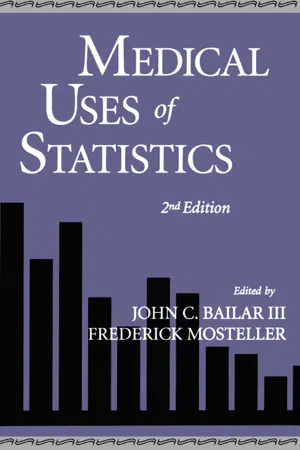
This is a test
- 480 pages
- English
- ePUB (mobile friendly)
- Available on iOS & Android
eBook - ePub
Medical Uses of Statistics
Book details
Book preview
Table of contents
Citations
About This Book
This work explains the purpose of statistical methods in medical studies and analyzes the statistical techniques used by clinical investigators, with special emphasis on studies published in "The New England Journal of Medicine". It clarifies fundamental concepts of statistical design and analysis, and facilitates the understanding of research results.
Frequently asked questions
At the moment all of our mobile-responsive ePub books are available to download via the app. Most of our PDFs are also available to download and we're working on making the final remaining ones downloadable now. Learn more here.
Both plans give you full access to the library and all of Perlegoâs features. The only differences are the price and subscription period: With the annual plan youâll save around 30% compared to 12 months on the monthly plan.
We are an online textbook subscription service, where you can get access to an entire online library for less than the price of a single book per month. With over 1 million books across 1000+ topics, weâve got you covered! Learn more here.
Look out for the read-aloud symbol on your next book to see if you can listen to it. The read-aloud tool reads text aloud for you, highlighting the text as it is being read. You can pause it, speed it up and slow it down. Learn more here.
Yes, you can access Medical Uses of Statistics by Bailar/Mostelle in PDF and/or ePUB format, as well as other popular books in Mathematics & Mathematics General. We have over one million books available in our catalogue for you to explore.
Information
SECTION III

Analysis
9

DECISION ANALYSIS
Excellent clinical judgment requires optimal decision making. Many of the decisions that physicians make in their practices involve little uncertainty and little risk: these rote or routine choices need no special contemplation because they are âtried and trueâ practices. But for each routine problem there are several for which no easy solution is at hand. To deal with these, the tough problems, a physician can search for a properly designed, double-blind controlled study that examined patients of the same age, sex, and race and with the same conditions in the same stage; use an algorithm developed for such patients; use the problem-oriented approach to data gathering and hope that the solution to the problem will emerge; or ask for the help of one or more consultants. The frustrations encountered with each of these approaches are familiar.
For 15 years we and others have been developing decision analysis and applying it to difficult clinical problems,16 and after experience with several hundred such analyses tailored to individual patients,7 we are convinced that this quantitative approach warrants careful consideration as a tool for making decisions not only for individual patients but also for classes of clinical problems. This actively evolving field provides insights not available from clinical studies or expert opinion.
In this chapter we provide a few examples of some advances in the methods and the application of decision analysis. We consider both the advantages of the method and its limitations and offer our thoughts about the extent of the dissemination of decision analysis in medicine.
BAYESâ RULE
The modern physician is inundated by data â both clinical information that has been obtained intentionally and unanticipated results of screening tests and imaging procedures. In most circumstances, clinical information does not establish diagnoses with certainty; instead, each finding allows the physician to revise the probability of various diagnostic alternatives. In this sequential, iterative process, three sets of probabilities are defined: (1) the probabilities of the diagnoses before the presence of a new finding is revealed (prior probabilities); (2) the probabilities that a given finding is observed in each disorder diagnosed (conditional probabilities); and (3) the probabilities of the diagnoses after the presence of a new finding is revealed (posterior or revised probabilities). The terms âpriorâ and âposteriorâ are defined with respect to a given diagnostic finding. In the sequential diagnostic process, the posterior probabilities for one finding become the prior probabilities for the next. Thus, the diagnostic implications of a given test result vary from patient to patient, depending on the presence of other findings.
