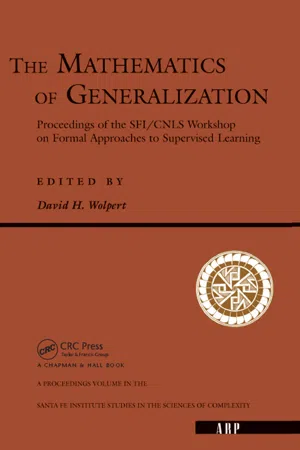
This is a test
- 460 pages
- English
- ePUB (mobile friendly)
- Available on iOS & Android
eBook - ePub
The Mathematics Of Generalization
Book details
Book preview
Table of contents
Citations
About This Book
This book provides different mathematical frameworks for addressing supervised learning. It is based on a workshop held under the auspices of the Center for Nonlinear Studies at Los Alamos and the Santa Fe Institute in the summer of 1992.
Frequently asked questions
At the moment all of our mobile-responsive ePub books are available to download via the app. Most of our PDFs are also available to download and we're working on making the final remaining ones downloadable now. Learn more here.
Both plans give you full access to the library and all of Perlego’s features. The only differences are the price and subscription period: With the annual plan you’ll save around 30% compared to 12 months on the monthly plan.
We are an online textbook subscription service, where you can get access to an entire online library for less than the price of a single book per month. With over 1 million books across 1000+ topics, we’ve got you covered! Learn more here.
Look out for the read-aloud symbol on your next book to see if you can listen to it. The read-aloud tool reads text aloud for you, highlighting the text as it is being read. You can pause it, speed it up and slow it down. Learn more here.
Yes, you can access The Mathematics Of Generalization by David. H Wolpert, David. H Wolpert in PDF and/or ePUB format, as well as other popular books in Mathematics & Mathematics General. We have over one million books available in our catalogue for you to explore.
Information
Baskin Center for Computer Engineering and Information Sciences, University of California, Santa Cruz, CA 95064; e-mail: [email protected].
Decision Theoretic Generalizations of the PAC Model for Neural Net and Other Learning Applications
This chapter, reprinted by permission, originally appeared in Information and Computation 100(1) (1992): 78–150. Copyright © by Academic Press.
We describe a generalization of the PAC learning model that is based on statistical decision theory. In this model the learner receives randomly drawn examples, each example consisting of an instance x ∈ X and an outcome y ∈ Y, and tries to find a decision rule h: X → A, where h ∈ , that specifies the appropriate action a ∈ A to take for each instance x, in order to minimize the expectation of a loss l(y,a). Here X, Y, and A are arbitrary sets, l is a real-valued function, and examples are generated according to an arbitrary joint distribution on X × Y. Special cases include the problem of learning a function from X into Y, the problem of learning the conditional probability distribution on Y given X (regression), and the problem of learning a distribution on X (density estimation).
We give theorems on the uniform convergence of empirical loss estimates to true expected loss rates for certain decision rule spaces , and show how this implies learnability with bounded sample size, disregarding computational complexity. As an application, we give distribution-independent upper bounds on the sample size needed for learning with feedforward neural networks. Our theorems use a generalized notion of VC dimension that applies to classes of real-valued functions, adapted from Vapnik and Pollard’s work, and a notion of capacity and metric dimension for classes of functions that map into a bounded metric space.
1. INTRODUCTION
The introduction of the Probably Approximately Correct (PAC) model4,86 of learning from examples has done an admirable job of ...
Table of contents
- Cover
- Half Title
- Title Page
- Copyright Page
- Table of Contents
- Preface
- The Status of Supervised Learning Science circa 1994—The Search for a Consensus
- Reflections After Refereeing Papers for NIPS
- The Probably Approximately Correct (PAC) and Other Learning Models
- Decision Theoretic Generalizations of the PAC Model for Neural Net and Other Learning Applications
- The Relationship Between PAC, the Statistical Physics Framework, the Bayesian Framework, and the VC Framework
- Statistical Physics Models of Supervised Learning
- On Exhaustive Learning
- A Study of Maximal-Coverage Learning Algorithms
- On Bayesian Model Selection
- Soft Classification, a.k.a. Risk Estimation, via Penalized Log Likelihood and Smoothing Spline Analysis of Variance
- Current Research
- Preface to Simplifying Neural Networks by Soft Weight Sharing
- Simplifying Neural Networks by Soft Weight Sharing
- Error-Correcting Output Codes: A General Method for Improving Multiclass Inductive Learning Programs
- Image Segmentation and Recognition
- Index