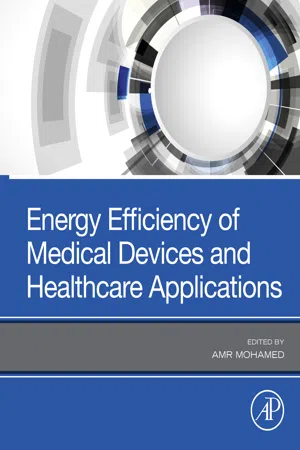
- 184 pages
- English
- ePUB (mobile friendly)
- Available on iOS & Android
Energy Efficiency of Medical Devices and Healthcare Applications
About This Book
Energy Efficiency of Medical Devices and Healthcare Facilities provides comprehensive coverage of cutting-edge, interdisciplinary research, and commercial solutions in this field. The authors discuss energy-related challenges, such as energy-efficient design, including renewable energy, of different medical devices from a hardware and mechanical perspectives, as well as energy management solutions and techniques in healthcare networks and facilities. They also discuss energy-related trade-offs to maximize the medical devices availability, especially battery-operated ones, while providing immediate response and low latency communication in emergency situations, sustainability and robustness for chronic disease treatment, in addition to high protection against cyber-attacks that may threaten patients' lives. Finally, the book examines technologies and future trends of next generation healthcare from an energy efficiency and management point of view, such as personalized or smart health and the Internet of Medical Things — IoMT, where patients can participate in their own treatment through innovative medical devices and software applications and tools. The books applied approach makes it a useful resource for engineering researchers and practitioners of all levels involved in medical devices development, healthcare systems, and energy management of healthcare facilities. Graduate students in mechanical and electric engineering, and computer science students and professionals also benefit.
- Provides in-depth knowledge and understanding of the benefits of energy efficiency in the design of medical devices and healthcare networks and facilities
- Presents best practices and state-of-art techniques and commercial solutions in energy management of healthcare networks and systems
- Explores key energy tradeoffs to provide scalable, robust, and effective healthcare systems and networks
Frequently asked questions
Information
AI-based techniques on edge devices to optimize energy efficiency in m-Health applications
Abstract
Keywords
1. Introduction

Table of contents
- Cover image
- Title page
- Table of Contents
- Copyright
- Reviewers
- Contributors
- Preface
- Chapter 1. AI-based techniques on edge devices to optimize energy efficiency in m-Health applications
- Chapter 2. Applying an efficient evolutionary algorithm for EEG signal feature selection and classification in decision-based systems
- Chapter 3. Edge computing for energy-efficient smart health systems: Data and application-specific approaches
- Chapter 4. Energy-efficient EEG monitoring systems for wireless epileptic seizure detection
- Chapter 5. Intelligent energy-aware decision-making at the edge in healthcare using fog infrastructure
- Chapter 6. Deep learning-based security schemes for implantable medical devices
- Chapter 7. Secure medical treatment with deep learning on embedded board
- Chapter 8. Blockchain applications for healthcare
- Index