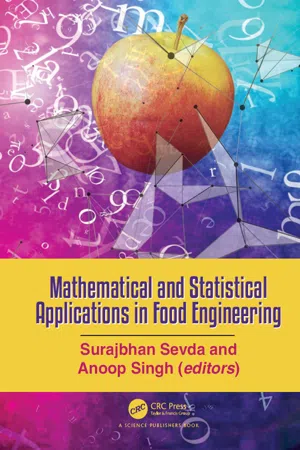
eBook - ePub
Mathematical and Statistical Applications in Food Engineering
This is a test
- 434 pages
- English
- ePUB (mobile friendly)
- Available on iOS & Android
eBook - ePub
Mathematical and Statistical Applications in Food Engineering
Book details
Book preview
Table of contents
Citations
About This Book
Written by experts from all over the world, the book comprises the latest applications of mathematical and models in food engineering and fermentation. It provides the fundamentals on statistical methods to solve standard problems associated with food engineering and fermentation technology. Combining theory with a practical, hands-on approach, this book covers key aspects of food engineering. Presenting cuttingedge information, the book is an essential reference on the fundamental concepts associated with food engineering.
Frequently asked questions
At the moment all of our mobile-responsive ePub books are available to download via the app. Most of our PDFs are also available to download and we're working on making the final remaining ones downloadable now. Learn more here.
Both plans give you full access to the library and all of Perlegoâs features. The only differences are the price and subscription period: With the annual plan youâll save around 30% compared to 12 months on the monthly plan.
We are an online textbook subscription service, where you can get access to an entire online library for less than the price of a single book per month. With over 1 million books across 1000+ topics, weâve got you covered! Learn more here.
Look out for the read-aloud symbol on your next book to see if you can listen to it. The read-aloud tool reads text aloud for you, highlighting the text as it is being read. You can pause it, speed it up and slow it down. Learn more here.
Yes, you can access Mathematical and Statistical Applications in Food Engineering by Surajbhan Sevda, Anoop Singh, Surajbhan Sevda, Anoop Singh in PDF and/or ePUB format, as well as other popular books in Mathematics & Applied Mathematics. We have over one million books available in our catalogue for you to explore.
Information
CHAPTER 1
Role of Mathematical and Statistical Modelling in Food Engineering
Surajbhan Sevda,1,* Vijay Kumar Garlapati2 and Anoop Singh3
1 Department of Biosciences and Bioengineering, Indian Institute of Technology Guwahati, Guwahati, India.
2 Department of Biotechnology and Bioinformatics, Jaypee University of Information Technology, Wakhanaghat-173234, Himachal Pradesh, India.
3 Government of India, Ministry of Science and Technology, Department of Scientific and Industrial Research (DSIR), Technology Bhawan, New Mehrauli Road, New Delhi-110016, India.
* Corresponding author: [email protected]
1.    Importance of Mathematical Modelling in Food Engineering
The food engineering domain is considered to be a complex process due to the presence of various scalable throughputs, rheological properties of food molecules, different processes and environmental parameters, quality and stability concerns. Moreover, the influencing parameters impact the other parameters through the mutual dependencies. The complexity also lies in the raw and processed food commodities in the form of variability, which impacts the composition and their processability in further operations. Various food engineering problems, such as heat and mass transfer operations, are heterogeneous and variable. Therefore, it is not possible to handle them with only basic disciplines, such as mathematics, physics and science (BarnabĂŠ et al., 2018).
The heterogeneity and variability encountered in the food engineering domain can be made manageable by utilizing the modelling approaches which put forth the non-linear relationship of the different process parameters on the performance of the process or on the final yield of the food product (Shenoy et al., 2015). Mathematical modelling plays a vital role in understanding the food process related to thermal (heat and mass transfer) and non-thermal processes (high-pressure processing, pulsed electric field) and in assessing the possible microbial and biochemical changes during the shelf-life of the food products (Farid, 2010). The modelling approaches also tackle the scale-up associated problem and are useful in simulations, since they take care of impossible process parameters, such as high temperatures (Dutta, 2016).
The machine-learning/simulation-based optimization approaches help in attaining/predicting the optimum output by considering the individual, square and interaction parameters of the process. The machine-learning based optimization approaches produce a huge set of data for processing with the intuitive knowledge of the nature-based phenomenon. Moreover, the modelling and optimization approach also helps in attaining a deductive conclusion with a smaller set of experiments with the positive expert-knowledge based methodologies (Perrot et al., 2016). Computational Fluid Mechanics (CFM) also play an essential role in the food engineering domain, with various applications in process operations, such as drying, freezing, cooking, sterilization and baking, by providing food engineers with new insights into the probable performance of the equipment during the time-course of quality or safety (Dutta, 2016). Artificial Neural Networks (ANN) are also one of the machine learning tools with the potential for use in the food industry to tackle the food characteristics and understand the structure-process interaction during the production step of food products (Huang et al., 2007).
