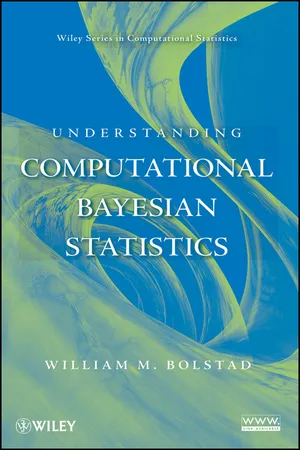
- English
- ePUB (mobile friendly)
- Available on iOS & Android
Understanding Computational Bayesian Statistics
About This Book
A hands-on introduction to computational statistics from a Bayesian point of view
Providing a solid grounding in statistics while uniquely covering the topics from a Bayesian perspective, Understanding Computational Bayesian Statistics successfully guides readers through this new, cutting-edge approach. With its hands-on treatment of the topic, the book shows how samples can be drawn from the posterior distribution when the formula giving its shape is all that is known, and how Bayesian inferences can be based on these samples from the posterior. These ideas are illustrated on common statistical models, including the multiple linear regression model, the hierarchical mean model, the logistic regression model, and the proportional hazards model.
The book begins with an outline of the similarities and differences between Bayesian and the likelihood approaches to statistics. Subsequent chapters present key techniques for using computer software to draw Monte Carlo samples from the incompletely known posterior distribution and performing the Bayesian inference calculated from these samples. Topics of coverage include:
- Direct ways to draw a random sample from the posterior by reshaping a random sample drawn from an easily sampled starting distribution
- The distributions from the one-dimensional exponential family
- Markov chains and their long-run behavior
- The Metropolis-Hastings algorithm
- Gibbs sampling algorithm and methods for speeding up convergence
- Markov chain Monte Carlo sampling
Using numerous graphs and diagrams, the author emphasizes a step-by-step approach to computational Bayesian statistics. At each step, important aspects of application are detailed, such as how to choose a prior for logistic regression model, the Poisson regression model, and the proportional hazards model. A related Web site houses R functions and Minitab macros for Bayesian analysis and Monte Carlo simulations, and detailed appendices in the book guide readers through the use of these software packages.
Understanding Computational Bayesian Statistics is an excellent book for courses on computational statistics at the upper-level undergraduate and graduate levels. It is also a valuable reference for researchers and practitioners who use computer programs to conduct statistical analyses of data and solve problems in their everyday work.
Frequently asked questions
Information
Table of contents
- Cover
- Title Page
- Series Page
- Copyright
- Dedication
- Preface
- 1: Introduction to Bayesian Statistics
- 2: Monte Carlo Sampling from the Posterior
- 3: Bayesian Inference
- 4: Bayesian Statistics Using Conjugate Priors
- 5: Markov Chains
- 6: Markov Chain Monte Carlo Sampling from Posterior
- 7: Statistical Inference from a Markov Chain Monte Carlo Sample
- 8: Logistic Regression
- 9: Poisson Regression and Proportional Hazards Model
- 10: Gibbs Sampling and Hierarchical Models
- 11: Going Forward with Markov Chain Monte Carlo
- A: Using the Included Minitab Macros
- B: Using the Included R Functions
- References
- WILEY SERIES IN COMPUTATIONAL STATISTICS
- Index