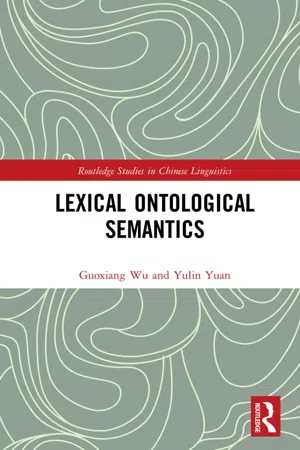
This is a test
- 234 pages
- English
- ePUB (mobile friendly)
- Available on iOS & Android
eBook - ePub
Lexical Ontological Semantics
Book details
Book preview
Table of contents
Citations
About This Book
Lexical Ontological Semantics introduces ontological methods into lexical semantic studies with the aim of giving impetus to various fields of endeavours which envision and model the semantic network of a language. Lexical ontological semantics (LOS) provides a cognition-based computation-oriented framework in which nouns and predicates are described in terms of their semantic knowledge and models the mechanism in which the noun system is coupled with the predicate system. It expands the scope of lexical semantics, updates methodologies to semantic representation, guides the construction of semantic resources for natural language processing, and develops new theories for human-machine interactions and communications.
Frequently asked questions
At the moment all of our mobile-responsive ePub books are available to download via the app. Most of our PDFs are also available to download and we're working on making the final remaining ones downloadable now. Learn more here.
Both plans give you full access to the library and all of Perlegoâs features. The only differences are the price and subscription period: With the annual plan youâll save around 30% compared to 12 months on the monthly plan.
We are an online textbook subscription service, where you can get access to an entire online library for less than the price of a single book per month. With over 1 million books across 1000+ topics, weâve got you covered! Learn more here.
Look out for the read-aloud symbol on your next book to see if you can listen to it. The read-aloud tool reads text aloud for you, highlighting the text as it is being read. You can pause it, speed it up and slow it down. Learn more here.
Yes, you can access Lexical Ontological Semantics by Guoxiang Wu,Yulin Yuan in PDF and/or ePUB format, as well as other popular books in Languages & Linguistics & Linguistics. We have over one million books available in our catalogue for you to explore.
Information
1The semantic network and ontological lexicology
Lexicons and ontologies have been used to model language into a semantic network by means of formalised lexical concepts and structured conceptual relations. The semantic model, as Halliday (2012a, p. 13; 2012b, p. 8) puts it, is âthe semiotic analogue of the interlocking material forcesâ of sophisticated systems. The semantic network can be compared to three of the most complicated systems in human history, that is, the internet, the neural network, and the social network. To an extent, these systems constitute physical, mental, and social foundations on which the semantic system operates. An effective integration of the semantic network and these three networks results in an ever-sophisticated multi-network system in which humans, machines, things and meanings interact.
1.1The internet, the web, and the Internet of Things
The internet is an ever-evolving human invention. It was initiated in 1969 when Advanced Research Projects Agency (ARPA) succeeded in their attempt to connect two computers. The connection was continuously extended by new nodes added to it and gradually transformed into what is referred to as ARPANET in the following few years. By the mid-1970s, ARPA had built up three types of computer networks, that is, ARPANET (connected through telephone lines), PRNET (Pocket Radio Net, connected through radio signals), and SATNET (Satellite Net, connected through satellite communications). The SATNET is a cross-Atlantic network. These three networks were quickly transformed into the internet through two communication protocols: Transmission Control Protocol (TCP) and Internet Protocol (IP). TCP handles communications between machines and IP handles connections between networks. They have been adopted as the internet standard since 1985. It is the mechanism of TCP/IP to work at both levels that gave impetus to the earlier growth of the internet in the late 1980s (Ryan, 2010, p. 44).
In 1990, Tim Berners-Lee developed a language called HTML (HyperText Markup Language). He specified a server to host documents in the HTML format and a client to view them. This is the first instance of the World Wide Web (www or the web) and âone of the most important advances in human communications historyâ (Ryan, 2010, p. 107). With HTML, texts can be marked up with their semantic features. In this way, two strands of information can be distinguished: the texts themselves and their semantic features. When information is displayed in the userâs browser, the texts are explicit whereas their features are implicit. Semantic features are used for semantics-based computational purposes, including information retrieval. The location of a document in the web is reduced into a universal resource identifier (URI address), and different URIs are in turn used as hyperlinks to point to different documents. These hyperlinks are analogous to material relations in the physical world or mental associations in the psychological world. Eventually, the web has transformed the internet from a meta-network of computer networks into a meta-platform of information networks.
