
Hands-On Generative Adversarial Networks with PyTorch 1.x
Implement next-generation neural networks to build powerful GAN models using Python
John Hany, Greg Walters
- 312 pages
- English
- ePUB (adapté aux mobiles)
- Disponible sur iOS et Android
Hands-On Generative Adversarial Networks with PyTorch 1.x
Implement next-generation neural networks to build powerful GAN models using Python
John Hany, Greg Walters
Ă propos de ce livre
Apply deep learning techniques and neural network methodologies to build, train, and optimize generative network models
Key Features
- Implement GAN architectures to generate images, text, audio, 3D models, and more
- Understand how GANs work and become an active contributor in the open source community
- Learn how to generate photo-realistic images based on text descriptions
Book Description
With continuously evolving research and development, Generative Adversarial Networks (GANs) are the next big thing in the field of deep learning. This book highlights the key improvements in GANs over generative models and guides in making the best out of GANs with the help of hands-on examples.
This book starts by taking you through the core concepts necessary to understand how each component of a GAN model works. You'll build your first GAN model to understand how generator and discriminator networks function. As you advance, you'll delve into a range of examples and datasets to build a variety of GAN networks using PyTorch functionalities and services, and become well-versed with architectures, training strategies, and evaluation methods for image generation, translation, and restoration. You'll even learn how to apply GAN models to solve problems in areas such as computer vision, multimedia, 3D models, and natural language processing (NLP). The book covers how to overcome the challenges faced while building generative models from scratch. Finally, you'll also discover how to train your GAN models to generate adversarial examples to attack other CNN and GAN models.
By the end of this book, you will have learned how to build, train, and optimize next-generation GAN models and use them to solve a variety of real-world problems.
What you will learn
- Implement PyTorch's latest features to ensure efficient model designing
- Get to grips with the working mechanisms of GAN models
- Perform style transfer between unpaired image collections with CycleGAN
- Build and train 3D-GANs to generate a point cloud of 3D objects
- Create a range of GAN models to perform various image synthesis operations
- Use SEGAN to suppress noise and improve the quality of speech audio
Who this book is for
This GAN book is for machine learning practitioners and deep learning researchers looking to get hands-on guidance in implementing GAN models using PyTorch. You'll become familiar with state-of-the-art GAN architectures with the help of real-world examples. Working knowledge of Python programming language is necessary to grasp the concepts covered in this book.
Foire aux questions
Informations
Section 1: Introduction to GANs and PyTorch
- Chapter 1, Generative Adversarial Networks Fundamentals
- Chapter 2, Getting Started with PyTorch 1.3
- Chapter 3, Best Practices in Model Design and Training
Generative Adversarial Networks Fundamentals
- Fundamentals of machine learning
- Generator and discriminator networks
- What GAN we do?
- References and a useful reading list
Fundamentals of machine learning
Machine learning â classification and generation
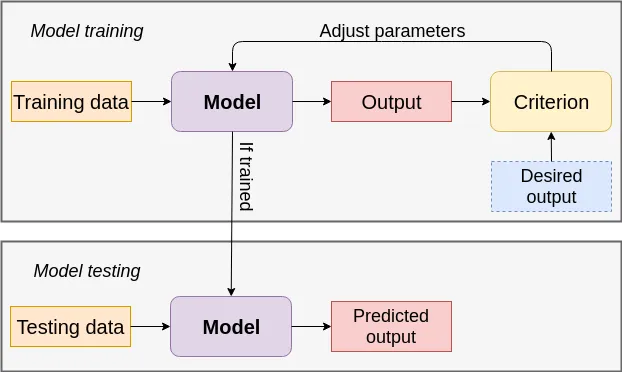