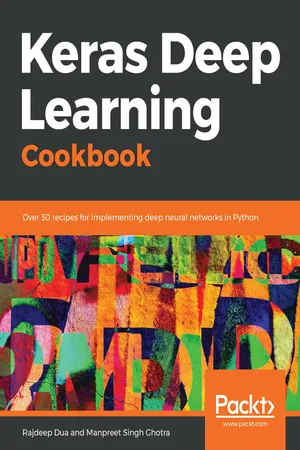
Keras Deep Learning Cookbook
Over 30 recipes for implementing deep neural networks in Python
Rajdeep Dua, Manpreet Singh Ghotra
- 252 pages
- English
- ePUB (adapté aux mobiles)
- Disponible sur iOS et Android
Keras Deep Learning Cookbook
Over 30 recipes for implementing deep neural networks in Python
Rajdeep Dua, Manpreet Singh Ghotra
Ă propos de ce livre
Leverage the power of deep learning and Keras to develop smarter and more efficient data models
Key Features
- Understand different neural networks and their implementation using Keras
- Explore recipes for training and fine-tuning your neural network models
- Put your deep learning knowledge to practice with real-world use-cases, tips, and tricks
Book Description
Keras has quickly emerged as a popular deep learning library. Written in Python, it allows you to train convolutional as well as recurrent neural networks with speed and accuracy.
The Keras Deep Learning Cookbook shows you how to tackle different problems encountered while training efficient deep learning models, with the help of the popular Keras library. Starting with installing and setting up Keras, the book demonstrates how you can perform deep learning with Keras in the TensorFlow. From loading data to fitting and evaluating your model for optimal performance, you will work through a step-by-step process to tackle every possible problem faced while training deep models. You will implement convolutional and recurrent neural networks, adversarial networks, and more with the help of this handy guide. In addition to this, you will learn how to train these models for real-world image and language processing tasks.
By the end of this book, you will have a practical, hands-on understanding of how you can leverage the power of Python and Keras to perform effective deep learning
What you will learn
- Install and configure Keras in TensorFlow
- Master neural network programming using the Keras library
- Understand the different Keras layers
- Use Keras to implement simple feed-forward neural networks, CNNs and RNNs
- Work with various datasets and models used for image and text classification
- Develop text summarization and reinforcement learning models using Keras
Who this book is for
Keras Deep Learning Cookbook is for you if you are a data scientist or machine learning expert who wants to find practical solutions to common problems encountered while training deep learning models. A basic understanding of Python and some experience in machine learning and neural networks is required for this book.
Foire aux questions
Informations
Recurrent Neural Networks
- Simple RNNs for time series data
- LSTM networks for time series data
- LSTM memory example time series forecasting with LSTM
- Sequence to sequence learning for the same length output with LSTM
Introduction
The need for RNNs

Simple RNNs for time series data
keras.layers.SimpleRNN(units, activation='tanh',
use_bias=True,
kernel_initializer='glorot_uniform',
recurrent_initializer='orthogonal',
bias_initializer='zeros',
kernel_regularizer=None,
recurrent_regularizer=None,
bias_regularizer=None,
activity_regularizer=None,
kernel_constraint=None,
recurrent_constraint=None,
bias_constraint=None,
dropout=0.0,
recurrent_dropout=0.0,
return_sequences=False,
return_state=False,
go_backwards=False,
stateful=False,
unroll=False)
Getting ready
"Month","Sales of shampoo over a three year period"
"1-01",266.0
"1-02",145.9
"1-03",183.1
"1-04",119.3
"1-05",180.3
"1-06",168.5
"1-07",231.8
from pandas import read_csv
from matplotlib import pyplot
from pandas import datetime
Loading the dataset
- We define a parser to convert YY to YYYY, shown as follow:
def parser(x):
return datetime.strptime('200' + x, '%Y-%m')
- Next, call the read_csv function of pandas to load a .csv file into a pandas DataFrame.
- The next read_csv function is called in the next code:
series = read_csv('sales-of-shampoo-over-a-three-ye.csv', header=0, parse_dates=[0], index_col=0,
squeeze=True, date_parser=parser)
- Once the series is loaded, let's summarize the first few rows:
print(series.head())
Month
2001-01-01 266.0
2001-02-01 145.9
2001-03-01 183.1
2001-04-01 119.3
2001-05-01 180.3
- Next, let's print the line plot using the pyplot library:
series.plot()
pyplot.show()
Table des matiĂšres
- Title Page
- Copyright and Credits
- Packt Upsell
- Contributors
- Preface
- Keras Installation
- Working with Keras Datasets and Models
- Data Preprocessing, Optimization, and Visualization
- Classification Using Different Keras Layers
- Implementing Convolutional Neural Networks
- Generative Adversarial Networks
- Recurrent Neural Networks
- Natural Language Processing Using Keras Models
- Text Summarization Using Keras Models
- Reinforcement Learning
- Other Books You May Enjoy