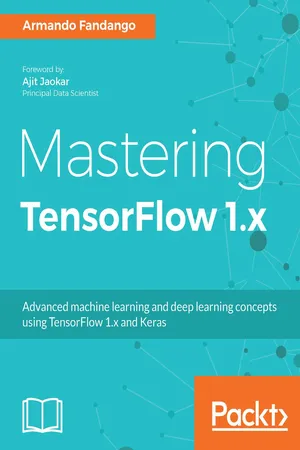
Mastering TensorFlow 1.x
Armando Fandango, Nick McClure
- 474 páginas
- English
- ePUB (apto para móviles)
- Disponible en iOS y Android
Mastering TensorFlow 1.x
Armando Fandango, Nick McClure
Información del libro
Build, scale, and deploy deep neural network models using the star libraries in Python
Key Features
- Delve into advanced machine learning and deep learning use cases using Tensorflow and Keras
- Build, deploy, and scale end-to-end deep neural network models in a production environment
- Learn to deploy TensorFlow on mobile, and distributed TensorFlow on GPU, Clusters, and Kubernetes
Book Description
TensorFlow is the most popular numerical computation library built from the ground up for distributed, cloud, and mobile environments. TensorFlow represents the data as tensors and the computation as graphs.
This book is a comprehensive guide that lets you explore the advanced features of TensorFlow 1.x. Gain insight into TensorFlow Core, Keras, TF Estimators, TFLearn, TF Slim, Pretty Tensor, and Sonnet. Leverage the power of TensorFlow and Keras to build deep learning models, using concepts such as transfer learning, generative adversarial networks, and deep reinforcement learning. Throughout the book, you will obtain hands-on experience with varied datasets, such as MNIST, CIFAR-10, PTB, text8, and COCO-Images.
You will learn the advanced features of TensorFlow1.x, such as distributed TensorFlow with TF Clusters, deploy production models with TensorFlow Serving, and build and deploy TensorFlow models for mobile and embedded devices on Android and iOS platforms. You will see how to call TensorFlow and Keras API within the R statistical software, and learn the required techniques for debugging when the TensorFlow API-based code does not work as expected.
The book helps you obtain in-depth knowledge of TensorFlow, making you the go-to person for solving artificial intelligence problems. By the end of this guide, you will have mastered the offerings of TensorFlow and Keras, and gained the skills you need to build smarter, faster, and efficient machine learning and deep learning systems.
What you will learn
- Master advanced concepts of deep learning such as transfer learning, reinforcement learning, generative models and more, using TensorFlow and Keras
- Perform supervised (classification and regression) and unsupervised (clustering) learning to solve machine learning tasks
- Build end-to-end deep learning (CNN, RNN, and Autoencoders) models with TensorFlow
- Scale and deploy production models with distributed and high-performance computing on GPU and clusters
- Build TensorFlow models to work with multilayer perceptrons using Keras, TFLearn, and R
- Learn the functionalities of smart apps by building and deploying TensorFlow models on iOS and Android devices
- Supercharge TensorFlow with distributed training and deployment on Kubernetes and TensorFlow Clusters
Who this book is for
This book is for data scientists, machine learning engineers, artificial intelligence engineers, and for all TensorFlow users who wish to upgrade their TensorFlow knowledge and work on various machine learning and deep learning problems. If you are looking for an easy-to-follow guide that underlines the intricacies and complex use cases of machine learning, you will find this book extremely useful. Some basic understanding of TensorFlow is required to get the most out of the book.
Preguntas frecuentes
Información
Transfer Learning and Pre-Trained Models
- ImageNet dataset
- Retraining or fine-tuning the models
- COCO animals dataset and preprocessing
- Image classification using pre-trained VGG16 in TensorFlow
- Image preprocessing in TensorFlow for pre-trained VGG16
- Image classification using retrained VGG16 in TensorFlow
- Image classification using pre-trained VGG16 in Keras
- Image classification using retrained VGG16 in Keras
- Image classification using Inception v3 in TensorFlow
- Image classification using retrained Inception v3 in TensorFlow
ImageNet dataset
http://image-net.org/challenges/LSVRC/2017/browse-synsets
http://image-net.org/challenges/LSVRC/2016/browse-synsets
http://image-net.org/challenges/LSVRC/2015/browse-synsets
http://image-net.org/challenges/LSVRC/2014/browse-synsets
http://image-net.org/challenges/LSVRC/2013/browse-synsets
http://image-net.org/challenges/LSVRC/2012/browse-synsets
http://image-net.org/challenges/LSVRC/2011/browse-synsets
http://image-net.org/challenges/LSVRC/2010/browse-synsets.
def build_id2label(self):
base_url = 'https://raw.githubusercontent.com/tensorflow/models/master/research/inception/inception/data/'
synset_url = '{}/imagenet_lsvrc_2015_synsets.txt'.format(base_url)
synset_to_human_url = '{}/imagenet_metadata.txt'.format(base_url)
filename, _ = urllib.request.urlretrieve(synset_url)
synset_list = [s.strip() for s in open(filename).readlines()]
nu...
Índice
- Title Page
- Copyright and Credits
- Packt Upsell
- Foreword
- Contributors
- Preface
- TensorFlow 101
- High-Level Libraries for TensorFlow
- Keras 101
- Classical Machine Learning with TensorFlow
- Neural Networks and MLP with TensorFlow and Keras
- RNN with TensorFlow and Keras
- RNN for Time Series Data with TensorFlow and Keras
- RNN for Text Data with TensorFlow and Keras
- CNN with TensorFlow and Keras
- Autoencoder with TensorFlow and Keras
- TensorFlow Models in Production with TF Serving
- Transfer Learning and Pre-Trained Models
- Deep Reinforcement Learning
- Generative Adversarial Networks
- Distributed Models with TensorFlow Clusters
- TensorFlow Models on Mobile and Embedded Platforms
- TensorFlow and Keras in R
- Debugging TensorFlow Models
- Tensor Processing Units
- Other Books You May Enjoy