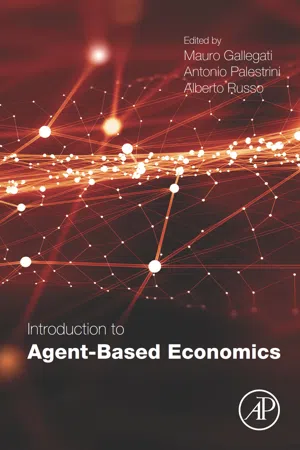
eBook - ePub
Introduction to Agent-Based Economics
Mauro Gallegati,Antonio Palestrini,Alberto Russo
This is a test
Share book
- 274 pages
- English
- ePUB (mobile friendly)
- Available on iOS & Android
eBook - ePub
Introduction to Agent-Based Economics
Mauro Gallegati,Antonio Palestrini,Alberto Russo
Book details
Book preview
Table of contents
Citations
About This Book
Introduction to Agent-Based Economics describes the principal elements of agent-based computational economics (ACE). It illustrates ACE's theoretical foundations, which are rooted in the application of the concept of complexity to the social sciences, and it depicts its growth and development from a non-linear out-of-equilibrium approach to a state-of-the-art agent-based macroeconomics. The book helps readers gain a better understanding of the limits and perspectives of the ACE models and their capacity to reproduce economic phenomena and empirical patterns.
- Reviews the literature of agent-based computational economics
- Analyzes approaches to agents' expectations
- Covers one of the few large macroeconomic agent-based models, the Modellaccio
- Illustrates both analytical and computational methodologies for producing tractable solutions of macro ACE models
- Describes diffusion and amplification mechanisms
- Depicts macroeconomic experiments related to ACE implementations
Frequently asked questions
How do I cancel my subscription?
Can/how do I download books?
At the moment all of our mobile-responsive ePub books are available to download via the app. Most of our PDFs are also available to download and we're working on making the final remaining ones downloadable now. Learn more here.
What is the difference between the pricing plans?
Both plans give you full access to the library and all of Perlegoâs features. The only differences are the price and subscription period: With the annual plan youâll save around 30% compared to 12 months on the monthly plan.
What is Perlego?
We are an online textbook subscription service, where you can get access to an entire online library for less than the price of a single book per month. With over 1 million books across 1000+ topics, weâve got you covered! Learn more here.
Do you support text-to-speech?
Look out for the read-aloud symbol on your next book to see if you can listen to it. The read-aloud tool reads text aloud for you, highlighting the text as it is being read. You can pause it, speed it up and slow it down. Learn more here.
Is Introduction to Agent-Based Economics an online PDF/ePUB?
Yes, you can access Introduction to Agent-Based Economics by Mauro Gallegati,Antonio Palestrini,Alberto Russo in PDF and/or ePUB format, as well as other popular books in Economics & Econometrics. We have over one million books available in our catalogue for you to explore.
Information
Part I
Introduction
Chapter 1
An Introduction to Agent-Based Computational Macroeconomics
Mauro Gallegati; Antonio Palestrini; Alberto Russo Marche Polytechnic University, Ancona, Italy
Economic research frontier of the XXI century has entered the post-neoclassical era. Although most of the textbooks still refers to the orthodox neoclassical theory, this does not reflect the way of thinking of those economists who work at the frontier of the discipline, including those who consider themselves mainstream. The domain of the neoclassical orthodoxy is over, and the discipline to its more advanced levels is looking for an alternative.
David Colander
The recent global crisis has challenged the mainstream approach. The economic crisis has indeed produced a crisis in macroeconomics. Not just because it was not able to predict the arrival of the crisis itself (a very doubtful possibility in social and complex disciplines, but that for Friedman [12] is at the root of the âas ifâ methodology), but rather because such a massive decrease of output cannot even be imagined by the dominant economic theory, obsessed as it is by the straitjacket of equilibrium. Like a physician âspecialized in healthy patients,â mainstream economics seems to work only when things are going well.
