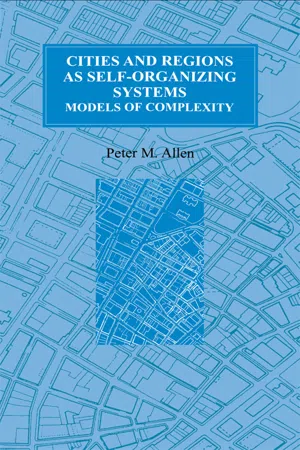
This is a test
- 267 pages
- English
- ePUB (mobile friendly)
- Available on iOS & Android
eBook - ePub
Book details
Book preview
Table of contents
Citations
About This Book
A clear methodological and philosophical introduction to complexity theory as applied to urban and regional systems is given, together with a detailed series of modelling case studies compiled over the last couple of decades. Based on the new complex systems thinking, mathematical models are developed which attempt to simulate the evolution of towns, cities, and regions and the complicated co-evolutionary interaction there is both between and within them. The aim of these models is to help policy analysis and decision-making in urban and regional planning, energy policy, transport policy, and many other areas of service provision, infrastructure planning, and investment that are necessary for a successful society.
Frequently asked questions
At the moment all of our mobile-responsive ePub books are available to download via the app. Most of our PDFs are also available to download and we're working on making the final remaining ones downloadable now. Learn more here.
Both plans give you full access to the library and all of Perlegoâs features. The only differences are the price and subscription period: With the annual plan youâll save around 30% compared to 12 months on the monthly plan.
We are an online textbook subscription service, where you can get access to an entire online library for less than the price of a single book per month. With over 1 million books across 1000+ topics, weâve got you covered! Learn more here.
Look out for the read-aloud symbol on your next book to see if you can listen to it. The read-aloud tool reads text aloud for you, highlighting the text as it is being read. You can pause it, speed it up and slow it down. Learn more here.
Yes, you can access Cities and Regions as Self-Organizing Systems by Peter M. Allen in PDF and/or ePUB format, as well as other popular books in Architecture & Urban Planning & Landscaping. We have over one million books available in our catalogue for you to explore.
Information
1.
TOWARDS A SCIENCE OF COMPLEX SYSTEMS
1.1 INTRODUCTION
Since the great work of Newton, the mechanical vision of the world has been the basis of our understanding. Even though sub-atomic and atomic physics were revolutionized by quantum physics, the mechanical vision remained paramount in our comprehension of events at the level of our everyday experience. This means that we explain things on the basis of âcausal mechanismsâ, where components influence each other and form systems, in which the change seen in one part is explained by change in another, or in the external environment in which the system is embedded. These mechanisms could be written down as mathematical equations, as mathematical models expressing fundamental laws of nature, and then used to predict behaviour. The paths traced by the system from any given initial state were pre-determined by the equations and therefore it was believed that surprise could only come from the outside.
However, although this might have seemed reasonable for mechanical systems before the recognition of deterministic chaos, in human systems it does seem at odds with everyday experience. For many people, the key choices of career or partner hinge upon events which are intrinsically extremely improbable, and as economists and politicians would agree, changes and trends in society are very difficult to anticipate correctly. Why are human systems different?
To see why, let us imagine a very simple human situation, for example, of traffic moving along a highway or of pedestrians milling around a shopping centre. Clearly, movements cannot be predicted using Newton's laws of motion because acceleration, change of direction, braking and stopping occur at the whim of each driver or pedestrian. Newton's laws, the laws of physics, are obeyed at all times by each part of the system, but, despite this, they are not of help in predicting what will happen because the decision to coast, turn, accelerate or brake lies with the human being. Planets, billiard balls, and point particles are helpless slaves to the force fields in which they move, but people are not! People can switch sources of energy on or off and can respond, react, learn and change according to their individual experience and personality. This of course poses some fundamental questions: how can âchoiceâ and âfreedomâ arise in living systems (assuming they do)? If they do not arise in âmechanicalâ systems, does it mean that living systems are not mechanical? But, if they are not mechanical, then what are they?
In this volume we explore how the new ideas emerging originally from the physics of open systems (ones which exchange energy and matter with their surroundings) begin to answer these questions, and of more practical interest, how they can be used to understand the emergence and evolution of spatial pattern and structure in human systems. They offer a new understanding of the origins, and evolution of patterns of human settlement and land-use, as well as urban growth and structure.
