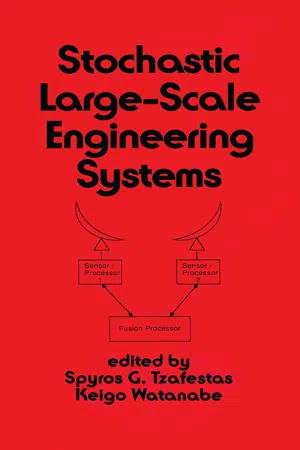
This is a test
- 400 pages
- English
- ePUB (mobile friendly)
- Available on iOS & Android
eBook - ePub
Stochastic Large-Scale Engineering Systems
Book details
Book preview
Table of contents
Citations
About This Book
This book focuses on the class of large-scale stochastic systems, which has dominated the attention of many academic and research groups. It discusses distributed-sensor networks, decentralized detection theory, and econometric models with integrated and decentralized policymakers.
Frequently asked questions
At the moment all of our mobile-responsive ePub books are available to download via the app. Most of our PDFs are also available to download and we're working on making the final remaining ones downloadable now. Learn more here.
Both plans give you full access to the library and all of Perlego’s features. The only differences are the price and subscription period: With the annual plan you’ll save around 30% compared to 12 months on the monthly plan.
We are an online textbook subscription service, where you can get access to an entire online library for less than the price of a single book per month. With over 1 million books across 1000+ topics, we’ve got you covered! Learn more here.
Look out for the read-aloud symbol on your next book to see if you can listen to it. The read-aloud tool reads text aloud for you, highlighting the text as it is being read. You can pause it, speed it up and slow it down. Learn more here.
Yes, you can access Stochastic Large-Scale Engineering Systems by Tzafestas in PDF and/or ePUB format, as well as other popular books in Mathematics & Probability & Statistics. We have over one million books available in our catalogue for you to explore.
Information
1
Decentralized Bayesian Detection Theory
PRAMOD K. VARSHNEY Syracuse University, Syracuse, New York
1. Introduction
Classical Bayesian hypothesis testing is based on centralized processing (Van Trees, 1968). A single agent or multiple agents collect observations about the state of the environment and transmit them to a central location for processing. Given the a priori probabilities and conditional densities of the observations under each hypothesis, fixed costs are assigned to each possible course of action. Optimum decision rules are then derived which minimize the average cost. The resulting decision rule is a likelihood ratio test.
There has been a lot of recent interest in the area of decentralized decision making. Under this paradigm, multiple agents collect observations about the state of the environment. These observations are processed by the agent, and the partial result to the global hypothesis-testing problem is transmitted to the data fusion center, which is responsible for combining the partial results to yield a global inference. Partial results could be in the form of sufficient statistics, some other form of compressed data, or even tentative decisions. The fusion center must solve a hypothesis-testing problem to yield the global inference, with incoming data being treated as its observations. Thus we are faced with...
Table of contents
- Cover
- Half Title
- Series Page
- Title Page
- Copyright Page
- Preface
- Contents
- Contributors
- Introduction
- Chapter 1. Decentralized Bayesian Detection Theory
- Chapter 2. Distributed Estimation in Distributed-Sensor Networks
- Chapter 3. Estimation of Large Sparse Systems
- Chapter 4. External Input Identification in Distributed Parameter Systems Using the Boundary Element Method
- Chapter 5. Interaction and Structure Concepts for Large-Scale Systems
- Chapter 6. Practical Issues of Coordination in Control and Optimization of Large-Scale Stochastic Systems
- Chapter 7. Filtering, Smoothing, and Control in Discrete-Time Stochastic Distributed-Sensor Networks
- Chapter 8. A Weak Contrast Function Approach to Adaptive Semi-Markov Decision Models
- Chapter 9. Large-Scale Stochastic Control Systems: Stability and Stabilization
- Chapter 10. Estimation of Attractors and Invariant Domains for Perturbed Complex and Large-Scale Systems
- Chapter 11. Controls of Flexible Mechanical Structures
- Chapter 12. Adaptive Control of Econometric Models with Integrated and Decentralized Policymakers
- Index