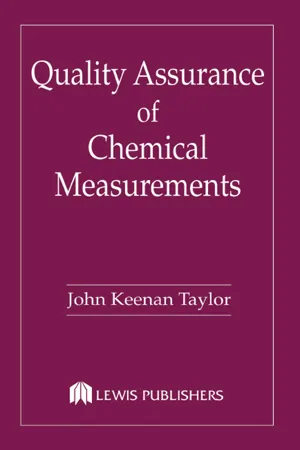
This is a test
- 352 pages
- English
- ePUB (mobile friendly)
- Available on iOS & Android
eBook - ePub
Quality Assurance of Chemical Measurements
Book details
Book preview
Table of contents
Citations
About This Book
This definitive new book should appeal to everyone who produces, uses, or evaluates scientific data. Ensures accuracy and reliability. Dr. Taylor's book provides guidance for the development and implementation of a credible quality assurance program, plus it also provides chemists and clinical chemists, medical and chemical researchers, and all scientists and managers the ideal means to ensure accurate and reliable work. Chapters are presented in a logical progression, starting with the concept of quality assurance, principles of good measurement, principles of quality assurance, and evaluation of measurement quality. Each chapter has a degree of independence so that it may be consulted separately from the others.
Frequently asked questions
At the moment all of our mobile-responsive ePub books are available to download via the app. Most of our PDFs are also available to download and we're working on making the final remaining ones downloadable now. Learn more here.
Both plans give you full access to the library and all of Perlegoās features. The only differences are the price and subscription period: With the annual plan youāll save around 30% compared to 12 months on the monthly plan.
We are an online textbook subscription service, where you can get access to an entire online library for less than the price of a single book per month. With over 1 million books across 1000+ topics, weāve got you covered! Learn more here.
Look out for the read-aloud symbol on your next book to see if you can listen to it. The read-aloud tool reads text aloud for you, highlighting the text as it is being read. You can pause it, speed it up and slow it down. Learn more here.
Yes, you can access Quality Assurance of Chemical Measurements by John K. Taylor in PDF and/or ePUB format, as well as other popular books in Physical Sciences & Chemistry. We have over one million books available in our catalogue for you to explore.
CHAPTER 1
Introduction to Quality Assurance
The demand for measurement data is ever increasing. Decisions need to be made on such questions as the suitability of a material for an intended purpose, the quality of the environment, and the health of individuals. The vast majority of such measurements entail chemical analyses in which some chemical property of the material, object, or system of interest is measured.
Dramatic changes have taken place in chemical analysis during the past several decades. Most measurements are now being made using complex instruments in sophisticated processes. Moreover, measurements made by several analysts and/or laboratories often need to be interrelated for use in a decision process. Monitoring programs of regional, national, or international scope may be involved. All of these developments involve stringent requirements for the reliability and compatibility of the data.
The quality of data is ordinarily evaluated on the basis of its uncertainty when compared with end-use requirements. If data have consistency and the uncertainty is small when compared to the requirements, they are considered to be of adequate quality. When excessively variable or the level of uncertainty exceeds the needs, the data may be said to be of low or inadequate quality. The evaluation of data quality is thus a relative determination. What is high quality in one situation could be unacceptable in another.
The quality of chemical data may be judged on the basis of two aspects: the accuracy of identification of the parameter measured and the numerical accuracy. The qualitative identification must be beyond reasonable doubt. The analyst must state precisely what was measured and be able to prove it. While empirical measurements defined by a specific apparatus or methodology may be useful in some situations, they must be specified as such and used with due consideration of their limitations.
Quantitative measurements are always estimates of the value of the measure and involve some level of uncertainty. The measurements must be made so that the limits of uncertainty can be assigned within a stated probability. Without such an assignment, no logical use can be made of the data. To achieve this, measurements must be made in such a way to provide statistical predictability. The experience of metrologists has demonstrated that this is achieved best by a well-designed and consistently implemented quality assurance program.
Quality assurance consists of two separate but related activities: quality control and quality assessment. Both must be operational and coordinated. The following definitions are offered [132].*
Quality assurance: A system of activities whose purpose is to provide to the producer or user of a product or a service the assurance that it meets defined standards of quality with a stated level of confidence.
Quality control: The overall system of activities whose purpose is to control the quality of a product or service so that it meets the needs of users. The aim is to provide quality that is satisfactory, adequate, dependable, and economic.
Quality assessment: The overall system of activities whose purpose is to provide assurance that the overall quality control job is being done effectively. It involves a continuing evaluation of the products produced and of the performance of the production system.
