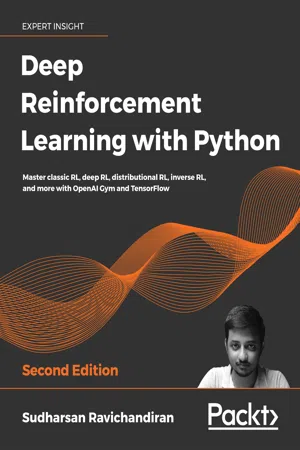
Deep Reinforcement Learning with Python
Master classic RL, deep RL, distributional RL, inverse RL, and more with OpenAI Gym and TensorFlow, 2nd Edition
Sudharsan Ravichandiran
- 760 páginas
- English
- ePUB (apto para móviles)
- Disponible en iOS y Android
Deep Reinforcement Learning with Python
Master classic RL, deep RL, distributional RL, inverse RL, and more with OpenAI Gym and TensorFlow, 2nd Edition
Sudharsan Ravichandiran
Información del libro
An example-rich guide for beginners to start their reinforcement and deep reinforcement learning journey with state-of-the-art distinct algorithms
Key Features
- Covers a vast spectrum of basic-to-advanced RL algorithms with mathematical explanations of each algorithm
- Learn how to implement algorithms with code by following examples with line-by-line explanations
- Explore the latest RL methodologies such as DDPG, PPO, and the use of expert demonstrations
Book Description
With significant enhancements in the quality and quantity of algorithms in recent years, this second edition of Hands-On Reinforcement Learning with Python has been revamped into an example-rich guide to learning state-of-the-art reinforcement learning (RL) and deep RL algorithms with TensorFlow 2 and the OpenAI Gym toolkit.
In addition to exploring RL basics and foundational concepts such as Bellman equation, Markov decision processes, and dynamic programming algorithms, this second edition dives deep into the full spectrum of value-based, policy-based, and actor-critic RL methods. It explores state-of-the-art algorithms such as DQN, TRPO, PPO and ACKTR, DDPG, TD3, and SAC in depth, demystifying the underlying math and demonstrating implementations through simple code examples.
The book has several new chapters dedicated to new RL techniques, including distributional RL, imitation learning, inverse RL, and meta RL. You will learn to leverage stable baselines, an improvement of OpenAI's baseline library, to effortlessly implement popular RL algorithms. The book concludes with an overview of promising approaches such as meta-learning and imagination augmented agents in research.
By the end, you will become skilled in effectively employing RL and deep RL in your real-world projects.
What you will learn
- Understand core RL concepts including the methodologies, math, and code
- Train an agent to solve Blackjack, FrozenLake, and many other problems using OpenAI Gym
- Train an agent to play Ms Pac-Man using a Deep Q Network
- Learn policy-based, value-based, and actor-critic methods
- Master the math behind DDPG, TD3, TRPO, PPO, and many others
- Explore new avenues such as the distributional RL, meta RL, and inverse RL
- Use Stable Baselines to train an agent to walk and play Atari games
Who this book is for
If you're a machine learning developer with little or no experience with neural networks interested in artificial intelligence and want to learn about reinforcement learning from scratch, this book is for you.
Basic familiarity with linear algebra, calculus, and the Python programming language is required. Some experience with TensorFlow would be a plus.
Preguntas frecuentes
Información
Appendix 1 – Reinforcement Learning Algorithms
Reinforcement learning algorithm
- First, the agent interacts with the environment by performing an action.
- The agent performs an action and moves from one state to another.
- Then the agent will receive a reward based on the action it performed.
- Based on the reward, the agent will understand whether the action is good or bad.
- If the action was good, that is, if the agent received a positive reward, then the agent will prefer performing that action, else the agent will try performing other actions that can result in a positive reward. So reinforcement learning is basically a trial-and-error learning process.
Value Iteration
- Compute the optimal value function by taking maximum over the Q function, that is,
- Extract the optimal policy from the computed optimal value function
Policy Iteration
- Initialize a random policy
- Compute the value function using the given policy
- Extract a new policy using the value function obtained from step 2
- If the extracted policy is the same as the policy used in step 2 then stop, else send the extracted new policy to step 2 and repeat steps 2 to 4
First-Visit MC Prediction
- Let total_return(s) be the sum of the return of a state across several episodes and N(s) be the counter, that is, the number of times a state is visited ac...
Índice
- Preface
- Fundamentals of Reinforcement Learning
- A Guide to the Gym Toolkit
- The Bellman Equation and Dynamic Programming
- Monte Carlo Methods
- Understanding Temporal Difference Learning
- Case Study – The MAB Problem
- Deep Learning Foundations
- A Primer on TensorFlow
- Deep Q Network and Its Variants
- Policy Gradient Method
- Actor-Critic Methods – A2C and A3C
- Learning DDPG, TD3, and SAC
- TRPO, PPO, and ACKTR Methods
- Distributional Reinforcement Learning
- Imitation Learning and Inverse RL
- Deep Reinforcement Learning with Stable Baselines
- Reinforcement Learning Frontiers
- Appendix 1 – Reinforcement Learning Algorithms
- Appendix 2 – Assessments
- Other Books You May Enjoy
- Index