Low-cost built-in micro-computers or digital memories have been developed recently to further connect physical objects (e.g., products) and/or social behaviours (e.g., services) into what is referred to as the âcyber-physical systemâ (CPS). This technological innovation involves information exchanges among physical objects through the internet. For example, digital memories are embedded into artefacts in their production processes and logistic services, with the aim of recording relevant events throughout their entire life cycle. Interactions involved in the whole process transform these inter-connected artefacts from passive objects into intelligent agents. CPS has a number of benefits, including product transparency, security, quality, production efficiency, and customisation (Wahlster, 2013, p. 3, 20). Through successful implementations and deployments of CPS into major industrial or service sectors, the internet has been evolving gradually from a meta-platform of information networks into the emerging Internet of Things (IoT). It is foreseeable that machine-machine, machine-human, and human-human interactions thereby further transform the living conditions of human beings.
1.2 Cognitive modelling for the neural network
The neural network tends to be modelled in a centralist, selectionist manner. With regard to how ânectionsâ or nodes are connected with each other in a neural network model, Lamb (1999, p. 329) hesitates to conclude that âall nections of the model correspond directly to cortical columnsâ. In a one-to-three connection, for example, the linking may be either centralised or decentralised. In the centralist hypothesis, one node is connected to the other three through a common link. In the decentralist hypothesis, in contrast, they are connected through three separate links. Lamb holds that, as neurons are prolific in their interactions, the centralist hypothesis is more âmetaphoricalâ than the decentralist hypothesis. That is to say, the centralist model is less likely to conform to cortical columns. This does not mean, however, that the centralist perspective should be avoided, because it serves effectively to simplify any complex model for the neural network.
Similarly, the constructionist and selectionist principles also serve for different purposes. From a constructionist perspective, knowledge is structured in a network manner and to acquire knowledge means to reconstruct the network, so knowledge is best to be modelled in the way nodes and connective lines are constructed in real time. From a selectionist perspective, in contrast, because the human brain is regarded as an atomic whole, in which existing nodes and latent connections are selected rather than being constructed, researchers can be excused from providing evidence for the actual neurological structure. As a result, although a simplified selectionist model may deviate from the actual mapping between knowledge structure and cortical structure, it is more practical than a constructionist model (Lamb, 1999, p. 322).
Recent studies hold that, although human brains conceptualise thousands of distinct nominal or verbal categories, to assign distinct cortical spaces for each of these lexical categories is inefficient, even if human brains are likely to have this capacity. With functional magnetic resonance imaging (fMRI), Huth et al. (2012) measure human brain activities evoked by 1,705 lexical categories taken from a lexical database (WordNet, which will be discussed later in this chapter) and conclude that a more effective way is to have a dual scheme: lexical categories are first evenly mapped onto a semantic space and this continuous semantic space is in turn mapped onto a smooth cortical surface. In this dual scheme, the semantic space serves as a common operating interface which mediates the lexical categories and the cortical structures. Principal component analysis (PCA) is used to ensure the projection of the cortical surface onto the semantic space. Huth et al. define nine semantic dimensions, including mobileâimmobile, humansânon-humans, socialânon-social, civilisationânature, animalânon-animal, biologicalânon-biological, placeânon-place, and real-world size. In this way, the semantic space becomes a cognitive interface through which the lexical network is mapped onto the neural network.
1.3 The social network
Human activities are of social significance. Inspired by Goffmanâs (1963) âbodily activityâ, Gu (2009, p. 436) uses the term âtotal saturated experienceâ (TSE) to refer to experience which is fully immersed in a real-world social interaction. This interactive experience among co-present individuals not only embodies messages but also constructs meanings. The totality of meanings constructed by TSE is referred to as âtotal saturated significanceâ (TSS). TSE, however, is not the only way to shed light on TSS. A copy of TSS can also be reconstructed through written language, spoken language, videos, or relevant media.
None of these modes is adequate, however â written language can âcapture faithfully some aspects of verbal behaviourâ but leaves others not encoded; videos are âundoubtedly the most powerfulâ, but they still fail to produce âa full copy of the original TSSâ (Gu, 2009, p. 437). The audio mode is located somewhere in between. Because these three modes are not exactly complementary with each other, even if they are combined into a multi-modal corpus, TSS cannot be fully recovered. For example, when a multi-modal corpus is used to document a situation in which family members assume posture for photo-taking, the following narrative can serve as compensation for certain information loss: â⊠senior members should be positioned in the centre of the front row ⊠the less senior ones spreading sidewise from the centre to both directionsâ (Gu, 2009, p. 443). This kind of narration adds social-cognitive information to the multi-modal TSS and this fuller copy may be sufficient for general purposes, but it is still inadequate for specific purposes in linguistic studies, especially insufficient for language-related applications that are developed by computer programmers. To reconstruct âa full copyâ of the original TSS, Gu proposes an Agent-Oriented Model (AOM) to substitute for the multi-modal corpus.