2.    Experiment Design and Data Analysis
The most important step in food engineering is the experiment design. In all the related work, such as enzyme production and other food related products, there is a need to design the experiment before beginning in the laboratory or at pilot scale. All of the dependent and independent variables need to be defined before the experiment, and based on the optimization methods, an accurate mathematical method should be chosen. Figure 1 explains the various basic terminologies used in experiment design.
Figure 2 shows the three basic principles used for the design of experiment (DOE). The three basic principles for DOE are âreplicationâ, ârandomizationâ and âcontrol of experimental errorâ.
Using randomization eliminates the systematic bias and aids in the distribution of all the designed experiments. In order to make a completely randomized design (CRD), the entire experiment must be divided into a number of experimental units, say x. In this design, the total number of replications for different treatments are fixed in advance. The replication procedure helps in repeating the experiment at the centre stage and it also estimates the experimental error. The experiments are repeated twice or thrice and mean values are also estimated. The control of experimental error will in turn increase the efficiency. If the sample size is large, the CRD experiments are not homogenous and there are n treatments to be compared, then it may be possible to: # Blocks are build and this procedure is called as randomized block design (RBD). The experimental material is divided into rows and columns in the Latin square method (LSD). In the LSD, each experiment occurred only once in the each row and column.
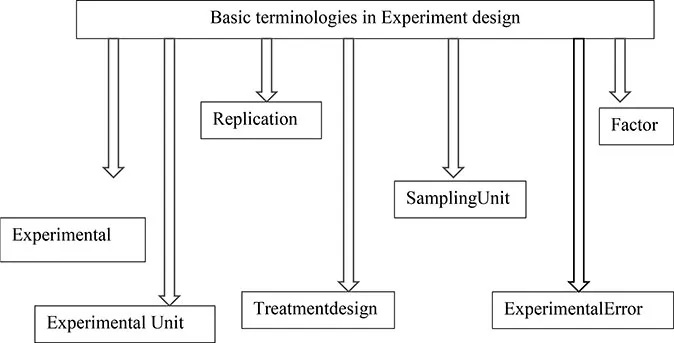
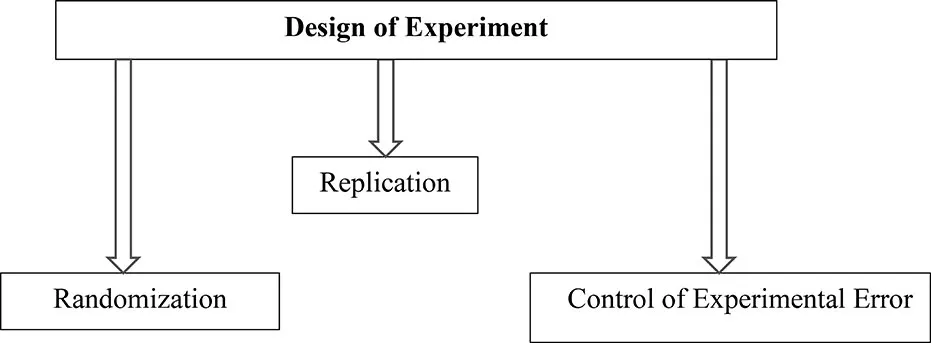
3.    Typical Problems in Application of Mathematical Modelling in Food Engineering
The typical problems encountered while using the modelling and optimization approaches in a food engineering discipline are mainly due to the usage of low-level manual-coded languages, such as FORTRAN or C, for implementation. This is highly resource-consuming and error-prone. Furthermore, it is impossible to utilize the approaches to plant-wide simulation by taking care of multiple interacting units. The FORTRAN or C-based modelling approaches also lack robustness and efficiency in dealing with the multitask problems associated with the food engineering domain (Banga et al., 2003).
Despite the well-proven modelling and optimization approaches in the food industry for the typical processes, the utilization of the adopted approaches for other food engineering operations is limited due to the following factors:
â˘Â  The developed food process modelâs applicability is not straightforward (Bimbenet et al., 2007).
â˘Â  Uncertainties encountered due to the raw material properties, process parameters and unreliability associated with the process conditions (Ioannou et al., 2006).
â˘Â  The developed process models are applicable to tiny sectors of the food engineering domain (Perrot et al., 2006).
â˘Â  The proposed process models are not able to integrate with the other developed models (Bimbenet et al., 2007).
Moreover, due to the dynamic nature of food processes, the developed food process models are very complicated in terms of the structural understanding, nature of simulation and optimized control. The complexity will be greater while developing a process model and taking care of relationships between composition, structure, properties, quality and shelf-life of the food, resulting in a very challenging task (Fito et al., 2007).
4.    Conclusion
Mathematical/statistical modelling facilitates a real understanding of the non-linear problems associated with food engineering operations with the aid of statistical heuristics and helps in the development of validated non-linear regression models. This approach helps in attaining the process optimization with well-planned and less experimental DOE. The developed mathematical models help in straightforward extrapolation towards the scale-up studies. In conclusion, there is a need for the industry and academia to work together in order to further the progression in modelling approaches by handling the typical problems encountered in the execution of modelling approaches in food engineering.