A mathematical combination of prior and conditional probabilities produces posterior probabilities. The relation among the three sets of probabilities â Bayesâ rule â has been understood for two centuries, but this formulation has been applied to clinical reasoning only in the past several decades. Initial applications of Bayesâ rule presented the physician with an equation* or with a computer program8, 9, 10, 11, 12 that had the characteristics of a âblack box.â Other popular alternatives to the formal equation have been introduced, including nomograms13, 14, 15 and tables.16 Unfortunately, these latter approaches are practical only when a single disease is considered to be either present or absent and when the test result can be considered to be binaryâ i.e., either positive or negative. Recently, two different tabular formulations of Bayesâ rule have appeared: a two-by-two table,5,17 best used for calculating measures of test performance in a given study, and a posterior-probability calculator, designed to provide the interpretation of test information in a given clinical setting.18,19 The latter technique can be used when several alternative diagnoses are possible and when test results lie along a continuum â e.g., serum enzyme levels. The calculation is performed easily with pencil and paper or with a hand-held calculator. With the almost ubiquitous availability of personal computers and spreadsheet programs, templates for this calculation are easily created. Table 1 demonstrates the use of a spreadsheet to interpret the results of preoperative dipyridamoleâthallium perfusion scanning in a 67-year-old man with peripheral vascular disease. This formulation of Bayesâ rule uses a table with five columns: Column A, a list of mutually exclusive and exhaustive diagnoses; Column B, the prior probability of each diagnosis; Column C, the conditional probability of the observed finding, given each diagnosis; Column D, the product of Columns B and C and the total of all of these products; and Column E, the posterior probability, which is calculated by dividing each product in Column D by the sum of the products. A negative dipyridamoleâthallium test diminishes the likelihood of critical coronary disease in this patient from 10 percent to less than 2 percent (Table 1), but almost half of comparable patients with such a test result will nonetheless have clinically important disease.
* where Pdis i denotes the prior probability of disease i, Pfindldis i the conditional probability of the finding in patients with disease i, and Pdis i find the posterior probability of disease i, given the presence of the finding. The particular disease, among the i possible diseases, is denoted as dis.
Table 1. Spreadsheet Template Using Bayesâ Rule to Interpret a Negative Thailium Test.*
AâDiagnosis: Coronary Artery Disease | B â Prior Probability percent | C â Conditional Probability of Negative Scan percent | D â Product (B Ă C) | E â Posterior Probability (100 Ă D/Sum) percent |
Critical | 10 | 5 | 50 | 1.6 |
Noncritical | 70 | 20 | 1400 | 43.6 |
Negligible | 20 | 88 | 1760 | 54.8 |
3210 |
* This template can be easily built on any standard spreadsheet program.
This simple technique can help the physician avoid the common reasoning error of neglecting the base rate.20, 21, 22 In fact, because of the availability of probabilistic data in the medical literature and because clinicians are taught to quote and rely on the literature, this type of reasoning error is quite prevalent. Such errors are most likely to arise when the diagnostic test provides an unexpected result; the unwary clinician may rely too heavily on a highly âaccurateâ diagnostic test, neglecting the critical influence of disease prevalence.
DECISION TREES
Decision analysis, a derivative of operations research and game theory, involves identifying all available choices and the potential outcomes of each and structuring a model of the decision, usually in the form of a decision tree. Such a tree consists of nodes, which describe choices, chances, and outcomes. The tree is used to represent the strategies available to the physician and to calculate the likelihood that each outcome will occur if a particular strategy is employed. The relative worth of each outcome is also described numerically, as a utility, on an explicitly defined scale â e.g., a life expectancy of 17 years, or a score of 50 on a scale on which immediate death is defined as 0 and normal life expectancy in good health is defined as 100. The utility of a chance node is calculated as the weighted average of the utilities of its possible outcomes, where the weights are the probabilities that each outcome will occur. For example, a chance node describing a 5 percent chance of immediate death (with a life expectancy of 0), a 20 percent chance of survival with disabling angina (with a life expectancy of 7 years), and a 75 percent chance of survival free of angina (with a life expectancy of 15 years) would represent a life expectancy of 12.65 years, i.e., (5% Ă 0) + (20% Ă 7)+ (75% Ă 15). The utility of a decision node is the maximum of the utilities of its component strategies, since the rational decision maker should choose the alternative that, on average, provides the highest value. Even when objective data are not available from the literature or from local experience,...
Table of contents
- Cover
- Half Title
- Title Page
- Copyright Page
- Dedication
- Table of Contents
- Contributing Authors
- Preface
- Preface to the First Edition
- Acknowledgments
- Origins of Chapters
- Introduction
- SECTION I BROAD CONCEPTS AND ANALYTIC TECHNIQUES
- SECTION II DESIGN
- SECTION III ANALYSIS
- SECTION IV COMMUNICATING RESULTS
- SECTION V REVIEWS AND META-STUDIES
- INDEX