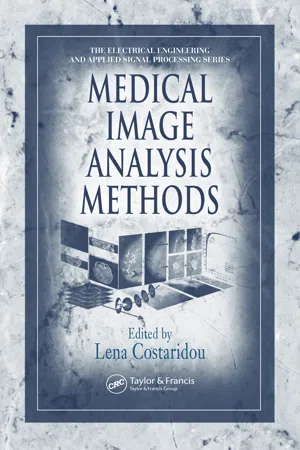
This is a test
- 504 pages
- English
- ePUB (mobile friendly)
- Available on iOS & Android
eBook - ePub
Medical Image Analysis Methods
Book details
Book preview
Table of contents
Citations
About This Book
To successfully detect and diagnose disease, it is vital for medical diagnosticians to properly apply the latest medical imaging technologies. It is a worrisome reality that due to either the nature or volume of some of the images provided, early or obscured signs of disease can go undetected or be misdiagnosed. To combat these inaccuracies, diagno
Frequently asked questions
At the moment all of our mobile-responsive ePub books are available to download via the app. Most of our PDFs are also available to download and we're working on making the final remaining ones downloadable now. Learn more here.
Both plans give you full access to the library and all of Perlegoās features. The only differences are the price and subscription period: With the annual plan youāll save around 30% compared to 12 months on the monthly plan.
We are an online textbook subscription service, where you can get access to an entire online library for less than the price of a single book per month. With over 1 million books across 1000+ topics, weāve got you covered! Learn more here.
Look out for the read-aloud symbol on your next book to see if you can listen to it. The read-aloud tool reads text aloud for you, highlighting the text as it is being read. You can pause it, speed it up and slow it down. Learn more here.
Yes, you can access Medical Image Analysis Methods by Lena Costaridou in PDF and/or ePUB format, as well as other popular books in Medicine & Biotechnology in Medicine. We have over one million books available in our catalogue for you to explore.
Information
1
Computer-Aided Diagnosis of Breast Cancer
Heang-Ping Chan, Berkman Sahiner, Nicholas Petrick, Lubomir Hadjiiski, and Sophie Paquerault
1.1 INTRODUCTION
Mammography is currently the only proven and cost-effective method to detect early breast cancer. A mammographic examination generally contains four images, two views for each breast. One is a craniocaudal (CC) view, and the other is a mediolateral oblique (MLO) view. These two views are designed to include most of the breast tissues within the X-ray images. Mammographic interpretation can be con- sidered a two-step process. A radiologist first screens the mammograms for abnor- malities. If a suspicious abnormality is detected, further diagnostic workup is then performed to estimate the likelihood that the abnormality is malignant. Diagnostic workup might include mammograms of additional views such as lateromedial (LM) or exaggerated craniocaudal (XCC) views, magnification views, spot views, as well as ultrasound scanning of the suspicious area.
The main mammographic signs of breast cancer are clustered microcalcifications and masses. Microcalcifications are calcium deposits in the breast tissue manifested as clusters of white specks of sizes from about 0.05 mm to 0.5 mm in diameter. Masses have X-ray absorption similar to that of fibroglandular tissue and are man- ifested as focal low-optical- density regions on mammograms. Some benign breast diseases also cause the formation of clustered microcalcifications and masses in the breast. The mammographic features of the malignant microcalcifications or masses are nonspecific and have a large overlap with those from benign diseases.
Because of the nonspecific features of malignant lesions, mammographic interpretation is a very challenging task for radiologists. Studies indicate that the sensi- tivity of breast cancer detection on mammograms is only about 70 to 90% [1ā6]. In a study that retrospectively reviewed prior mammograms taken of breast cancer patients before the exam in which the cancer was detected, it was found that 67% (286/427) of the cancers were visible on the prior mammograms and about 26% (112/427) were considered actionable by radiologists [7].
Missed cancers can be caused by detection errors or characterization errors. Detection errors can be attributed to factors such as oversight or camouflaging of the lesions by overlapping tissues. Even if a lesion is detected, the radiologist may underestimate the likelihood of malignancy of the lesion so that no action is taken. This corresponds to a characterization error. On the other hand, the radiologist may overestimate the likelihood of malignancy and recommend benign lesions for biopsy. It has been reported that of the lesions that radiologists recommended for biopsy, only about 15 to 30% are actually malignant [8]. The large number of benign biopsies not only causes patient anxiety, but also increases health-care costs. In addition, the scar tissue resulting from biopsy often makes it more difficult to interpret the patientās mammograms in the future. The sensitivity and specificity of mammography for detecting a lesion and differentiating the lesion as malignant or benign will need to be improved. It can be expected that early diagnosis and treatment will further improve the chance of survival for breast cancer patients [9ā12].