References
Banga, J.R., Balsa-Canto, E., Moles, C.G. and Alonso, A.A. 2003. Improving food processing using modern optimization methods. Trends in Food Science & Technology 14: 131â144.
BarnabĂŠ, M., Blanc, N., Chabin, T., Delenne, J.Y., Duri, A., Frank, X. et al. 2018. Multiscale modelling for bioresources and bioproducts. Innovative Food Science & Emerging Technologies 46: 41â53.
Bimbenet, J.J., Schubert, H. and Trystram, G. 2007. Advances in research in food process engineering as presented at ICEF9. Journal of Food Engineering 78: 390â404.
Datta, A.K. 2016. Toward computer-aided food engineering: Mechanistic frameworks for evolution of product, quality and safety during processing. Journal of Food Engineering 176: 9â27.
Farid, M.M. 2010. Mathematical Modelling of Food Processing, published by CRC Press, Taylor & Francis Group, 2010, ISBN 978-1-4200-5351-7.
Fito, P., LeMaguer, M., Betoret, N. and Fito, P.J. 2007. Advanced food process engineering to model real foods and processes: The SAFES methodology. Journal of Food Engineering 83: 390â404.
Huang, Y., Kangas, L.J. and Rasco, B.A. 2007. Applications of artificial neural networks (ANNs) in food science. Crit Rev Food SciNutr 47(2): 113â26.
Ioannou, I., Mauris, G., Trystram, G. and Perrot, N. 2006. Back-propagation of imprecision in a cheese ripening fuzzy model based on human sensory evaluations. Fuzzy Sets and Systems 157: 1179â1187.
Perrot, N., Ioannou, I., Allais, I., Curt, C., Hossenlopp, J. and Trystram, G. 2006. Fuzzy concepts applied to food product quality control: A review. Fuzzy Sets and Systems 157: 1145â1154.
Perrot, N., De Vries, H., Lutton, E., Van Mil, H.G., Donner, M. et al. 2016. Some remarks on computational approaches towards sustainable complex agrifood systems. Trends in Food Science & Technology 48: 88â101.
Shenoy, P., Viau, M., Tammel, K., Innings, F., Fitzpatrick, J. and AhrnĂŠ, L. 2015. Effect of powder densities, particle size and shape on mixture quality of binary food powder mixtures. Powder Technology 272: 165â172.
CHAPTER 2
Evolutionary Optimization Techniques as Effective Tools for Process Modelling in Food Processing
Lakshmishri Roy,1,* Debabrata Bera2 and Vijay Kumar Garlapati3
1 Dept. of Food Technology, Techno India, Kolkata, West Bengal-700091, India.
2 Dept. of Food and Biochemical Engineering, Jadavpur University, Kolkata, West ...
Table of contents
- Cover
- Title Page
- Copyright Page
- Foreword
- Preface
- Table of Contents
- 1. Role of Mathematical and Statistical Modelling in Food Engineering
- 2. Evolutionary Optimization Techniques as Effective Tools for Process Modelling in Food Processing
- 3. Optimization of Food Processes Using Mixture Experiments: Some Applications
- 4. Microorganisms and Food Products in Food Processing Using Full Factorial Design
- 5. The Use of Correlation, Association and Regression Techniques for Analyzing Processes and Food Products
- 6. Application of Cluster Analysis in Food Science and Technology
- 7. Multiway Statistical Methods for Food Engineering and Technology
- 8. Application of Multivariate Statistical Analysis for Quality Control of Food Products
- 9. Importance of Normality Testing, Parametric and Non-Parametric Approach, Association, Correlation and Linear Regression (Multiple & Multivariate) of Data in Food & Bio-Process Engineering
- 10. Regression Analysis Methods for Agri-Food Quality and Safety Evaluations Using Near-Infrared (NIR) Hyperspectral Imaging
- 11. Partial Least Square Regression for Food Analysis: Basis and Example
- 12. Mathematical Modelling of High Pressure Processing in Food Engineering
- 13. Food Process Modeling and Optimization by Response Surface Methodology (RSM)
- 14. A Mathematical Approach to the Modelling of the Rheological Properties of Solid Foods
- 15. Mathematical Models for Analyzing the Microbial Growth in Food
- 16. Computational Fluid Dynamics (CFD) Simulations in Food Processing
- 17. Application of Multivariate Statistical Analysis for Food Safety and Quality Assurance
- 18. Mathematical Modelling in Food Science through the Paradigm of Eggplant Drying
- 19. Use of Mathematical Modelling of Dough Biscuits Baking Behaviour
- 20. Applications of Principal Component Analysis (PCA) for Fruit Juice Recovery and Quality Analysis
- 21. Use of Artificial Neural Networks in Optimizing Food Processes
- 22. Application of Neural Networks in Optimizing Different Food Processes: Case Study
- 23. Mathematical Modelling for Predicting the Temperatures During Microwave Heating of Solid Foods: A Case Study
- 24. Microwave Drying of Food Materials Modelled by the Reaction Engineering Approach (REA)âFramework
- 25. Modelling of Heat Transfer During Deep Fat Frying of Food
- Index
- Color Section