.), Statistical methods in longitudinal research (Vol. 2). New York: Academic Press.
Tanaka, J. S. (1993). Multifaceted conceptions of fit in structural equation models. In K. A. Bollen & J. S. Long (Eds.), Testing structural equation models. Newbury Park, CA: Sage.
CHAPTER TWO
Models for Multitrait–Multimethod Matrix Analysis
SmallWaters Corporation, Chicago
The well-known contribution by Campbell and Fiske (1959) presented the multitrait–multimethod (MTMM) matrix forma...
Índice
- Cover
- Half Title
- Title Page
- Copyright Page
- Table of Contents
- Acknowledgments
- 1 Introduction
- 2 Models for Multitrait–Multimethod Matrix Analysis
- 3 Nonlinear Structural Equation Models: The Kenny-Judd Model With Interaction Effects
- 4 Multilevel Models From a Multiple Group Structural Equation Perspective
- 5 Cross-Domain Analyses of Change Over Time: Combining Growth Modeling and Covariance Structure Analysis
- 6 A Hierarchy of Univariate and Multivariate Structural Times Series Models
- 7 Bootstrapping Techniques in Analysis of Mean and Covariance Structures
- 8 A Limited-Information Estimator for LISREL Models With or Without Heteroscedastic Errors
- 9 Full Information Estimation in the Presence of Incomplete Data
- 10 Inference Problems With Equivalent Models
- 11 An Evaluation of Incremental Fit Indices: A Clarification of Mathematical and Empirical Properties
- Author Index
- Subject Index
- About the Authors
Estilos de citas para Advanced Structural Equation Modeling
APA 6 Citation
[author missing]. (2013). Advanced Structural Equation Modeling (1st ed.). Taylor and Francis. Retrieved from https://www.perlego.com/book/1548091/advanced-structural-equation-modeling-issues-and-techniques-pdf (Original work published 2013)
Chicago Citation
[author missing]. (2013) 2013. Advanced Structural Equation Modeling. 1st ed. Taylor and Francis. https://www.perlego.com/book/1548091/advanced-structural-equation-modeling-issues-and-techniques-pdf.
Harvard Citation
[author missing] (2013) Advanced Structural Equation Modeling. 1st edn. Taylor and Francis. Available at: https://www.perlego.com/book/1548091/advanced-structural-equation-modeling-issues-and-techniques-pdf (Accessed: 14 October 2022).
MLA 7 Citation
[author missing]. Advanced Structural Equation Modeling. 1st ed. Taylor and Francis, 2013. Web. 14 Oct. 2022.