Various methods are being developed to improve the sensitivity and specificity of breast cancer detection [13]. Double reading can reduce the miss rate of radiographic reading [14, 15]. However, double reading by radiologists is costly. Com- puter-aided detection (CAD) is considered to be one of the promising approaches that may improve the efficacy of mammography [16, 17]. Computer-aided lesion detection can be used during screening to reduce oversight of suspicious lesions that warrant further diagnostic workup. Computer-aided lesion characterization can also be used during workup to provide additional information for making biopsy recom- mendation. It has been shown that CAD can improve radiologistsā detection accuracy significantly [18ā23]. Receiver operating characteristic (ROC) studies [24, 25] showed that computer-aided characterization of lesions can improve radiologistsā ability in differentiating malignant and benign masses or microcalcifications. CAD is thus a viable cost-effective alternative to double reading by radiologists.
The promise of CAD has stimulated research efforts in this area. Many com- puter vision techniques have been developed in various areas of CAD for mam- mography. Examples of work include: detection of microcalcifications [18, 26ā38], characterization of microcalcifications [39ā49], detection of masses [19, 40, 50ā73], and characterization of masses [24, 74ā78]. Computerized classification of mammographic lesions using radiologist-extracted features has also been reported by a number of investigators [79ā 84]. There are similarities and differ- ences among the computer vision techniques used by researchers. However, it is difficult to compare the performance of different detection programs because the performance strongly depends on the data set used for testing. These studies generally indicate that an effective CAD system can be developed using properly designed computer vision techniques.
Efforts to evaluate the usefulness of CAD in reducing missed cancers are ongo- ing. Results of a prospective study by Nishikawa et al. [85] indicated that their CAD algorithms can detect 54% (9/16) of breast cancer in the prior year with four false positives (FPs) per image when the mammograms were called negative but the cancer was visible in retrospect. In our recent study of detection on independent prior films [86], we found that 74% (20/27) of the malignant masses and 57% (4/7) of the malignant microcalcifications were detected with 2.2 mass marks/image and 0.8 cluster marks/image by our computer programs. A commercial system also reported a sensitivity of 77% (88/115) in one study [7] and 61% (14/23) in another study [87]for detection of the cancers in the prior years that were considered actionable in retrospect by expert mammographers. A prospective study of 12,860 patients in a community breast cancer center with a commercial CAD system that had about one mark per image reported a cancer detection rate of 81.6% (40/49), with eight of the cancers initially detected by computer only. This corresponded to a 20% increase in the number of cancers detected (41 vs. 49) when radiologists used CAD. Similar gain in cancer detection has been observed in a premarket retrospective study of another commercial system [23].
These results demonstrate that, even if a CAD system does not detect all cancers present and has some FPs, it can still reduce the missed cancer rate when used as a second opinion by radiologists. This is consistent with the first laboratory ROC study in CAD reported by us in 1990 [18], which demonstrated that a CAD program with a sensitivity of 87% and an FP rate of 0.5 to 4 per image could significantly improve radiologistsā accuracy in detection of subtle microcalcifications. In a recent prospective pilot clinical trial [88] of a CAD system developed by our group, a total of 11 cancers were detected in a screening patient cohort of about 2600 patients. The radiologists detected 10 of the 11 cancers without our CAD system. The CAD system also detected 10 of the 11 cancers. However, one of the computer-detected cancers was different from those detected by the radiologists, and this additional cancer was diagnosed when the radiologist was alerted to the site by the CAD system. In a 1-year follow-up of the cases, it was found that five more cancers were diagnosed in the patient cohort. Our computer system marked two of the five cancers, although all five cancers were deemed not actionable in the year of the pilot study when the mammograms were reviewed retrospectively by an experi- enced radiologist.
