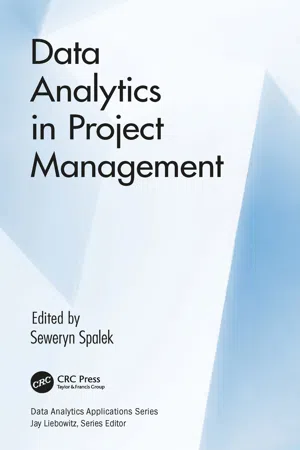
This is a test
- 222 pages
- English
- ePUB (mobile friendly)
- Available on iOS & Android
eBook - ePub
Data Analytics in Project Management
Book details
Book preview
Table of contents
Citations
About This Book
This book aims to help the reader better understand the importance of data analysis in project management. Moreover, it provides guidance by showing tools, methods, techniques and lessons learned on how to better utilize the data gathered from the projects. First and foremost, insight into the bridge between data analytics and project management aids practitioners looking for ways to maximize the practical value of data procured. The book equips organizations with the know-how necessary to adapt to a changing workplace dynamic through key lessons learned from past ventures. The book's integrated approach to investigating both fields enhances the value of research findings.
Frequently asked questions
At the moment all of our mobile-responsive ePub books are available to download via the app. Most of our PDFs are also available to download and we're working on making the final remaining ones downloadable now. Learn more here.
Both plans give you full access to the library and all of Perlegoâs features. The only differences are the price and subscription period: With the annual plan youâll save around 30% compared to 12 months on the monthly plan.
We are an online textbook subscription service, where you can get access to an entire online library for less than the price of a single book per month. With over 1 million books across 1000+ topics, weâve got you covered! Learn more here.
Look out for the read-aloud symbol on your next book to see if you can listen to it. The read-aloud tool reads text aloud for you, highlighting the text as it is being read. You can pause it, speed it up and slow it down. Learn more here.
Yes, you can access Data Analytics in Project Management by Seweryn Spalek, Seweryn Spalek in PDF and/or ePUB format, as well as other popular books in Business & Finance. We have over one million books available in our catalogue for you to explore.
1
Introduction
Seweryn Spalek
Data analytics (DA) plays a crucial role in business analytics. Without a rigid approach to analyzing data, we wouldnât be able to glean insights from it. According to Evan Stubbs,* strong analytical abilities are a prerequisite to taking action and creating change, which then must be followed by identification, communication, delivery, and measurement of the value of business analytics. Furthermore, data is usually produced in massive amounts nowadays, and that tendency will continue to increase dramatically. This data production on a massive scale also happens with the Internet of Things (IoT), and, as we near the fourth industrial revolution, the number of sources of data is soaring. Machines connected through the Cloud will create large amounts of data associated with their daily tasks. Moreover, the IoT, interacting with humans, will add additional sets of information. A digital transformation without numerous flows of data would be impossible. This means that projects will be surrounded by or even immersed in oceans of data. Therefore, we need, more urgently than ever, to have mechanisms in place that are able to acquire this data, and then clean, aggregate, and present it. This process is often called the management of data. Then analytics comes in with models and analysis, moving toward a final interpretation. Hence, DA is an extremely important and central part of the entire process. It should, in short, be seen as a driver leading to change, as shown in Figure 1.1.
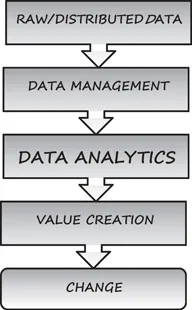
FIGURE 1.1
The key role of data analytics in the process of driving change in project management.
The key role of data analytics in the process of driving change in project management.
Business analytics ensures the expected value of change, while that change is implemented by projects in the business environment. With the significant increase in the number of projects and the amount of data associated with them, it is crucial to understand the areas in which DA can be applied in project management (PM). Therefore, in this book, we address DA in relation to key areas, approaches, and methods in PM.