Our example of the highway traffic or pedestrian precinct shows us that there is a critical difference between asking whether a system obeys the laws of physics or whether its behaviour can be predicted from a knowledge of those laws. Only when the energy and momentum of the elements of a system obey a precise unvarying law is prediction possible. In the case of the human systems such as those above, clearly, the autonomy of the actors, and their ability to add and subtract energy to the system according to their own wishes is what makes prediction impossible unless we can find some fixed rules of behaviour, or some fixed âgoalâ from which behaviour can be derived. This latter basis would suppose that the people concerned could correctly infer what behaviour they should have in order to attain their goals, and this of course is open to considerable doubt. Prediction would rely on such clarity of vision however, as well as there being no interaction between behaviours, and indeed between goals.
Prediction concerning a car that has run out of fuel is much easierâit will slow down and eventually stop! But living systems with access to reserves of energy are not like this. People have choices and can make decisions. Even if an individual knows exactly what he would like to achieve, then if this involves other people, because he cannot know with certainty how everyone else will respond, he can never calculate exactly what he must do. He must make his decision, and see what happens, being ready to take corrective actions, if necessary. Since, in society, on the road and in the shopping centre we are all making these kinds of decisions, simultaneously, all the time, it is not surprising that occasionally there are accidents, or that such systems run in a ânon-mechanicalâ way. An important point to remember here is of course that human beings have evolved within such a system and therefore that the capacity to live with such permanent uncertainty is quite natural to us. It may even be what characterises the living. However, it also implies that much of what we do may be inexplicable in rational terms.
In trying to bring the power of science to the understanding of human systems, understandably, these complexities were initially ignored. It was assumed that if someone knew what he wanted, then he could get enough information to make sure that his choice led to that goal. This course led directly to rational man and in the limit homo economicus where observed behaviour was supposed to be derived from the individual's definition of his goalsâhis âutilityâ, usually expressed in monetary terms. By assuming a fixed form for this âutilityâ, the behaviour of the individual could be âpredictedâ in changing circumstances, and the system could thus be made âdeterministicâ. In this way, mathematical models could be built describing similar individuals faced with a series of choices and predictions concerning, for example, residential or economic location choices, or shopping centre hinterlands. But these predictions used inferred âutilitiesâ which were based on observed structures, assuming tacitly therefore that everyone was fully satisfied, and the system was at equilibrium. This does not seem a promising basis for studying and understanding change, since this could not come from any pent up desire within the system, but only as a result of some external influence.
This vision of equilibrium, and this machine-like image of individuals and organizations with clear and unique responses to events that are perceived with absolute clarity is, of course, not one that normal people would readily recognise as corresponding to reality. People in real life seem much less clear about their choices, as well as seeming changeable according to their mood, and even when they are clear, the outcomes that ensue are often not what they expected. This âover rationalâ approach has been softened but not fundamentally changed by statistical models of decision processes where the probability of making a particular choice is proportional to the expected utility derived. This gives rise to probabilistic behaviour for individuals and deterministic behaviour for sufficiently large populations. However, this simple approach ignores the fact that decisions made by individuals are not really independent of each other, and that there is an effect of the communication between individuals. Fashions, styles and risk minimising strategies affect collective behaviour considerably, and mean that it cannot be derived necessarily as the sum of independent, individual responses. Another problem is that we can only calibrate (put a value to) âutility functionsâ by ensuring that they âreproduceâ observed behaviour, and without some independent determination of the âgoalsâ people have, then the modelling process is in danger of circularity approaching tautology.
What is required if we are to ever understand human systems is some new approach which accepts that people interact and that their âutilitiesâ are linked in a complex, co-evolutionary fashion. In addition we need to understand how we can ever write mathematical models which can capture such phenomena and in which structural changes can occur and new behaviours can emerge, going beyond the mechanical vision of classical science.
1.2 DETERMINISM, SELF-ORGANIZATION AND EVOLUTION
In order to try to set up a conceptual framework within which these complexities can be considered let us step back and consider the process of âmodellingâ itself. By considering this from first principles we can begin to see the assumptions involved in the mechanical representation of the world, and how we may hope to improve upon this with the new ideas of self-organizing and evolving systems.
If we examine a region, and consider the remains of populations and artifacts that litter the landscape, then after dating and classifying them, an evolutionary tree of some kind emerges, possibly with discontinuities suggesting disaster and invasion, but nevertheless suggesting a changing set of activities and behaviours, that have occupied the site over time. Development concerns the introduction and growth of new activities, and the successful mutual adaptation of the landscape and the population to these changes, leading to their maintenance and continued development. In order to provide decision support for the planning and implementation of development policies we must have models that can capture the creative dialogue between new investments and infrastructure and the chain of responses of the populations and of the environment these. Development is about structural change on many scales, from the patterns of thought and habits of the inhabitants, to the patterns of intra and inter-regional and world trade and commerce.
