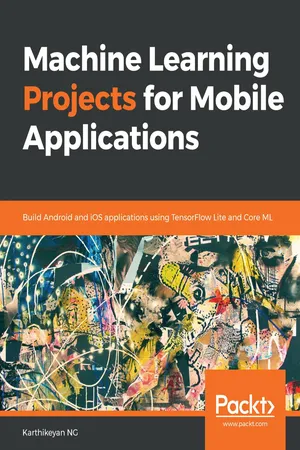
Machine Learning Projects for Mobile Applications
Build Android and iOS applications using TensorFlow Lite and Core ML
Karthikeyan NG
- 246 pages
- English
- ePUB (adapté aux mobiles)
- Disponible sur iOS et Android
Machine Learning Projects for Mobile Applications
Build Android and iOS applications using TensorFlow Lite and Core ML
Karthikeyan NG
Ă propos de ce livre
Bring magic to your mobile apps using TensorFlow Lite and Core ML
Key Features
- Explore machine learning using classification, analytics, and detection tasks.
- Work with image, text and video datasets to delve into real-world tasks
- Build apps for Android and iOS using Caffe, Core ML and Tensorflow Lite
Book Description
Machine learning is a technique that focuses on developing computer programs that can be modified when exposed to new data. We can make use of it for our mobile applications and this book will show you how to do so.
The book starts with the basics of machine learning concepts for mobile applications and how to get well equipped for further tasks. You will start by developing an app to classify age and gender using Core ML and Tensorflow Lite. You will explore neural style transfer and get familiar with how deep CNNs work. We will also take a closer look at Google's ML Kit for the Firebase SDK for mobile applications. You will learn how to detect handwritten text on mobile. You will also learn how to create your own Snapchat filter by making use of facial attributes and OpenCV. You will learn how to train your own food classification model on your mobile; all of this will be done with the help of deep learning techniques. Lastly, you will build an image classifier on your mobile, compare its performance, and analyze the results on both mobile and cloud using TensorFlow Lite with an RCNN.
By the end of this book, you will not only have mastered the concepts of machine learning but also learned how to resolve problems faced while building powerful apps on mobiles using TensorFlow Lite, Caffe2, and Core ML.
What you will learn
- Demystify the machine learning landscape on mobile
- Age and gender detection using TensorFlow Lite and Core ML
- Use ML Kit for Firebase for in-text detection, face detection, and barcode scanning
- Create a digit classifier using adversarial learning
- Build a cross-platform application with face filters using OpenCV
- Classify food using deep CNNs and TensorFlow Lite on iOS
Who this book is for
Machine Learning Projects for Mobile Applications is for you if you are a data scientist, machine learning expert, deep learning, or AI enthusiast who fancies mastering machine learning and deep learning implementation with practical examples using TensorFlow Lite and CoreML. Basic knowledge of Python programming language would be an added advantage.
Foire aux questions
Informations
Deep Diving into the ML Kit with Firebase
- Understanding the basics of the ML Kit
- Learning to add Firebase to our applications
- Creating multiple applications that can be used for face detection, barcode scanning, and on-device text recognition using Firebase
ML Kit basics
- A very good mobile application developer may not be good at building an ML model. Building an ML model definitely takes time. This may vary on a case-by-case basis.
- Finding the right set of data models that will solve your use case will be a very difficult problem. Let's say you want to detect age and gender classification on an Asian person's face. In this case, the existing models that are available may not be accurate enough for your use case.
- Hosting your own model will be costlier and will require extra care on the server side of the application.

Basic feature set
- Text recognition
- Face detection
- Barcode scanning
- Image labeling
- Landmark detection
Table des matiĂšres
- Title Page
- Copyright and Credits
- Dedication
- Packt Upsell
- Contributors
- Preface
- Mobile Landscapes in Machine Learning
- CNN Based Age and Gender Identification Using Core ML
- Applying Neural Style Transfer on Photos
- Deep Diving into the ML Kit with Firebase
- A Snapchat-Like AR Filter on Android
- Handwritten Digit Classifier Using Adversarial Learning
- Face-Swapping with Your Friends Using OpenCV
- Classifying Food Using Transfer Learning
- What's Next?
- Other Books You May Enjoy