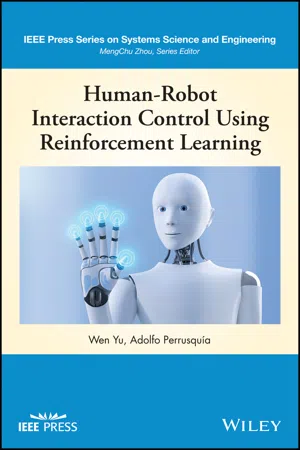
Human-Robot Interaction Control Using Reinforcement Learning
Wen Yu,Adolfo Perrusquia
- English
- ePUB (adapté aux mobiles)
- Disponible sur iOS et Android
Human-Robot Interaction Control Using Reinforcement Learning
Wen Yu,Adolfo Perrusquia
Ă propos de ce livre
A comprehensive exploration of the control schemes of human-robot interactions
In Human-Robot Interaction Control Using Reinforcement Learning, an expert team of authors deliversa concise overview of human-robot interaction control schemes and insightful presentations of novel, model-free and reinforcement learning controllers. The book begins with a brief introduction to state-of-the-art human-robot interaction control and reinforcement learning before moving on to describe the typical environment model. The authors also describe some of the most famous identification techniques for parameter estimation.
Human-Robot Interaction Control Using Reinforcement Learning offers rigorous mathematical treatments and demonstrations that facilitate the understanding of control schemes and algorithms.It also describes stability and convergence analysis of human-robot interaction control and reinforcement learning based control.
The authorsalsodiscussadvanced and cutting-edge topics, like inverse and velocity kinematics solutions, H2 neural control, and likely upcoming developments in the field of robotics.
Readers will also enjoy:
- A thorough introduction to model-based human-robot interaction control
- Comprehensive explorations of model-free human-robot interaction control and human-in-the-loop control using Euler angles
- Practical discussions of reinforcement learning for robot position and force control, as well as continuous time reinforcement learning for robot force control
- In-depth examinations of robot control in worst-case uncertainty using reinforcement learning and the control of redundant robots using multi-agent reinforcement learning
Perfect forsenior undergraduate and graduate students, academic researchers, and industrial practitioners studying and working in the fields of robotics, learning control systems, neural networks, and computational intelligence, Human-Robot Interaction Control Using Reinforcement Learning is also an indispensable resource forstudents and professionals studying reinforcement learning.
Foire aux questions
Informations
Part I
Humanârobot Interaction Control
1
Introduction
1.1 HumanâRobot Interaction Control






Table des matiĂšres
- Cover
- Table of Contents
- Title Page
- Copyright
- Dedication
- Author Biographies
- List of Figures
- List of Tables
- Preface
- Part I: Humanârobot Interaction Control
- Part II: Reinforcement Learning for Robot Interaction Control
- A Robot Kinematics and Dynamics
- B Reinforcement Learning for Control
- Index
- End User License Agreement