The objective of quality control is to fine tune and maintain a measurement process in a desired state of stability and reproducibility. In such, the process can be considered to have a high degree of similarity to an industrial production process, with the ability to produce objects (in this case data) with a high degree of reproducibility. Once this is established, the precision may be defined and any biases can usually be identified and eliminated or compensated, so that requisite data quality is achieved. Quality assessment provides the evidence that this has been accomplished.
The general approach to quality assurance of the measurement process parallels that for the industrial production process and borrows concepts and techniques proven trustworthy for the latter, to the extent possible. In industry, the procedure may be called statistical product control. In metrology, the procedure may be called statistical measurement control.
The quality assurance procedure followed in a typical production process is illustrated in Figure 1.1. Experience dictates the kind and degree of control that must be achieved and maintained to obtain products of desired quality. Random samples of the output are tested or inspected with respect to specifications in order to release or accept the production lot, to reject it, or to take corrective actions in the process.
The quality assurance aspects of a typical measurement process are illustrated in Figure 1.2. Quality control procedures are used to tune and maintain the system in a state of statistical control in which it may be considered as capable of generating an infinite number of measurements on any material, of which the data of the moment are a typical sample. Quality assessment procedures are then used to evaluate the quality of the data that is produced. Unfortunately, it is generally impossible, intrinsically, to evaluate fully the quality of the data on unknown test materials. However, if the measurement system is maintained in a state of statistical control, known samples such as reference materials (RM), which simulate the test samples, may be measured concurrently and the results compared with the reference values. Such a comparison can be used to evaluate the performance of the measurement system and permit inferences to be made on the quality of the data for test samples.
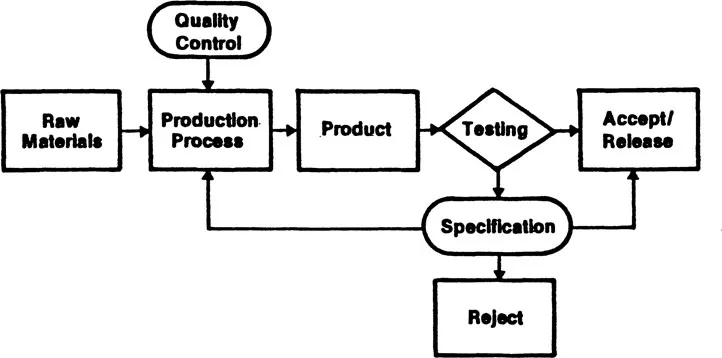
Figure 1.1Ā Ā Production process quality assurance.
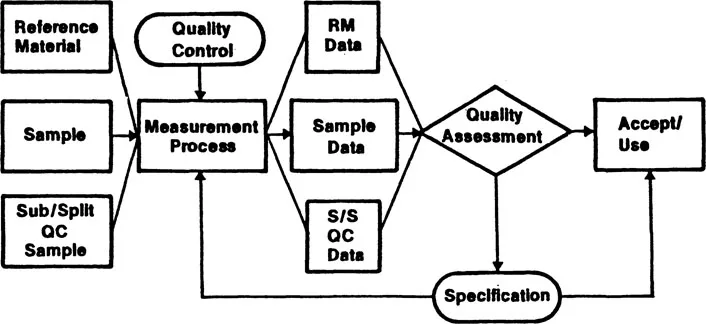
Figure 1.2Ā Ā Measurement process quality assurance.
It should now be evident that statistics is an integral part of quality assurance. In fact, what has really been discussed here is statistical assurance of quality. It may be looked at in the following way:
Statistical |
Use of data from a stable measurement system |
Assurance |
to provide probabilistic confidence of achievement |
Quality |
of a desired level of acceptability |
By common usage, the term has been shortened to quality assurance.
This discussion concerns the quality of measurement data, and indeed the quality of individual measured values. When considered collectively as a body of numerical information, additional quality descriptors may be useful. Thus the Environmental Protection Agency proposes [125]:
CompletenessāA measure of the amount of data obtained from a measurement process compared to the amount that was expected to be obtained under the conditions of measurement.
RepresentativenessāThe degree to which the data accurately and precisely represent a characteristic of a population parameter, variation of a property, a process characteristic, or an operational condition.
ComparabilityāThe confidence with which one data set can be compared to another.
Although they are difficult to quantify, such characteristics need to be considered when evaluating the usefulness of a set of data for scientific interpretation.
