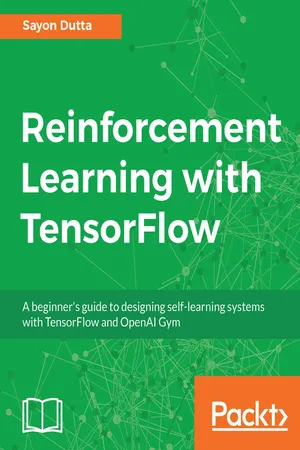
Reinforcement Learning with TensorFlow
Sayon Dutta
- 334 páginas
- English
- ePUB (apto para móviles)
- Disponible en iOS y Android
Reinforcement Learning with TensorFlow
Sayon Dutta
Información del libro
Leverage the power of the Reinforcement Learning techniques to develop self-learning systems using TensorflowAbout This Book• Learn reinforcement learning concepts and their implementation using TensorFlow• Discover different problem-solving methods for Reinforcement Learning• Apply reinforcement learning for autonomous driving cars, robobrokers, and moreWho This Book Is ForIf you want to get started with reinforcement learning using TensorFlow in the most practical way, this book will be a useful resource. The book assumes prior knowledge of machine learning and neural network programming concepts, as well as some understanding of the TensorFlow framework. No previous experience with Reinforcement Learning is required.What You Will Learn• Implement state-of-the-art Reinforcement Learning algorithms from the basics• Discover various techniques of Reinforcement Learning such as MDP, Q Learning and more• Learn the applications of Reinforcement Learning in advertisement, image processing, and NLP• Teach a Reinforcement Learning model to play a game using TensorFlow and the OpenAI gym• Understand how Reinforcement Learning Applications are used in roboticsIn DetailReinforcement Learning (RL), allows you to develop smart, quick and self-learning systems in your business surroundings. It is an effective method to train your learning agents and solve a variety of problems in Artificial Intelligence—from games, self-driving cars and robots to enterprise applications that range from datacenter energy saving (cooling data centers) to smart warehousing solutions.The book covers the major advancements and successes achieved in deep reinforcement learning by synergizing deep neural network architectures with reinforcement learning. The book also introduces readers to the concept of Reinforcement Learning, its advantages and why it's gaining so much popularity. The book also discusses on MDPs, Monte Carlo tree searches, dynamic programming such as policy and value iteration, temporal difference learning such as Q-learning and SARSA. You will use TensorFlow and OpenAI Gym to build simple neural network models that learn from their own actions. You will also see how reinforcement learning algorithms play a role in games, image processing and NLP.By the end of this book, you will have a firm understanding of what reinforcement learning is and how to put your knowledge to practical use by leveraging the power of TensorFlow and OpenAI Gym.Style and approachAn Easy-to-follow, step-by-step guide to help you get to grips with real-world applications of Reinforcement Learning with TensorFlow.
Preguntas frecuentes
Información
Deep Learning – Architectures and Frameworks
- Deep learning
- Reinforcement learning
- Introduction to TensorFlow and OpenAI Gym
- The influential researchers and projects in reinforcement learning
Deep learning
Inputs(x) | Output(y) | Application domain | Suggested neural network approach |
House features | Price of the house | Real estate | Standard neural network with rectified linear unit in the output layer |
Ad and user info Click on ad ? | Yes(1) or No(0) | Online advertising | Standard neural network with binary classification |
Image object | Classifying from 100 different objects, that is (1,2,.....,100) | Photo tagging | Convolutional neural network (since image, that is, spatial data) |
Audio | Text transcript | Speech recognition | Recurrent neural network (since both input-output are sequential data) |
English | Chinese | Machine translation | Recurrent neural network (since the input is a sequential data) |
Image, radar information | Position of other cars | Autonomous driving | Customized hybrid/complex neural network |
Índice
- Title Page
- Copyright and Credits
- Packt Upsell
- Contributors
- Preface
- Deep Learning – Architectures and Frameworks
- Training Reinforcement Learning Agents Using OpenAI Gym
- Markov Decision Process
- Policy Gradients
- Q-Learning and Deep Q-Networks
- Asynchronous Methods
- Robo Everything – Real Strategy Gaming
- AlphaGo – Reinforcement Learning at Its Best
- Reinforcement Learning in Autonomous Driving
- Financial Portfolio Management
- Reinforcement Learning in Robotics
- Deep Reinforcement Learning in Ad Tech
- Reinforcement Learning in Image Processing
- Deep Reinforcement Learning in NLP
- Further topics in Reinforcement Learning
- Other Books You May Enjoy