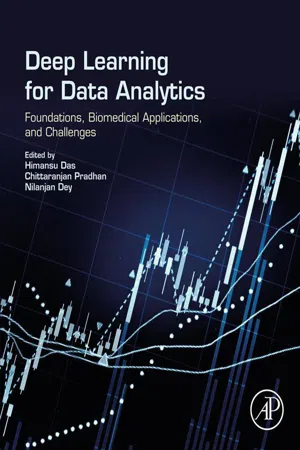
Deep Learning for Data Analytics
Foundations, Biomedical Applications, and Challenges
- 218 pages
- English
- ePUB (mobile friendly)
- Available on iOS & Android
Deep Learning for Data Analytics
Foundations, Biomedical Applications, and Challenges
About This Book
Deep learning, a branch of Artificial Intelligence and machine learning, has led to new approaches to solving problems in a variety of domains including data science, data analytics and biomedical engineering. Deep Learning for Data Analytics: Foundations, Biomedical Applications and Challenges provides readers with a focused approach for the design and implementation of deep learning concepts using data analytics techniques in large scale environments. Deep learning algorithms are based on artificial neural network models to cascade multiple layers of nonlinear processing, which aids in feature extraction and learning in supervised and unsupervised ways, including classification and pattern analysis. Deep learning transforms data through a cascade of layers, helping systems analyze and process complex data sets. Deep learning algorithms extract high level complex data and process these complex sets to relatively simpler ideas formulated in the preceding level of the hierarchy. The authors of this book focus on suitable data analytics methods to solve complex real world problems such as medical image recognition, biomedical engineering, and object tracking using deep learning methodologies. The book provides a pragmatic direction for researchers who wish to analyze large volumes of data for business, engineering, and biomedical applications. Deep learning architectures including deep neural networks, recurrent neural networks, and deep belief networks can be used to help resolve problems in applications such as natural language processing, speech recognition, computer vision, bioinoformatics, audio recognition, drug design, and medical image analysis.
- Presents the latest advances in Deep Learning for data analytics and biomedical engineering applications.
- Discusses Deep Learning techniques as they are being applied in the real world of biomedical engineering and data science, including Deep Learning networks, deep feature learning, deep learning toolboxes, performance evaluation, Deep Learning optimization, deep auto-encoders, and deep neural networks
- Provides readers with an introduction to Deep Learning, along with coverage of deep belief networks, convolutional neural networks, Restricted Boltzmann Machines, data analytics basics, enterprise data science, predictive analysis, optimization for Deep Learning, and feature selection using Deep Learning
Frequently asked questions
Information
Short and noisy electrocardiogram classification based on deep learning
Abstract
Keywords
1.1 Introduction
Table of contents
- Cover image
- Title page
- Table of Contents
- Copyright
- List of contributors
- Preface
- Chapter one. Short and noisy electrocardiogram classification based on deep learning
- Chapter two. Single-layer convolution neural network for cardiac disease classification using electrocardiogram signals
- Chapter three. Generalization performance of deep autoencoder kernels for identification of abnormalities on electrocardiograms
- Chapter four. Deep learning for early diagnosis of Alzheimer’s disease: a contribution and a brief review
- Chapter five. Musculoskeletal radiographs classification using deep learning
- Chapter six. Deep-wavelet neural networks for breast cancer early diagnosis using mammary termographies
- Chapter seven. Deep learning on information retrieval and its applications
- Chapter eight. Electrical impedance tomography image reconstruction based on autoencoders and extreme learning machines
- Chapter nine. Crop disease classification using deep learning approach: an overview and a case study
- Index