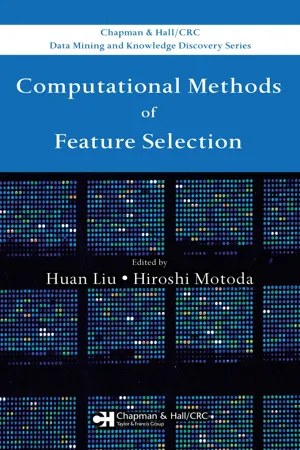
eBook - PDF
Computational Methods of Feature Selection
This is a test
- 440 pages
- English
- PDF
- Available on iOS & Android
eBook - PDF
Computational Methods of Feature Selection
Book details
Table of contents
Citations
About This Book
Due to increasing demands for dimensionality reduction, research on feature selection has deeply and widely expanded into many fields, including computational statistics, pattern recognition, machine learning, data mining, and knowledge discovery. Highlighting current research issues, Computational Methods of Feature Selection introduces the
Frequently asked questions
At the moment all of our mobile-responsive ePub books are available to download via the app. Most of our PDFs are also available to download and we're working on making the final remaining ones downloadable now. Learn more here.
Both plans give you full access to the library and all of Perlegoâs features. The only differences are the price and subscription period: With the annual plan youâll save around 30% compared to 12 months on the monthly plan.
We are an online textbook subscription service, where you can get access to an entire online library for less than the price of a single book per month. With over 1 million books across 1000+ topics, weâve got you covered! Learn more here.
Look out for the read-aloud symbol on your next book to see if you can listen to it. The read-aloud tool reads text aloud for you, highlighting the text as it is being read. You can pause it, speed it up and slow it down. Learn more here.
Yes, you can access Computational Methods of Feature Selection by Huan Liu, Hiroshi Motoda, Huan Liu, Hiroshi Motoda in PDF and/or ePUB format, as well as other popular books in Economics & Statistics for Business & Economics. We have over one million books available in our catalogue for you to explore.
Information
Table of contents
- Cover
- Title
- Copyright
- Preface
- Acknowledgments
- Contributors
- Contents
- Part I: Introduction and Background
- Chapter 1: Less Is More
- Chapter 2: Unsupervised Feature Selection
- Chapter 3: Randomized Feature Selection
- Chapter 4: Causal Feature Selection
- Part II: Extending Feature Selection
- Chapter 5: Active Learning of Feature Relevance
- Chapter 6: A Study of Feature Extraction Techniques Based on Decision Border Estimate
- Chapter 7: Ensemble-Based Variable Selection Using Independent Probes
- Chapter 8: Efficient Incremental-Ranked Feature Selection in Massive Data
- Part III: Weighting and Local Methods
- Chapter 9: Non-Myopic Feature Quality Evaluation with (R)ReliefF
- Chapter 10: Weighting Method for Feature Selection in K-Means
- Chapter 11: Local Feature Selection for Classification
- Chapter 12: Feature Weighting through Local Learning
- Part IV: Text Classification and Clustering
- Chapter 13: Feature Selection for Text Classification
- Chapter 14: A Bayesian Feature Selection Score Based on NaĂŻve Bayes Models
- Chapter 15: Pairwise Constraints-Guided Dimensionality Reduction
- Chapter 16: Aggressive Feature Selection by Feature Ranking
- Part V: Feature Selection in Bioinformatics
- Chapter 17: Feature Selection for Genomic Data Analysis
- Chapter 18: A Feature Generation Algorithm with Applications to Biological Sequence Classification
- Chapter 19: An Ensemble Method for Identifying Robust Features for Biomarker Discovery
- Chapter 20: Model Building and Feature Selection with Genomic Data
- Index