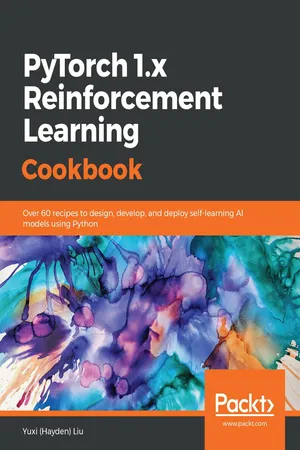
PyTorch 1.x Reinforcement Learning Cookbook
Over 60 recipes to design, develop, and deploy self-learning AI models using Python
Yuxi (Hayden) Liu
- 340 pages
- English
- ePUB (mobile friendly)
- Available on iOS & Android
PyTorch 1.x Reinforcement Learning Cookbook
Over 60 recipes to design, develop, and deploy self-learning AI models using Python
Yuxi (Hayden) Liu
About This Book
Implement reinforcement learning techniques and algorithms with the help of real-world examples and recipes
Key Features
- Use PyTorch 1.x to design and build self-learning artificial intelligence (AI) models
- Implement RL algorithms to solve control and optimization challenges faced by data scientists today
- Apply modern RL libraries to simulate a controlled environment for your projects
Book Description
Reinforcement learning (RL) is a branch of machine learning that has gained popularity in recent times. It allows you to train AI models that learn from their own actions and optimize their behavior. PyTorch has also emerged as the preferred tool for training RL models because of its efficiency and ease of use.
With this book, you'll explore the important RL concepts and the implementation of algorithms in PyTorch 1.x. The recipes in the book, along with real-world examples, will help you master various RL techniques, such as dynamic programming, Monte Carlo simulations, temporal difference, and Q-learning. You'll also gain insights into industry-specific applications of these techniques. Later chapters will guide you through solving problems such as the multi-armed bandit problem and the cartpole problem using the multi-armed bandit algorithm and function approximation. You'll also learn how to use Deep Q-Networks to complete Atari games, along with how to effectively implement policy gradients. Finally, you'll discover how RL techniques are applied to Blackjack, Gridworld environments, internet advertising, and the Flappy Bird game.
By the end of this book, you'll have developed the skills you need to implement popular RL algorithms and use RL techniques to solve real-world problems.
What you will learn
- Use Q-learning and the stateâactionârewardâstateâaction (SARSA) algorithm to solve various Gridworld problems
- Develop a multi-armed bandit algorithm to optimize display advertising
- Scale up learning and control processes using Deep Q-Networks
- Simulate Markov Decision Processes, OpenAI Gym environments, and other common control problems
- Select and build RL models, evaluate their performance, and optimize and deploy them
- Use policy gradient methods to solve continuous RL problems
Who this book is for
Machine learning engineers, data scientists and AI researchers looking for quick solutions to different reinforcement learning problems will find this book useful. Although prior knowledge of machine learning concepts is required, experience with PyTorch will be useful but not necessary.
Frequently asked questions
Information
Markov Decision Processes and Dynamic Programming
- Creating a Markov chain
- Creating an MDP
- Performing policy evaluation
- Simulating the FrozenLake environment
- Solving an MDP with a value iteration algorithm
- Solving an MDP with a policy iteration algorithm
- Solving the coin-flipping gamble problem
Technical requirements
- Python 3.6, 3.7, or above
- Anaconda
- PyTorch 1.0 or above
- OpenAI Gym
Creating a Markov chain
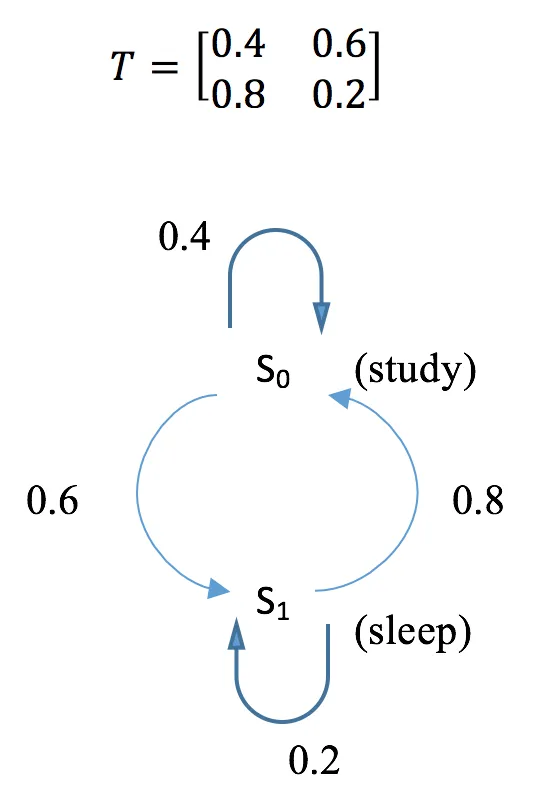
How to do it...
