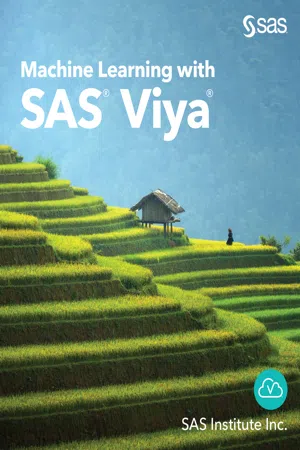
Machine Learning with SAS Viya
- 386 pages
- English
- ePUB (adapté aux mobiles)
- Disponible sur iOS et Android
Machine Learning with SAS Viya
Ă propos de ce livre
Master machine learning with SAS Viya!
Machine learning can feel intimidating for new practitioners. Machine Learning with SAS Viya provides everything you need to know to get started with machine learning in SAS Viya, including decision trees, neural networks, and support vector machines. The analytics life cycle is covered from data preparation and discovery to deployment. Working with open-source code? Machine Learning with SAS Viya has you covered â step-by-step instructions are given on how to use SAS Model Manager tools with open source. SAS Model Studio features are highlighted to show how to carry out machine learning in SAS Viya. Demonstrations, practice tasks, and quizzes are included to help sharpen your skills.
In this book, you will learn about:
- Supervised and unsupervised machine learning
- Data preparation and dealing with missing and unstructured data
- Model building and selection
- Improving and optimizing models
- Model deployment and monitoring performance
Foire aux questions
Informations
Table des matiĂšres
- About This Book
- Acknowledgments
- Preface
- Chapter 1: Introduction to Machine Learning
- Chapter 2: Preparing Your Data: Introduction
- Chapter 3: Preparing Your Data: Missing and Unstructured Data
- Chapter 4: Preparing Your Data: Extract Features
- Chapter 5: Discovery: Selecting an Algorithm
- Chapter 6: Decision Trees: Introduction
- Chapter 7: Decision Trees: Improving the Model
- Chapter 8: Decision Trees: Ensembles and Forests
- Chapter 9: Neural Networks: Introduction and Model Architecture
- Chapter 10: Neural Networks: Optimizing the Model and Learning
- Chapter 11: Support Vector Machines
- Chapter 12: Model Assessment and Deployment
- Chapter 13: Additional Model Manager Tools and Open-Source Code
- Appendix A
- Appendix B: Solutions
- References