Blanchard [3] published a paper with an at least improvident title âThe state of macroeconomics is good,â though he provided a correction a few years after maintaining that until the 2008 global financial crisis, mainstream U.S. macroeconomics had taken an increasingly benign view of economic fluctuations in output and employment; the crisis has made it clear that this view was wrong and that there is a need for a deep reassessment [4]. Blanchard asked whether these models should also be able to describe how the economy behaves in crisis, and the answer he gave was both disarming and ingenious: when things go well, we can still use the DSGE; but another class of economic models, designed to measure systemic risk, can be used to give warning signals when we are getting too close to a crisis and evaluate policies to reduce risk. Trying to create a model that integrates both normal and crisis times (and thus suitable for all seasons) is beyond the conceptual and technical capacity of the profession, at least at this stage. As economists, we can only aspire to a model that explains how the sun rises and another that explains how the sun sets. Science is different: Earthquakes are always attributable to the movement of tectonic plates, regardless of whether they have a magnitude of 0 on the Richter scale (eight thousand a day) or a magnitude of 9 (one every twenty years).
The crisis of mainstream economics is well documented by academic works (see, for instance, [16], [17] and [18], [21]) and central bankers' contributions ([23], [24]). In our opinion, a fundamental feature of macroeconomic modeling resides in the ability to analyze evolutionary complex systems like the economic one. What characterizes a complex system is the notion of emergence, that is, the spontaneous formation of self-organized structures at different layers of a hierarchical system configuration. Agent-Based Modeling (ABM) is a methodological instrumentâthat can be usefully employed by both neoclassical or Keynesian economists, or whatever theoretical approachâwhich is appropriate to study complex dynamics as the result of the interaction of heterogeneous agents (where a degenerate case would be a ârepresentative agentâ model in which the degree of both heterogeneity and interaction is set to zero, which is a situation that reduces holism to reductionism in a hypothetical world without networks and coordination problems).
For the past couple of decades, ABM have seriously taken to heart the concept of economy as an evolving complex system [2,5,22]. Two keywords characterize this approach:
1. Evolving, which means the system is adaptive through learning. Agents' behavioral rules are not fixed (this does not mean that it is not legitimate to build ABMs with fixed rules, for example, to understand what the dynamics of an economic system would be if agents behaved in a certain way), but change adapting to variations of the economic environment in which they interact. As explained in Chapter 6, the traditional approach, which assumes optimizing agents with rational expectations, has been and is a powerful tool for deriving optimal behavioral rules that are valid when economic agents have perfect knowledge of their objective function, and it is common knowledge that all agents optimize an objective function, which is perfectly known unless there are exogenous stochastic disturbances. If agents are not able to optimize, or the common knowledge property is not satisfied, then the rules derived with the traditional approach lose their optimality and become simple rules. Moreover, they are fixed, that is, nonadaptive. In an ABM individual adaptive behavioral rules evolve according to their past performance: this provides a mechanism for an endogenous change of the environment. As a consequence, the ârational expectation hypothesisâ loses significance. However, agents are still rational in the sense that they do what they can in order not to commit systematic errors. In this setting, there is still room for policy intervention outside the mainstream myth of optimal policies. Because emergent facts are transient phenomena, policy recommendations are less certain, and they should be institution and historically oriented.
2. The expression complex system is just as important. It implies that the economic systems have a high level of heterogeneity, indirect and above all direct interactions that can generate emergent properties not inferred from the simple analysis of microeconomic relations [14]. This is the key point of the aggregation problem: starting from the microequations describing the (optimal) choices of the economic units, what can we say about the macroequations? Do they have the same functional form of the microequations? If not, what is the macrotheory derived from? What characterizes a complex system is exactly this notion of emergence, that is, the spontaneous formation of self-organized structures at different layers of a hierarchical system configuration. Mainstream economics conceptualizes the economic system as consisting of several identical and isolated components. If we make N copies of the optimizing agent, then we obtain (under the specific conditions of perfect aggregation) aggregate dynamics. This implies that there are no emergent properties, apart from the ones encapsulated in the microrelations. In economics we know that there are emergent properties not evident from the microlevel. Famous old examples are (i) [19] segregation problem, in which a small preference for same-colored neighbors may produce complete segregation and not a small segregation as one may think from microanalysis, and (ii) the [6] game of life, in which very simple local rules of movement in a two-dimensional grid of square cells produce very complex emergent dynamics.
