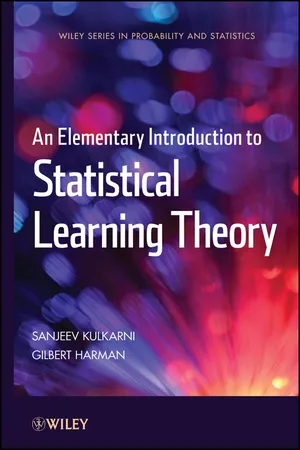
An Elementary Introduction to Statistical Learning Theory
- English
- ePUB (mobile friendly)
- Available on iOS & Android
An Elementary Introduction to Statistical Learning Theory
About This Book
A thought-provoking look at statistical learning theory and its role in understanding human learning and inductive reasoning
A joint endeavor from leading researchers in the fields of philosophy and electrical engineering, An Elementary Introduction to Statistical Learning Theory is a comprehensive and accessible primer on the rapidly evolving fields of statistical pattern recognition and statistical learning theory. Explaining these areas at a level and in a way that is not often found in other books on the topic, the authors present the basic theory behind contemporary machine learning and uniquely utilize its foundations as a framework for philosophical thinking about inductive inference.
Promoting the fundamental goal of statistical learning, knowing what is achievable and what is not, this book demonstrates the value of a systematic methodology when used along with the needed techniques for evaluating the performance of a learning system. First, an introduction to machine learning is presented that includes brief discussions of applications such as image recognition, speech recognition, medical diagnostics, and statistical arbitrage. To enhance accessibility, two chapters on relevant aspects of probability theory are provided. Subsequent chapters feature coverage of topics such as the pattern recognition problem, optimal Bayes decision rule, the nearest neighbor rule, kernel rules, neural networks, support vector machines, and boosting.
Appendices throughout the book explore the relationship between the discussed material and related topics from mathematics, philosophy, psychology, and statistics, drawing insightful connections between problems in these areas and statistical learning theory. All chapters conclude with a summary section, a set of practice questions, and a reference sections that supplies historical notes and additional resources for further study.
An Elementary Introduction to Statistical Learning Theory is an excellent book for courses on statistical learning theory, pattern recognition, and machine learning at the upper-undergraduate and graduatelevels. It also serves as an introductory reference for researchers and practitioners in the fields of engineering, computer science, philosophy, and cognitive science that would like to further their knowledge of the topic.
Frequently asked questions
Information
Table of contents
- Cover
- Series Page
- Title Page
- Copyright
- Preface
- Chapter 1: Introduction: Classification, Learning, Features, and Applications
- Chapter 2: Probability
- Chapter 3: Probability Densities
- Chapter 4: The Pattern Recognition Problem
- Chapter 5: The Optimal Bayes Decision Rule
- Chapter 6: Learning from Examples
- Chapter 7: The Nearest Neighbor Rule
- Chapter 8: Kernel Rules
- Chapter 9: Neural Networks: Perceptrons
- Chapter 10: Multilayer Networks
- Chapter 11: PAC Learning
- Chapter 12: VC Dimension
- Chapter 13: Infinite VC Dimension
- Chapter 14: The Function Estimation Problem
- Chapter 15: Learning Function Estimation
- Chapter 16: Simplicity
- Chapter 17: Support Vector Machines
- Chapter 18: Boosting
- Bibliography
- Author Index
- Subject Index