AOM âtranslatesâ the photo-taking activity into a photo-taking model. In Guâs sample model, eight family members are involved and only two chairs are provided. This narrative provides the convention in conformity to which family members assume their positions, including who are to sit on the chairs or stand by and which chair they should take or where they should stand. Accordingly, the model comprises three key modules: (i) the agent module, in which properties of different agents and their potential behaviours are defined; (ii) the behaviour module, in which properties of different behaviours and their entry conditions are specified; and (iii) the agent-behaviour interaction module, which includes how agents and behaviours potentially interact with each other. In this way, the photo-taking activity is transformed into AOM. The concept of agent plays a central role in this model â different agents are connected to each other by means of their behaviours and these connections correspond to a network of social interactions. AOM is twofold in nature: a real-world situation is transformed into a well-defined information system and the system in turn reconstructs social significance.
The implementation and application of AOM produces a set of technical terms and rules which is referred to as Agent-Oriented Model Language (AOML). AOML enables linguists to document available resources in a machine-readable format.
1.4 The semantic network: Lexicons and ontologies
The Internet of Things (IoT) is a network composed of objects and their relations; the neural network is composed of neurons and their connections; the social network is modelled in terms of agents and their interactions. Similarly, the semantic network is commonly modelled in terms of lexical concepts and their relations.
Lexical information has been well documented in traditional dictionaries, including: lexical items and their phonetic features, morphological forms, syntactic collocations, and semantic characteristics. Semantic interactions in context, however, are not easy to describe in a systematic manner, not to mention semantic association. Unexceptionally, it is dictionary users who make use of both linguistic knowledge and world knowledge to discover the semantic network in which lexical interaction and semantic association are involved. In An Analytical Dictionary of Charactersâ (èŻŽæè§Łć ShuĆ WĂ©n JiÄ ZĂŹ), for example, the character è huĂŹ is defined as âthe cover term of various grassesâ (èžäčæ»ć cÇo zhÄ« zÇng mĂng). With this piece of lexical information, dictionary users can first build up a familiar semantic association and then remove the sense of strangeness from this character. Generally speaking, lexicographers document lexical information into dictionaries, whereas lexicologists focus more on semantic association in context or in use. Digital technologies weaken this division of labour, so lexical information and semantic association can be integrated into a unified lexical database, for example, a âproduction dictionaryâ (Carter, 1998/2012, pp. 174â5).
In recent studies of âontological lexicographyâ, ontologies have been integrated with lexicons. These efforts have brought about a number of ontological lexicons, lexical ontologies, or interfaces between lexicons and ontologies (that is, âontolexâ interfaces; PrĂ©vot et al., 2010/2014, p. 9), including: WordNet (a lexical database for English), Chinese Conceptual Dictionary (CCD), HowNet (HowNet Knowledge Database), and Sinica BOW (Bilingual Ontological Word Net).
1.4.1 WordNet and Chinese Conceptual Dictionary
WordNet, housed in the Cognitive Science Laboratory at Princeton University, is an electronic database for the English lexical system. It draws on the theory of mental lexicon in psychology and provides a consistent, formal model for linguistic knowledge. Like a thesaurus, sets of synonyms (or synsets) are taken as basic organising units. Words in a synset encode the same concept, so they are âcognitively equivalent synonymsâ (Pease and Fellbaum, 2010, p. 25). If one of these words is retrieved, all of the other words that express the concept can be retrieved. WordNet (version 3.0) contains over 117,000 synsets. These synsets are interlinked into a semantic network by a number of semantic relations, incl...
Table of contents
- Cover
- Half Title
- Series Information
- Title Page
- Copyright Page
- Contents
- List of illustrations
- Preface
- Abbreviations
- Introduction: From lexis to lexical ontological semantics
- 1 The semantic network and ontological lexicology
- 2 A qualia-structure descriptive system for nouns
- 3 An argument-structure descriptive system for predicates
- 4 The QS-AS coupling mechanism
- 5 A corpus-based lexicon-construction interactive model
- 6 Automatic restoration of implicit predicates
- 7 Qualia structure and translated text analysis
- 8 Conclusion
- References
- Index