From this point of view, ABM models take the microfounded approach seriously because they do not assume that microeconomic dynamics always have the same properties of aggregate dynamics [11]. The dynamics of the âevolving agentsâ are aggregated without requiring special conditions for perfect aggregation and a dynamic always in equilibrium, which in traditional analysis lead to mistakes, perhaps negligible in normal economic situations, but to badly wrong analyses in situations of crisis like the present one.
As mentioned before, another point that differentiates the ABM approach used today is the possibility of considering in the former approach also nonequilibrium dynamics. The equilibrium of a system in an ABM no longer requires that every single element be in equilibrium by itself, but rather that the statistical distributions describing aggregate phenomena be stable, that is, in â[...] a state of macroscopic equilibrium maintained by a large number of transitions in opposite directionsâ [10]. In other words, one of the objectives of an ABM simulation (but not the only one) is to make the joint distributions of economic agents converge in a suitable space of distributions. Even when fluctuations of agents occur around equilibrium, which we could calculate using the standard approach, the ABM analyses would not necessarily lead to the same conclusions. This is because the characteristics of the fluctuations would depend on higher moments of the joint distribution and often on the properties of the tails, or three kurtosis of the distribution [13].
ABM is a methodology that allows us to construct, based on simple (evolving) rules of behavior and interaction, models with heterogeneous interacting agents, where the resulting aggregate dynamics and empirical regularities, not known a priori and not deducible from individual behavior, are characterized by three main tenets:
⢠there is a multitude of objects that interact with each other and with the environment;
⢠the objects are autonomous, that is, there is no central or âtop downâ control over their behavior; and
⢠the outcome of their interaction is numerically computed.
We can further characterize the methodology by enumerating a number of features that, although not necessary to define an agent-based model, are often present. These are: Heterogeneity, explicit description of the space of rules, local interaction, bounded rationality, nonequilibrium dynamics, micro-meso-macro empirics. The meso analysis means that you do not have only the micro and the aggregate level, but you can also investigate different levels of aggregation. Differently from Keynesian economic policy, which theorizes aggregate economic policy tools, and mainstream neoclassical economics, which prescribes individual incentives based on [15] but ignores interaction, which is a major but still neglected part of that critique, the ABM approach proposes a bottom-up analysis. Generally what comes out is not a âone-size-fits-allâ policy since its effectiveness depends on the general as well as the idiosyncratic economic conditions; moreover, it generally has to be conducted at different levels (from micro to meso to macro). Furthermore, nonlinear dynamics far from the equilibrium is characterized by an evolutionary process of differentiation, selection, and amplification, which provides the system with novelty and is responsible for its growth in order and complexity, whereas the mainstream approach has no such a mechanism to endogenously creating novelty or generating growth in order and complexity.
This micro-meso-macro possibility does not complete the novelty introduced by the ABM models. As stated before, the moments higher than the first and the tails of joint distribution are often decisive in explaining the aggregate dynamics. Agent-based models have shown a high ability to explain statistical properties of empirical distributions and of stylized facts of the economic and financial cycles [8]: from debt/asset evolution to bankruptcies; from the size and variances of business cycles to their comovements; from industrial dynamics properties, such as growth rate and firm size distribution, to income distribution [9], the probability of exit, and still others.
As mentioned before, results of the ABM model are new because they take into consideration a very important element of economic systems: the networks of direct and indirect interactions, which are often extremely complex and not approximated by simple graphs such as random graphs. Real economies are composed by millions of interacting agents, whose distribution is far from being a simple transformation of the ânormalâ one. As an example, consider the distribution of the trade-credit relations among firms in the electronic-equipment sector in Japan in 2003 [7]. It is quite evident that there exist several hubs, that is, firms with many connections: the distribution of the degree of connectivity (the links) is scale free (power la...