Thus, in Chapter 2, J. Davidson Frame and Yanping Chen, in an excellent way, address the question: Why data analytics in project management? They note the differences between ancient and modern data analyses. Then they show the evolutionary path from Analytics 1.0 to Analytics 2.0 and reveal that it is necessary for PM DA to combine two inputs coming from business analytics and PM metrics. This means not only relying on historical, real-time, and prescriptive data, but also on strategic key performance indicators (KPIs), financials, marketing, operations, supply chains, and external factors. Moreover, this means depending on planning and controlling the data concerning schedules, budgets, specs, resources, and work performance. The authors address the importance of the data warehouse in the entire analytical process and outline its configuration. Moreover, they present the value that project DA brings to the key players: project manager, project office, chief operating officer, and the executive committee. Frame and Chen include relevant examples and business cases to better explain their reasoning, especially when describing descriptive, predictive, and prescriptive DA. At the end of the chapter, the authors outline the vision (or near future?) of Analytics 3.0, including the key aspects of real time, the tracking of trillions of data points, artificial intelligence, and machine learning.
Risk is associated with any type of activity while managing projects. We can ignore risk, but it will not ignore us. Therefore, Carl Pritchard, in Chapter 3, addresses this important topic in relation to DA in PM. He describes the tolerance issue and its implications. Moreover, he outlines the qualitative approach to data, asking the question: How big is big? He also examines the main sources of bias in data flows. Thus, he discusses the issues associated with data sourcing and its consistency. Further, he explains the concepts of exploratory, confirmatory, and predictive risks in DA. Finally, Pritchard discusses the very important topic of communicating results and the risks associated with them. He points out that the timing of the former is crucial, as well as the means of communication, and underlines that communication, if done improperly, can even be harmful. All of this is explored in an engaging narrative style, aided by a plethora of excellent cases.
The amount of data associated with projects is enormous nowadays and will likely soar. Therefore, there is a need to somehow systematize company data flows, and it appears that the project management office (PMO) is best positioned to oversee this process. This idea is described in Chapter 4. To cope with new challenges, PMOs need to evolve from their current stages toward analytical maturity in the areas of methods and tools, human resources, project environments, and project knowledge management. As data can be obtained from different sources, the concept of the PMO as a multilevel data analysis center is presented. Moreover, the crucial issue of delivering data to the project, portfolio, and organization outcome levels is discussed. As each project interacts with the operations, tactics, and strategies of a company, that issue is outlined, as well. Finally, the concept of the PMO as the centralized office for data flows in a multidimensional project and company environment is proposed.
Projects nowadays are no longer treated by organizations as single, separate endeavors. Companies group them together in portfolios according to the value they should bring to the organization. This creates additional sets of data, which should be analyzed properly. Therefore, Alfonso Bucero focuses on applying DA in project portfolio management (PPM) in a lively and engaging manner. He argues that the focus should be on four dimensions for portfolio optimization: cost value, resources, schedules, and work type. To make this a reality, he proposes the application of a five-step methodology, consisting of the following stages: define, adapt, visualize, assess, and recommend. Furthermore, he explains why DA is key for the decision-making process in PPM and how it can cooperate with business performance management (BPM). Finally, he outlines the issue of DA and project portfolio performance.
When discussing DA, the earned value method (EVM) definitely should be mentioned since EVM is widely recognized and used in PM. This topic is covered by Werner Meyer in Chapter 6. Using a very good and clear approach, he explains such terms as budget at completion, planned value, actual cost, and, finally, earned value. All of these terms belong to descriptive EVM, while predictive EVM covers estimate at completion, estimate to complete, variance at completion, to complete performance index, and time estimate at completion indicators. Furthermore, the interpretation of EVM results is explained. Finally, Werner Meyer highlights the pitfalls of using EVM.
The concept of âbig dataâ occurs in more and more situations these days. Therefore, it is crucial to discuss this concept in the context of PM. Thus, in Chapter 7, Ryan Legard at first explains what attributes differentiate big data from other types of data. Demonstrating his mastery of the subject he discusses, on a step-by-step basis, how to handle big data in projects. In doing so, he distinguishes between three general types or categories of data: structured, unstructured, and semistructured. He proposes a scheme for pairing data with analytical methods taking into account storage technologies. Moreover, he provides advice on how to establish proper controls in order to use the effects of big data analysis as success enablers. He covers important topics such as the means with which to communicate value statements and the chief data officer, a new job title across many organizations. Furthermore, Ryan Legard elaborates on biases in data collection, noting that they are most common during the early stages of organizational and data analysis maturity. Finally, he proposes resolutions for common big data issues.
