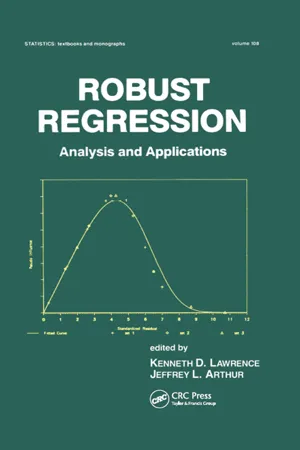
This is a test
- 310 pages
- English
- ePUB (mobile friendly)
- Available on iOS & Android
eBook - ePub
Book details
Book preview
Table of contents
Citations
About This Book
Robust Regression: Analysis and Applications characterizes robust estimators in terms of how much they weight each observation discusses generalized properties of Lp-estimators. Includes an algorithm for identifying outliers using least absolute value criterion in regression modeling reviews redescending M-estimators studies Li linear regression proposes the best linear unbiased estimators for fixed parameters and random errors in the mixed linear model summarizes known properties of Li estimators for time series analysis examines ordinary least squares, latent root regression, and a robust regression weighting scheme and evaluates results from five different robust ridge regression estimators.
Frequently asked questions
At the moment all of our mobile-responsive ePub books are available to download via the app. Most of our PDFs are also available to download and we're working on making the final remaining ones downloadable now. Learn more here.
Both plans give you full access to the library and all of Perlego’s features. The only differences are the price and subscription period: With the annual plan you’ll save around 30% compared to 12 months on the monthly plan.
We are an online textbook subscription service, where you can get access to an entire online library for less than the price of a single book per month. With over 1 million books across 1000+ topics, we’ve got you covered! Learn more here.
Look out for the read-aloud symbol on your next book to see if you can listen to it. The read-aloud tool reads text aloud for you, highlighting the text as it is being read. You can pause it, speed it up and slow it down. Learn more here.
Yes, you can access Robust Regression by Kenneth D. Lawrence in PDF and/or ePUB format, as well as other popular books in Mathematics & Mathematics General. We have over one million books available in our catalogue for you to explore.
Information
Part I
Advances in Robust Regression
1
The Weighting Pattern of a Bayesian Robust Estimator
1. INTRODUCTION
Data has frequently been analyzed as if, to an adequate approximation, errors are normally, identically, and independently distributed. Because it has come to be believed that the first two of the assumptions are frequently inappropriate and in fact that error distributions are likely to be leptokurtic and/or contaminated by occasional bad values giving rise to outliers, attention has been directed, in particular by Huber (1972), Andrews et al. (1972), Hogg (1974), and Barnett and Lewis (1978), to various estimators which are insensitive to such departures.
Such procedures usually give smaller weights to observations that appear discrepant and so can be characterized by their weighting patterns.
In Sections 2 and 3 the structure of certain robust Bayesian estimators is examined from this point of view, and in Section 4 the weighting pattern for a robust Bayesian posterior mean is compared with those for some M-estimators.
2. A BAYESIAN APPROACH TO ROBUST ESTIMATION
Consider the linear model
where Υ is an n × 1 vector of observations, Χ an n × ℓ matrix of fixe...
Table of contents
- Cover
- Half Title
- Title Page
- Copyright Page
- Preface
- Table of Contents
- Contributors
- Part I Advances in Robust Regression
- Part II Robust Regression Methods
- Part III Forecasting and Robust Regression
- Part IV Robust Ridge Regression
- Index