In addition to meeting the requirements stated above, data must be both technically sound and legally defensible. That is to say, it must be defensible against any reasonable adversarial inquiry or action, legal or otherwise. There is a difference in these requirements and both need to be met. The first is necessary but not sufficient. It includes all those things that a careful competent analytical chemist should do. The second includes the proof that sound work was done. Good quality assurance practices are central to achieving both goals. They stress documentation which takes the burden off memory and can remove any suspicion or shadow of doubt of details of what was done. Good quality assurance practices cannot give credence to poor technical work. In fact, they can help to identify poor quality when it exists. But, lack of documentation can make defense deficient if not impossible. While legal defense in its broadest connotation is often the issue, technical reliability is always at stake. Quality assurance practices can promote this as well. In fact, measurements should always be made, believing that there is someone somewhere who may have some questions about what was done. When questions are anticipated, measurement plans often can be adjusted to answer them and to document what is done with little or no extra effort.
Before ending this introductory discussion, we should remember that there are two aspects of quality. First, there is design quality which is based on a plan to meet an envisioned need. This is essential if anything useful is to result from any production process, whether it provides products, services, or data. The second is conformance quality and is concerned with the achievement of design quality. The first does not guarantee the second, but the second is hardly achievable without the first.
Production of quality data does not occur automatically and is not guaranteed by following any one of several established procedures. It is enhanced by:
ā¢Ā Ā understanding the measurement process
ā¢Ā Ā understanding what needs to be measured
ā¢Ā Ā understanding what needs to be done to obtain reliable measurements
Quality can be achieved by dedicated effort, by careful attention to detail, and by practicing recognized principles of quality assurance. Such efforts must be accompanied by a desire for excellence and the creation of an atmosphere conducive to ensuring quality.
Quality assurance is more than a program; it is a philosophy, a way of life. As a program that is mechanically followed, quality assurance is doomed to failure. As a philosophy, there is a chance for success. When it is approached as both a program and a philosophy, the chances for producing high quality data are excellent.
*Ā Ā The numbers in brackets here and throughout the book are citations to publications in the Bibliography contained in Appendix E.
CHAPTER 2
Precision, Bias, and Accuracy
Accuracy is concerned with correctness. If a measurement process produces the correct results, it is accurate, and the measured value is also accurate. Strictly speaking, only a counting process is exact and hence accurate. A measured value is an estimate and may be āclose enoughā to the correct value to be considered āerrorless,ā and the process so producing it may be considered to be accurate.
When closely scrutinized, repetitive measurements will differ from one another, and the means of sets will differ, however to a lesser degree. The scatter of the values is a measure of the precision; the less the scatter, the higher the precision. In a stable measurement process, a large number of individual values will tend to converge toward a limiting mean, which may or may not be the true value. If not, the process is said to be biased.
The above discussion leads to the following definitions:
Accuracy | āThe degree of agreement of a measured value with the true or expected value of the quantity of concern. |
Bias | āA systematic error inherent in a method or caused by some artifact or idiosyncrasy of the measurement system. Temperature effects and extraction inefficiencies are examples of the first kind. Blanks, contamination, mechanical losses, and calibration errors are examples of the latter kinds. Bias may be both positive and negative, and several kinds can exist concurrently so that net bias is all that can be evaluated, except under special conditions. |
Precision | āThe degree of mutual agreement characteristic of independent measurements as the result of... |
Table of contents
- Cover
- Title Page
- Copyright Page
- Table of Contents
- Preface
- CHAPTER 1. Introduction to Quality Assurance
- CHAPTER 2. Precision, Bias, and Accuracy
- CHAPTER 3. Statistical Control
- CHAPTER 4. Statistical Techniques
- CHAPTER 5. Chemical Analysis as a System
- CHAPTER 6. The Model
- CHAPTER 7. Planning
- CHAPTER 8. Principles of Sampling
- CHAPTER 9. Principles of Measurement
- CHAPTER 10. Principles of Calibration
- CHAPTER 11. Principles of Quality Assurance
- CHAPTER 12. Principles of Quality Control
- CHAPTER 13. Blank Correction
- CHAPTER 14. Control Charts
- CHAPTER 15. Principles of Quality Assessment
- CHAPTER 16. Evaluation Samples
- CHAPTER 17. Reference Materials
- CHAPTER 18. Traceability
- CHAPTER 19. Quality Audits
- CHAPTER 20. Quality Circles
- CHAPTER 21. Validation
- CHAPTER 22. Reporting Analytical Data
- CHAPTER 23. An Improved Approach to Performance Testing
- CHAPTER 24. Correction of Errors and/or Improving Accuracy
- CHAPTER 25. Laboratory Evaluation
- CHAPTER 26. The Quality Assurance Program
- Appendix A. Terminology Used in Quality Assurance
- Appendix B. Quality Assurance Program Documentation
- Appendix C. Statistical Tables
- Appendix D. Study Aids
- Appendix E. Annotated Bibliography of Selected Publications on Quality Assurance of Measurements
- Index