Managing data cannot be done without information technology (IT) support, which is currently present in many aspects of a companyâs activities. Therefore, Michael Bragen competently guides the reader through the main ideas and outlines the key characteristics which type of software should have to support DA in Chapter 8. He underlines the need for functionality in terms of early warning signs and associated metrics. Then, based on an example of an online system used for visibility and control, he presents different dashboard scenarios. He also discusses an enterprise governance system based on an interesting case study. As it is crucial that each IT system is located within a company, he presents a list of implementation challenges and how to deal with them. Finally, he outlines the future of automated analytical tools, geared toward artificial intelligence operations technology.
Managing projects means making decisions. This process can be supported by data mining, which is the selection, refinement, and analysis of data in order to make decisions or resolve some type of problem. Klas Skogmar, in Chapter 9, capably tackles the key issues via cogent analyses and clear understanding on this important topic by, first, explaining the difference between âdoing the right thingâ and âdoing things right.â Then, he discusses the five aspects of bad decisions, which are biases, bad data, wrong data, too much data, and not using the data. Among these many important aspects, he explains the âbias biasâ phenomenon. Moreover, he discusses how to make good decisions and provides three steps with which to improve the way decisions are made. Next, Skogmar explains how to conduct data mining in four steps, starting with the collection of data, then moving on to mining the information, regression analysis, and, finally, presenting and concluding. Furthermore, he discusses how to extend beyond traditional data mining techniques to improve project success rates. His point is that, in order to do so, unconventional methods should be utilized. He proposes using (1) the lean startup best practice framework or (2) reinforcing or steering behavior. Finally, he explores the possibility of using machine learning methods, or artificial intelligence, to aid in decision-making.
In todayâs turbulent environment, an increasing number of projects are run using the agile approach. Although developed for IT, this approach is being adapted by other industries with progressive success. Thus, Deanne Larson, in Chapter 10, shows her prowess by skillfully addressing, in a straightforward fashion, the important issues of agile PM and DA. At first, she describes the change in the data landscape that has occurred over time, focusing on three data characteristics: volume, variety, then velocity and veracity. Moreover, noting that existing approaches need to be more dynamic, she discusses the methods used in DA projects. Then, she goes into detail concerning agile software development (ASD) principles and CRISP-DM, a data mining process model that describes conceptually the stages that are used to tackle data mining problems. She goes on to discuss the alignment of ASD values and CRISP-DM, as well as the challenges of ASD as applied to DA. Next, she describes best practices in DA PM, starting with the importance of due diligence in defining business goals and problem statements. Further, she examines the issue of underestimating the time required to acquire data for a project and the technical tools that are needed for analytical modeling and data wrangling. Finally, Larson spells out future trends and efforts in the agile approach and DA.
Since Scrum is currently the most popular agile software development method, Brijs Bert sensibly takes a practical approach in Chapter 11 to the issue and goes into this method in some detail. Being aware of the challenging nature of his chapter, he likens combining DA and Scrum as something akin to mixing oil and water together. However, what, at first, seems to be an insurmountable task proves to be well within his grasp. He begins by explaining online transactional processing (OLTP) and online analytical processing (OLAP). He then describes the contrasts between OLAP and OLTP with respect to DA. In addition, he discusses the type of business intelligence (BI) project that may provide a project manager with analytical support. Finally, based on his considerations, he proposes the use of the Sprint Minus One approach in setting up an analytical model, just as a Sprint Zero approach is used for setting up the technical environment.
DA in PM is of increasing importance but extremely challenging. On the one hand, there is rapid multiplication of data volumes, and, on the other hand, the structure of the data is more and more complex. Digging through exabytes of data currently and zettabytes in the close future is a technological challenge in and of itself. When the basic needs of PM to create value are placed on top of that, we face a real concern. In this book, we try to address the most common issues of applying DA in PM. Through supporting theory with numerous examples and case studies, we would like this book to be both valuable for academics and practitioners alike. Although we are aware that the topic of DA in PM is much wider than the topics presented in this book, we hope that we will at least shed some light on the topic by discussing the current state of applications of DA in PM and future developments.
