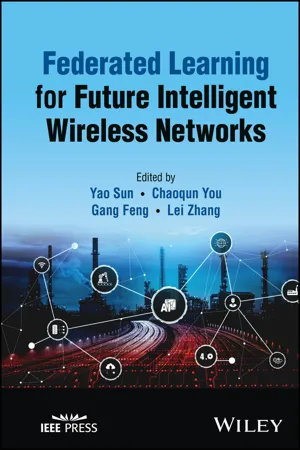
Federated Learning for Future Intelligent Wireless Networks
- English
- PDF
- Available on iOS & Android
Federated Learning for Future Intelligent Wireless Networks
About This Book
Federated Learning for Future Intelligent Wireless Networks
Explore the concepts, algorithms, and applications underlying federated learning
In Federated Learning for Future Intelligent Wireless Networks, a team of distinguished researchers deliver a robust and insightful collection of resources covering the foundational concepts and algorithms powering federated learning, as well as explanations of how they can be used in wireless communication systems. The editors have included works that examine how communication resource provision affects federated learning performance, accuracy, convergence, scalability, and security and privacy.
Readers will explore a wide range of topics that show how federated learning algorithms, concepts, and design and optimization issues apply to wireless communications. Readers will also find:
- A thorough introduction to the fundamental concepts and algorithms of federated learning, including horizontal, vertical, and hybrid FL
- Comprehensive explorations of wireless communication network design and optimization for federated learning
- Practical discussions of novel federated learning algorithms and frameworks for future wireless networks
- Expansive case studies in edge intelligence, autonomous driving, IoT, MEC, blockchain, and content caching and distribution
Perfect for electrical and computer science engineers, researchers, professors, and postgraduate students with an interest in machine learning, Federated Learning for Future Intelligent Wireless Networks will also benefit regulators and institutional actors responsible for overseeing and making policy in the area of artificial intelligence.
Frequently asked questions
Information
Table of contents
- Cover
- Title Page
- Copyright
- Contents
- About the Editors
- Preface
- Chapter 1 Federated Learning with Unreliable Transmission in Mobile Edge Computing Systems
- Chapter 2 Federated Learning with non‐IID data in Mobile Edge Computing Systems
- Chapter 3 How Many Resources Are Needed to Support Wireless Edge Networks
- Chapter 4 Device Association Based on Federated Deep Reinforcement Learning for Radio Access Network Slicing
- Chapter 5 Deep Federated Learning Based on Knowledge Distillation and Differential Privacy
- Chapter 6 Federated Learning‐Based Beam Management in Dense Millimeter Wave Communication Systems
- Chapter 7 Blockchain‐Empowered Federated Learning Approach for An Intelligent and Reliable D2D Caching Scheme
- Chapter 8 Heterogeneity‐Aware Dynamic Scheduling for Federated Edge Learning
- Chapter 9 Robust Federated Learning with Real‐World Noisy Data
- Chapter 10 Analog Over‐the‐Air Federated Learning: Design and Analysis
- Chapter 11 Federated Edge Learning for Massive MIMO CSI Feedback
- Chapter 12 User‐Centric Decentralized Federated Learning for Autoencoder‐Based CSI Feedback
- Index
- EULA