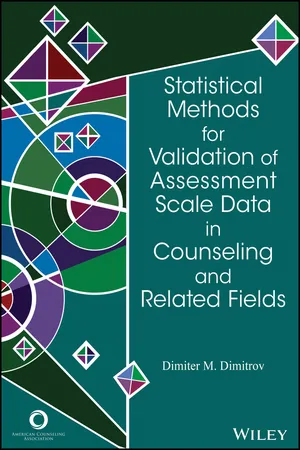
Statistical Methods for Validation of Assessment Scale Data in Counseling and Related Fields
- English
- ePUB (mobile friendly)
- Available on iOS & Android
Statistical Methods for Validation of Assessment Scale Data in Counseling and Related Fields
About This Book
"Dr. Dimitrov has constructed a masterpieceâa classic resource that should adorn the shelf of every counseling researcher and graduate student serious about the construction and validation of high quality research instruments.
âBradley T. Erford, PhD
Loyola University Maryland
Past President, American Counseling Association
"This book offers a comprehensive treatment of the statistical models and methods needed to properly examine the psychometric properties of assessment scale data. It is certain to become a definitive reference for both novice and experienced researchers alike."
âGeorge A. Marcoulides, PhD
University of California, Riverside
This instructive book presents statistical methods and procedures for the validation of assessment scale data used in counseling, psychology, education, and related fields. In Part I, measurement scales, reliability, and the unified construct-based model of validity are discussed, along with key steps in instrument development. Part II describes factor analyses in construct validation, including exploratory factor analysis, confirmatory factor analysis, and models of multitrait-multimethod data analysis. Traditional and Rasch-based analyses of binary and rating scales are examined in Part III.
Dr. Dimitrov offers students, researchers, and clinicians step-by-step guidance on contemporary methodological principles, statistical methods, and psychometric procedures that are useful in the development or validation of assessment scale data. Numerous examples, tables, and figures provided throughout the text illustrate the underlying principles of measurement in a clear and concise manner for practical application.
*Requests for digital versions from ACA can be found on www.wiley.com.
*To purchase print copies, please visit the ACA website here.
*Reproduction requests for material from books published by ACA should be directed [email protected]
Frequently asked questions
Information
Part I
Scales, Reliability, and Validity
Chapter 1
Variables and Measurement Scales
1.1 Variables in Social and Behavioral Research
1.1.1 Observable Versus Latent Variables
1.1.2 Continuous Versus Discrete Variables
Note 1.1
1.2 What Is Measurement?
Table of contents
- Cover
- Title Page
- Copyright
- Dedication
- Preface
- Acknowledgments
- About the Author
- Part I: Scales, Reliability, and Validity
- Part II: Factor Analysis in Construct Validation
- Part III: Psychometric Scale Analysis
- References
- Index
- Technical Support
- End User License Agreement