- Import the library and define the transition matrix:
>>> import torch
>>> T = torch.tensor([[0.4, 0.6],
... [0.8, 0.2]])
- Calculate the transition probability after k steps. Here, we use k = 2, 5, 10, 15, and 20 as examples:
>>> T_2 = torch.matrix_power(T, 2)
>>> T_5 = torch.matrix_power(T, 5)
>>> T_10 = torch.matrix_power(T, 10)
>>> T_15 = torch.matrix_power(T, 15)
>>> T_20 = torch.matrix_power(T, 20)
- Define the initial distribution of two states:
>>> v = torch.tensor([[0.7, 0.3]])
- Calculate the state distribution after k = 1, 2, 5, 10, 15, and 20 steps:
>>> v_1 = torch.mm(v, T)
>>> v_2 = torch.mm(v, T_2)
>>> v_5 = torch.mm(v, T_5)
>>> v_10 = torch.mm(v, T_10)
>>> v_15 = torch.mm(v, T_15)
>>> v_20 = torch.mm(v, T_20)
How it works...
>>> print("Transition probability after 2 steps:\n{}".format(T_2))
Transition probability after 2 steps:
tensor([[0.6400, 0.3600],
[0.4800, 0.5200]])
>>> print("Transition probability after 5 steps:\n{}".format(T_5))
Transition probability after 5 steps:
tensor([[0.5670, 0.4330],
[0.5773, 0.4227]])
>>> print(
"Transition probability after 10 steps:\n{}".format(T_10))
Transition probability after 10 steps:
tensor([[0.5715, 0.4285],
[0.5714, 0.4286]])
>>> print(
"Transition probability after 15 steps:\n{}".format(T_15))
Transition probability after 15 steps:
tensor([[0.5714, 0.4286],
[0.5714, 0.4286]])
>>> print(
"Transition probability after 20 steps:\n{}".format(T_20))
Transition probability after 20 steps:
tensor([[0.5714, 0.4286],
[0.5714, 0.4286]])
>>> print("Distribution of states after 1 step:\n{}".format(v_1))
Distribution of states after 1 step:
tensor([[0.5200, 0.4800]])
>>> print("Distribution of states after 2 steps:\n{}".format(v_2))
Distribution of states after 2 steps:
tensor([[0.5920, 0.4080]])
>>> print("Distribution of states after 5 steps:\n{}".format(v_5))
Distribution of states after 5 steps:
te...
Table of contents
- Title Page
- Copyright and Credits
- About Packt
- Contributors
- Preface
- Getting Started with Reinforcement Learning and PyTorch
- Markov Decision Processes and Dynamic Programming
- Monte Carlo Methods for Making Numerical Estimations
- Temporal Difference and Q-Learning
- Solving Multi-armed Bandit Problems
- Scaling Up Learning with Function Approximation
- Deep Q-Networks in Action
- Implementing Policy Gradients and Policy Optimization
- Capstone Project â Playing Flappy Bird with DQN
- Other Books You May Enjoy