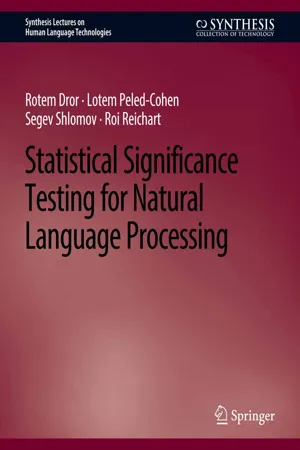
Statistical Significance Testing for Natural Language Processing
Rotem Dror,Lotem Peled-Cohen,Segev Shlomov,Roi Reichart
- English
- PDF
- Disponible sur iOS et Android
Statistical Significance Testing for Natural Language Processing
Rotem Dror,Lotem Peled-Cohen,Segev Shlomov,Roi Reichart
Ă propos de ce livre
Data-driven experimental analysis has become the main evaluation tool of Natural Language Processing (NLP) algorithms. In fact, in the last decade, it has become rare to see an NLP paper, particularly one that proposes a new algorithm, that does not include extensive experimental analysis, and the number of involved tasks, datasets, domains, and languages is constantly growing. This emphasis on empirical results highlights the role of statistical significance testing in NLP research: If we, as a community, rely on empirical evaluation to validate our hypotheses and reveal the correct language processing mechanisms, we better be sure that our results are not coincidental.
The goal of this book is to discuss the main aspects of statistical significance testing in NLP. Our guiding assumption throughout the book is that the basic question NLP researchers and engineers deal with is whether or not one algorithm can be considered better than another one. This question drivesthe field forward as it allows the constant progress of developing better technology for language processing challenges. In practice, researchers and engineers would like to draw the right conclusion from a limited set of experiments, and this conclusion should hold for other experiments with datasets they do not have at their disposal or that they cannot perform due to limited time and resources. The book hence discusses the opportunities and challenges in using statistical significance testing in NLP, from the point of view of experimental comparison between two algorithms. We cover topics such as choosing an appropriate significance test for the major NLP tasks, dealing with the unique aspects of significance testing for non-convex deep neural networks, accounting for a large number of comparisons between two NLP algorithms in a statistically valid manner (multiple hypothesis testing), and, finally, the unique challenges yielded by the nature of the data and practices of the field.
Foire aux questions
Informations
Table des matiĂšres
- Cover
- Copyright
- Title Page
- Content
- Preface
- Acknowledgments
- Introduction
- Statistical Hypothesis Testing
- Statistical Significance Tests
- Statistical Significance in NLP
- Deep Significance
- Replicability Analysis
- Open Questions and Challenges
- Conclusions
- Bibliography
- Authors' Biographies