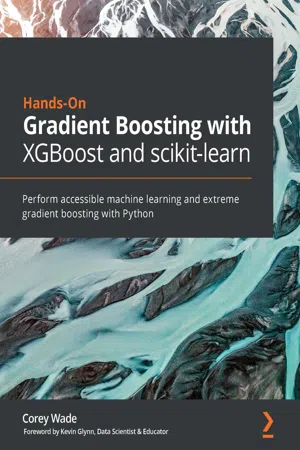
Hands-On Gradient Boosting with XGBoost and scikit-learn
Perform accessible machine learning and extreme gradient boosting with Python
Corey Wade
- 310 páginas
- English
- ePUB (apto para móviles)
- Disponible en iOS y Android
Hands-On Gradient Boosting with XGBoost and scikit-learn
Perform accessible machine learning and extreme gradient boosting with Python
Corey Wade
Información del libro
Get to grips with building robust XGBoost models using Python and scikit-learn for deployment
Key Features
- Get up and running with machine learning and understand how to boost models with XGBoost in no time
- Build real-world machine learning pipelines and fine-tune hyperparameters to achieve optimal results
- Discover tips and tricks and gain innovative insights from XGBoost Kaggle winners
Book Description
XGBoost is an industry-proven, open-source software library that provides a gradient boosting framework for scaling billions of data points quickly and efficiently.
The book introduces machine learning and XGBoost in scikit-learn before building up to the theory behind gradient boosting. You'll cover decision trees and analyze bagging in the machine learning context, learning hyperparameters that extend to XGBoost along the way. You'll build gradient boosting models from scratch and extend gradient boosting to big data while recognizing speed limitations using timers. Details in XGBoost are explored with a focus on speed enhancements and deriving parameters mathematically. With the help of detailed case studies, you'll practice building and fine-tuning XGBoost classifiers and regressors using scikit-learn and the original Python API. You'll leverage XGBoost hyperparameters to improve scores, correct missing values, scale imbalanced datasets, and fine-tune alternative base learners. Finally, you'll apply advanced XGBoost techniques like building non-correlated ensembles, stacking models, and preparing models for industry deployment using sparse matrices, customized transformers, and pipelines.
By the end of the book, you'll be able to build high-performing machine learning models using XGBoost with minimal errors and maximum speed.
What you will learn
- Build gradient boosting models from scratch
- Develop XGBoost regressors and classifiers with accuracy and speed
- Analyze variance and bias in terms of fine-tuning XGBoost hyperparameters
- Automatically correct missing values and scale imbalanced data
- Apply alternative base learners like dart, linear models, and XGBoost random forests
- Customize transformers and pipelines to deploy XGBoost models
- Build non-correlated ensembles and stack XGBoost models to increase accuracy
Who this book is for
This book is for data science professionals and enthusiasts, data analysts, and developers who want to build fast and accurate machine learning models that scale with big data. Proficiency in Python, along with a basic understanding of linear algebra, will help you to get the most out of this book.
Preguntas frecuentes
Información
Section 1: Bagging and Boosting
- Chapter 1, Machine Learning Landscape
- Chapter 2, Decision Trees in Depth
- Chapter 3, Bagging with Random Forests
- Chapter 4, From Gradient Boosting to XGBoost
Chapter 1: Machine Learning Landscape
- Previewing XGBoost
- Wrangling data
- Predicting regression
- Predicting classification
Previewing XGBoost
What is machine learning?
Data wrangling
Índice
- Hands-On Gradient Boosting with XGBoost and scikit-learn
- Why subscribe?
- Preface
- Section 1: Bagging and Boosting
- Chapter 1: Machine Learning Landscape
- Chapter 2: Decision Trees in Depth
- Chapter 3: Bagging with Random Forests
- Chapter 4: From Gradient Boosting to XGBoost
- Section 2: XGBoost
- Chapter 5: XGBoost Unveiled
- Chapter 6: XGBoost Hyperparameters
- Chapter 7: Discovering Exoplanets with XGBoost
- Section 3: Advanced XGBoost
- Chapter 8: XGBoost Alternative Base Learners
- Chapter 9: XGBoost Kaggle Masters
- Chapter 10: XGBoost Model Deployment
- Other Books You May Enjoy