* The value of business analytics, in: Business Analytics. An Introduction. Jay Liebowitz (ed.), CRC Press, Taylor & Francis Group, Boca Raton, FL.
2
Why Data Analytics in Project Management?
J. Davidson Frame and Yanping Chen
CONTENTS
Taking Root
The View from 10,000 Meters
Data Warehousing
Project Manager
Project Office
Chief Operating Officer (COO)
Executive Committee
Descriptive, Predictive, and Prescriptive Data Analytics
Data Analytics 3.0?
Once Again: Why Data Analytics in Project Management?
References
Broadly speaking, data analytics entails the systematic use of data to guide decision-making in organizations. It requires acquiring pertinent data, organizing it, discerning meaningful patterns in it, and determining how the newly gained insights can guide decision-making. By following this process, decision makers minimize reliance on guesswork and gut feeling and engage in evidence-based decision-making.
This chapter addresses the question, why data analytics in project management?
In a broad sense, data analytics is not new to project management. Consider the Giza pyramid, which was built 4,500 years ago. Given the enormity of the construction effort and the astonishing logistical challenges of quarrying, cutting, shipping, and emplacing some 2.3 million stone blocks, each weighing 2.5 to 15 tons, it is clear that the pyramid builders engaged in some sort of analysis that entailed a review of historical data coupled with making estimates of material and logistical requirements for the new structure. Since the time of the Giza pyramid through the nineteenth century, many major construction projects have been carried out, each of which required sorting through data and using the information gleaned from it to plan and implement the work effort. Some of the information was historical, which reflected learning from past project experiences, good or bad, and some new efforts, capturing the unique requirements of the current project.
What is new since the mid-twentieth century is the conscious collection, documentation, and employment of data for the purpose of executing projects effectively. Until fairly recently, data-driven learning was captured in the heads of the players through apprenticeship. On a large project, a highly experienced master-builder would play the role of a project manager, and a collection of various workers would serve under him. They learned their trade on the job, and as they assumed senior roles themselves, they would pass their learning on to the next generation of apprentices.
This chapter examines the evolution of data analytics employed in projects, from the 1950s through today. Following Davenport (2013), it notes that data analytics has gone through two major stages: before big data (1950s through mid-2000s, which we term Analytics 1.0) and after big data (mid-2000s through today, which we call Analytics 2.0). With Analytics 1.0, decision makers employed whatever data that was available to inform their decision-making. At the outset, the data focused on internal operations. What materials were available to produce something? How long did the production effort take? What did it cost? Analysis of the data was chiefly directed at employing it to improve the operations of the enterprise. Data collection and analysis were largely serendipitous with little consideration for collecting and integrating metrics across the full range of the business of the enterprise. The data used was the data that happened to be captured. On occasions when business entities used data to improve the management of their operations, their findings were constrained by the data that was available. Still, even in this primitive situation, the decisions that were made informed by data. In a sense, it could be assumed that data analytics has been employed in project management since the time of the pyramids.
Analytics 2.0 is built on the foundation established by Analytics 1.0, b...
Table of contents
- Cover
- Half Title
- Series Page
- Title Page
- Copyright Page
- Table of Contents
- About the Editor
- Contributors
- Chapter 1 Introduction
- Chapter 2 Why Data Analytics in Project Management?
- Chapter 3 Data Analytics Risk: Lost in Translation?
- Chapter 4 Analytical Challenges of a Modern PMO
- Chapter 5 Data Analytics and Project Portfolio Management
- Chapter 6 Earned Value Method
- Chapter 7 How to Manage Big Data Issues in a Project Environment
- Chapter 8 IT Solutions of Data Analytics as Applied to Project Management
- Chapter 9 Conventional and Unconventional Data Mining for Better Decision-Making
- Chapter 10 Agile Project Management and Data Analytics
- Chapter 11 Data Analytics and Scrum
- Index