For classification of malignant and benign masses, our ROC study 24 indicated that a classifier with an area under the ROC curve, Az, of 0.92 could significantly improve radiologistsā classification accuracy with a predicted increase in the positive predictive value of biopsy. Jiang et al. [25] also found in an ROC study that their classifier with an Az of 0.80 could significantly improve radiologistsā characterization of malignant and benign microcalcifications, with a predicted reduction in biopsies. Recently, Hadjiiski et al. [89, 90] performed an ROC study to evaluate the effects of a classifier based on interval-change analysis on radiologistsā classification accu- racy of masses in serial mammograms. They found that when the radiologists took into account the rating of the computer classifier, they reduced the biopsy recom- mendation of the benign masses in the data set while slightly increasing the biopsy recommendation of the malignant masses. This result indicated that CAD improved radiologistsā accuracy in classifying malignant and benign masses based on serial mammograms and has the potential of reducing unnecessary biopsy.
In the last few years, full-field digital mammography (FFDM) technology has advanced rapidly because of the potential of digital imaging to improve breast cancer detection. Four manufacturers have obtained clearance from the Food and Drug Administration (FDA) for clinical use. It is expected that digital mammography detectors will provide higher signal-to-noise ratio (SNR) and detective quantum efficiency (DQE), wider dynamic range, and higher contrast sensitivity than digitized film mammograms. Because of the higher SNR and linear response of digital detec- tors, there is a strong potential that more effective feature-extraction techniques can be designed to optimally extract signal content from the direct digital images and improve the accuracy of CAD. The potential of improving CAD accuracy by exploit- ing the imaging properties of digital mammography is a subject of ongoing research.
In mammographic screening, it has been reported that taking two views of each breast, a CC and an MLO view, provides a higher sensitivity and specificity than one view for breast cancer detection [2,91ā93]. Radiologists use the two views to confirm true positives (TPs) and to reduce FPs. Current CAD algorithms detect lesions only on a single mammographic view. New CAD algorithms that utilize the correlation of computer-detected lesions between the two views are being developed [69, 94ā99]. Our studies demonstrated that the correlated lesion information from two views could be used to reduce FPs and improve detection [100, 101]. Although the development is still at the early stage and continued effort is needed to further improve the two-view correlation techniques, this promising development will be summarized here in the hope that it will stimulate research interests.
Another important technique that radiologists use in mammographic interpreta- tion is to compare the current and prior mammograms and to evaluate the interval changes. Interval-change analysis can be used to detect newly developed abnormality or to evaluate growth of existing lesions. Hadjiiski et al. [97, 98] developed a regional-registration technique to automatically identify the location of a correspond- ing lesion on the same view of a prior mammogram. Feature extraction and classi- fication techniques could then be developed to differentiate malignant and benign lesions using interval-change information. Interval-change features were found to be useful in improving the classification accuracy. In this chapter, we will concentrate on lesion detection, rather than characterization. Computer vision methods for clas- sification of malignant and benign lesions, ...
Table of contents
- COVER PAGE
- TITLE PAGE
- COPYRIGHT PAGE
- PREFACE
- THE EDITOR
- CONTRIBUTORS
- 1: COMPUTER-AIDED DIAGNOSIS OF BREAST CANCER
- 2: MEDICAL-IMAGE PROCESSING AND ANALYSIS FOR CAD SYSTEMS
- 3: TEXTURE AND MORPHOLOGICAL ANALYSIS OF ULTRASOUND IMAGES OF THE CAROTID PLAQUE FOR THE ASSESSMENT OF STROKE
- 4: BIOMEDICAL-LMAGE CLASSIFICATION METHODS AND TECHNIQUES
- 5: TEXTURE CHARACTERIZATION USING AUTOREGRESSIVE MODELS WITH APPLICATION TO MEDICAL IMAGING
- 6: LOCALLY ADAPTIVE WAVELET CONTRAST ENHANCEMENT
- 7: THREE-DIMENSIONAL MULTISCALE WATERSHED SEGMENTATION OF MR IMAGES
- 8: A MRF-BASED APPROACH FOR THE MEASUREMENT OF SKIN THICKNESS IN MAMMOGRAPHY
- 9: LANDMARK-BASED REGISTRATION OF MEDICAL-IMAGE DATA
- 10: GRAPH-BASED ANALYSIS OF AMINO ACID SEQUENCES
- 11: ESTIMATION OF HUMAN CORTICAL CONNECTIVITY WITH MULTIMODAL INTEGRATION OF FMRI AND HIGH-RESOLUTION EEG
- 12: EVALUATION STRATEGIES FOR MEDICAL-IMAGE ANALYSIS AND PROCESSING METHODOLOGIES