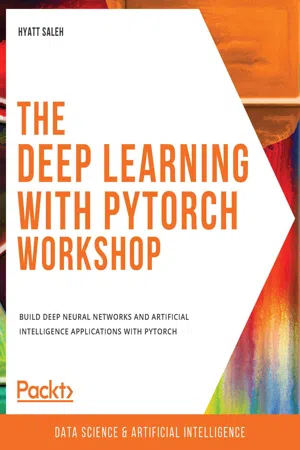
The Deep Learning with PyTorch Workshop
Build deep neural networks and artificial intelligence applications with PyTorch
Hyatt Saleh
- 330 pagine
- English
- ePUB (disponibile sull'app)
- Disponibile su iOS e Android
The Deep Learning with PyTorch Workshop
Build deep neural networks and artificial intelligence applications with PyTorch
Hyatt Saleh
Informazioni sul libro
Get a head start in the world of AI and deep learning by developing your skills with PyTorch
Key Features
- Learn how to define your own network architecture in deep learning
- Implement helpful methods to create and train a model using PyTorch syntax
- Discover how intelligent applications using features like image recognition and speech recognition really process your data
Book Description
Want to get to grips with one of the most popular machine learning libraries for deep learning? The Deep Learning with PyTorch Workshop will help you do just that, jumpstarting your knowledge of using PyTorch for deep learning even if you're starting from scratch.
It's no surprise that deep learning's popularity has risen steeply in the past few years, thanks to intelligent applications such as self-driving vehicles, chatbots, and voice-activated assistants that are making our lives easier. This book will take you inside the world of deep learning, where you'll use PyTorch to understand the complexity of neural network architectures.
The Deep Learning with PyTorch Workshop starts with an introduction to deep learning and its applications. You'll explore the syntax of PyTorch and learn how to define a network architecture and train a model. Next, you'll learn about three main neural network architectures - convolutional, artificial, and recurrent - and even solve real-world data problems using these networks. Later chapters will show you how to create a style transfer model to develop a new image from two images, before finally taking you through how RNNs store memory to solve key data issues.
By the end of this book, you'll have mastered the essential concepts, tools, and libraries of PyTorch to develop your own deep neural networks and intelligent apps.
What you will learn
- Explore the different applications of deep learning
- Understand the PyTorch approach to building neural networks
- Create and train your very own perceptron using PyTorch
- Solve regression problems using artificial neural networks (ANNs)
- Handle computer vision problems with convolutional neural networks (CNNs)
- Perform language translation tasks using recurrent neural networks (RNNs)
Who this book is for
This deep learning book is ideal for anyone who wants to create and train deep learning models using PyTorch. A solid understanding of the Python programming language and its packages will help you grasp the topics covered in the book more quickly.
Domande frequenti
Informazioni
1. Introduction to Deep Learning and PyTorch
Introduction
Why Deep Learning?
- Neural networks require, and actually capitalize on, vast amounts of labeled data to achieve an optimal solution. This means that for the algorithm to create an outstanding model, it requires hundreds of thousands or even millions of entries, containing both the features and the target values. For instance, as regards the image recognition of cats, the more images you have, the more features the model is able to detect, which makes it better.NoteLabeled data refers to data that contains a set of features (characteristics that describe an instance) and a target value (the value to be achieved); for example, a dataset containing demographical and financial information, with a target feature that determines the wage of a person.The following plot shows the performance of deep learning against other algorithms in terms of the quantity of data:

- Neural networks require considerable computing power to be able to process such large amounts of data without taking weeks (or even longer) to be trained. Because the process of achieving the best possible model is based on trial and error, it is necessary to be able to run the training process as efficiently as possible.This can be achieved today through the use of GPUs, which can cut down the training time of a neural network from weeks to hours.NoteWith the objective of accelerating deep learning in order to be able to make use of large amounts of training data and construct state-of-the-art models, field-programmable gate arrays (FPGAs) and tensor processing units (TPUs) are being developed by major cloud computing providers, such as AWS, Microsoft Azure, and Google.
Applications of Deep Learning
- Self-driving vehicles: Several companies, such as Google, have been working on the development of partially or totally self-driving vehicles that learn to drive by using digital sensors to identify the objects around them.
- Medical diagnosis: Deep learning is impacting this industry by improving the diagnosis accuracy of terminal diseases such as brain and breast cancer. This is done by classifying X-rays (or any other diagnostic imagery mechanisms) of new patients, based on labeled X-rays from previous patients that did or did not have cancer.
- Voice assistants: This may be one of the most popular applications nowadays, due to the proliferation of different voice-activated intelligent assistants, such as Apple's Siri, Google Home, and Amazon's Alexa.
- Automatic text generation: This means generating new text based on an input sentence. This is popularly used in email writing, where the email provider suggests the next couple of words to the user, based on the text that's already been written.
- Advertising: In the commercial world, deep learning is helping to increase the return on investment of advertising campaigns by targeting the right audiences and by creating more effective ads. One example of this is the generation of content in order to produce up-to-date and informative blogs that help to engage current customers and attract new ones.
- Price forecasting: For beginners, this is a typical example of what can be achieved through the use of machine learning algorithms. Price forecasting consists of training a model based on real data. For instance, in the field of real estate, this would consist of feeding a model with property characteristics and their final price in order to be able to predict the prices of future entries based solely on property characteristics.
Introduction to PyTorch
GPUs in PyTorch
Indice dei contenuti
- The Deep Learning with PyTorch Workshop
- Preface
- 1. Introduction to Deep Learning and PyTorch
- 2. Building Blocks of Neural Networks
- 3. A Classification Problem Using DNN
- 4. Convolutional Neural Networks
- 5. Style Transfer
- 6. Analyzing the Sequence of Data with RNNs
- Appendix