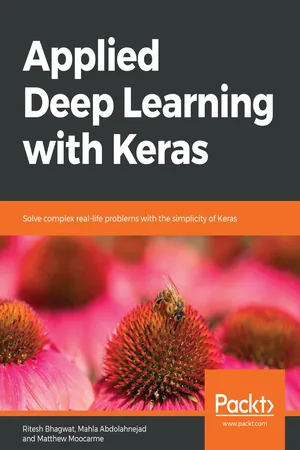
Applied Deep Learning with Keras
Solve complex real-life problems with the simplicity of Keras
Ritesh Bhagwat, Mahla Abdolahnejad, Matthew Moocarme
- 412 pagine
- English
- ePUB (disponibile sull'app)
- Disponibile su iOS e Android
Applied Deep Learning with Keras
Solve complex real-life problems with the simplicity of Keras
Ritesh Bhagwat, Mahla Abdolahnejad, Matthew Moocarme
Informazioni sul libro
Take your neural networks to a whole new level with the simplicity and modularity of Keras, the most commonly used high-level neural networks API.
Key Features
- Solve complex machine learning problems with precision
- Evaluate, tweak, and improve your deep learning models and solutions
- Use different types of neural networks to solve real-world problems
Book Description
Though designing neural networks is a sought-after skill, it is not easy to master. With Keras, you can apply complex machine learning algorithms with minimum code.
Applied Deep Learning with Keras starts by taking you through the basics of machine learning and Python all the way to gaining an in-depth understanding of applying Keras to develop efficient deep learning solutions. To help you grasp the difference between machine and deep learning, the book guides you on how to build a logistic regression model, first with scikit-learn and then with Keras. You will delve into Keras and its many models by creating prediction models for various real-world scenarios, such as disease prediction and customer churning. You'll gain knowledge on how to evaluate, optimize, and improve your models to achieve maximum information. Next, you'll learn to evaluate your model by cross-validating it using Keras Wrapper and scikit-learn. Following this, you'll proceed to understand how to apply L1, L2, and dropout regularization techniques to improve the accuracy of your model. To help maintain accuracy, you'll get to grips with applying techniques including null accuracy, precision, and AUC-ROC score techniques for fine tuning your model.
By the end of this book, you will have the skills you need to use Keras when building high-level deep neural networks.
What you will learn
- Understand the difference between single-layer and multi-layer neural network models
- Use Keras to build simple logistic regression models, deep neural networks, recurrent neural networks, and convolutional neural networks
- Apply L1, L2, and dropout regularization to improve the accuracy of your model
- Implement cross-validate using Keras wrappers with scikit-learn
- Understand the limitations of model accuracy
Who this book is for
If you have basic knowledge of data science and machine learning and want to develop your skills and learn about artificial neural networks and deep learning, you will find this book useful. Prior experience of Python programming and experience with statistics and logistic regression will help you get the most out of this book. Although not necessary, some familiarity with the scikit-learn library will be an added bonus.
Domande frequenti
Informazioni
Chapter 1
Introduction to Machine Learning with Keras
Learning Objectives
- Present data for use in machine learning models
- Explain how to preprocess data for a machine learning model
- Build a logistic regression model with scikit-learn
- Use regularization in machine learning models
- Evaluate model performance with model evaluation metrics
Introduction

Figure 1.1: A trivial classification task for humans, but quite difficult for machines

Figure 1.2: A non-trivial classification task for humans. Are you able to spot the tumors?
Data Representation
Tables of Data

Figure 1.3: A color image and its representation as red, green, and blue images
Note

Figure 1.4: An image showing the first 20 instances of the marketing dataset
Loading Data
Note
Indice dei contenuti
- Preface
- Chapter 1
- Introduction to Machine Learning with Keras
- Chapter 2
- Machine Learning versus Deep Learning
- Chapter 3
- Deep Learning with Keras
- Chapter 4
- Evaluate Your Model with Cross-Validation using Keras Wrappers
- Chapter 5
- Improving Model Accuracy
- Chapter 6
- Model Evaluation
- Chapter 7
- Computer Vision with Convolutional Neural Networks
- Chapter 8
- Transfer Learning and Pre-Trained Models
- Chapter 9
- Sequential Modeling with Recurrent Neural Networks
- Appendix