Models can be thought of in terms of a hierarchy, where increased âsimplicityâ of understanding and predictive capability is obtained by making increasingly strong assumptions, which may be increasingly unbelievable. On the left of Figure 1.1 we have ârealityâ. It makes no assumptions, and is drawn as a cloud, since we can say little about it other than that it includes all detail of everything, everywhere, as well as all perceptions and all points of view. However, from experience and intuition concerning the âproblemsâ that we want to address, we may construct an initial set of taxonomic rules concerning the differences and similarities of the objects, together with their dates, which leads to the construction of an âevolutionary treeâ, showing that species, behaviours, forms, or artifacts emerged and evolved over time.
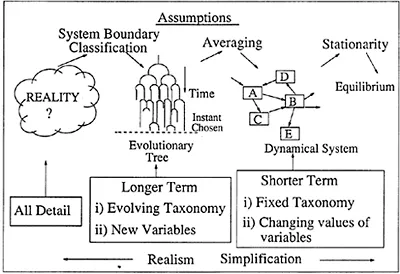
Figure 1.1 Understanding results from bounding the domain of interest, classifying the contents and their interactions, and then making assumptions which can lead to deterministic, self-organizing or evolutionary models.
The rules of classification that we use result from previous experience about such systems and what matters in them. Are there socio-economic âtypesâ? Do firms of the same sector and size behave similarly? What is a sector? Is there as much variation within a group as between groups? Whatever the precise arguments advanced, in order to âunderstandâ a situation, and its possible outcomes, we do classify the system into components, and attempt to build mathematical models that capture the processes that are increasing or decreasing these different components.
In order to build mathematical models, we identify the different objects or organisms that are present at a particular moment and attempt to write down the mechanisms describing the increase and decrease of each type. We apply the traditional approach of physics, which is to identify the components of a system, and the interactions operating on these, both to and from the outside world and between the different populations of the system. By considering the demographic, economic and environmental processes in play at a given time, a mathematical model can be produced which appears to offer deterministic predictions concerning the future, assuming different possible policies or exogenous events.
However, as we see clearly from our broader picture of the âevolutionary treeâ, the predictions that such a model gives can only be correct for as long as the qualitative structure of the system remains unchanged. Development concerns particularly the emergence of new spatial organization, new activities and behaviours, and the structural changes that these lead to. The mechanical model of deterministic equations, the System Dynamics, that we can construct at any given time has no way of producing ânewâ types of objects, new variables, and so the âpredictionsâ that it generates will only be true until some moment, unpredictable within the model, when there is an adaptation or innovation, and new behaviour emerges.
In recent research new models have been developed (Allen, 1992, 1993, 1994) which can generate a true structurally changing evolution, with new entities and activities appearing. However, the relationship between these âevolutionaryâ models and the more conventional ones has not been made clear, and a clarification of this will be one of the aims of this first section. The conceptual framework of Figure 1.1 allows us to understand the relationship between different modelling techniques used to provide decision support, in terms of the assumptions that underlie them. We compare the assumptions made by different approaches to policy exploration and planning, such as static optimization models, evaluations based on short term cost/benefits, and the difficulties involved in long term, complex simulations.
Clearly, the evolutionary tree reflects the changing structure of the system, with different variables, over the long term, as different types of actor emerge, flourish and then disappear or change. In the short term, however, we can identify the different objects or actors that are present, and write down some âsystem dynamics equationsâ describing the mutual interaction of the different actors present. In other words, in describing the short term we can apply the traditional approach of physics (Prigogine and Stengers, 1987; Allen, 1988), which is to identify the components of a system, and the interactions operating on these, both to and from the outside world and between the different populations of the system. In ecology, this...
Table of contents
- COVER PAGE
- TITLE PAGE
- COPYRIGHT PAGE
- FOREWORD
- PREFACE
- ACKNOWLEDGEMENTS
- 1. TOWARDS A SCIENCE OF COMPLEX SYSTEMS
- INTER-URBAN EVOLUTION
- CASE STUDIES AND FURTHER DEVELOPMENTS
- INTRA-URBAN EVOLUTION
- POLICY EXPLORATION AND DECISION SUPPORT FOR SUSTAINABLE DEVELOPMENT
- APPENDIX
- REFERENCES