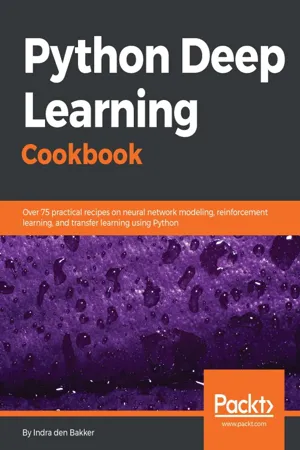
Python Deep Learning Cookbook
- English
- ePUB (disponibile sull'app)
- Disponibile solo in versione web
Python Deep Learning Cookbook
Informazioni sul libro
Solve different problems in modelling deep neural networks using Python, Tensorflow, and Keras with this practical guideAbout This Book• Practical recipes on training different neural network models and tuning them for optimal performance• Use Python frameworks like TensorFlow, Caffe, Keras, Theano for Natural Language Processing, Computer Vision, and more• A hands-on guide covering the common as well as the not so common problems in deep learning using PythonWho This Book Is ForThis book is intended for machine learning professionals who are looking to use deep learning algorithms to create real-world applications using Python. Thorough understanding of the machine learning concepts and Python libraries such as NumPy, SciPy and scikit-learn is expected. Additionally, basic knowledge in linear algebra and calculus is desired.What You Will Learn• Implement different neural network models in Python• Select the best Python framework for deep learning such as PyTorch, Tensorflow, MXNet and Keras• Apply tips and tricks related to neural networks internals, to boost learning performances• Consolidate machine learning principles and apply them in the deep learning field• Reuse and adapt Python code snippets to everyday problems• Evaluate the cost/benefits and performance implication of each discussed solutionIn DetailDeep Learning is revolutionizing a wide range of industries. For many applications, deep learning has proven to outperform humans by making faster and more accurate predictions. This book provides a top-down and bottom-up approach to demonstrate deep learning solutions to real-world problems in different areas. These applications include Computer Vision, Natural Language Processing, Time Series, and Robotics.The Python Deep Learning Cookbook presents technical solutions to the issues presented, along with a detailed explanation of the solutions. Furthermore, a discussion on corresponding pros and cons of implementing the proposed solution using one of the popular frameworks like TensorFlow, PyTorch, Keras and CNTK is provided. The book includes recipes that are related to the basic concepts of neural networks. All techniques s, as well as classical networks topologies. The main purpose of this book is to provide Python programmers a detailed list of recipes to apply deep learning to common and not-so-common scenarios.Style and approachUnique blend of independent recipes arranged in the most logical manner
Domande frequenti
Informazioni
Indice dei contenuti
- Title Page
- Copyright
- Credits
- About the Author
- About the Reviewer
- www.PacktPub.com
- Customer Feedback
- Preface
- Programming Environments, GPU Computing, Cloud Solutions, and Deep Learning Frameworks
- Feed-Forward Neural Networks
- Convolutional Neural Networks
- Recurrent Neural Networks
- Reinforcement Learning
- Generative Adversarial Networks
- Computer Vision
- Natural Language Processing
- Speech Recognition and Video Analysis
- Time Series and Structured Data
- Game Playing Agents and Robotics
- Hyperparameter Selection, Tuning, and Neural Network Learning
- Network Internals
- Pretrained Models