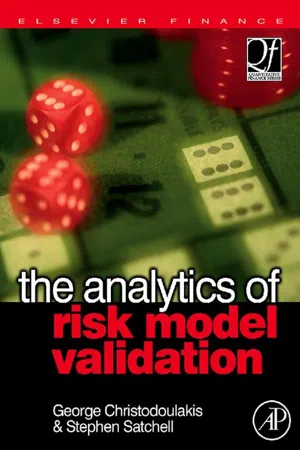
- 216 pages
- English
- ePUB (mobile friendly)
- Available on iOS & Android
The Analytics of Risk Model Validation
About This Book
Risk model validation is an emerging and important area of research, and has arisen because of Basel I and II. These regulatory initiatives require trading institutions and lending institutions to compute their reserve capital in a highly analytic way, based on the use of internal risk models. It is part of the regulatory structure that these risk models be validated both internally and externally, and there is a great shortage of information as to best practise. Editors Christodoulakis and Satchell collect papers that are beginning to appear by regulators, consultants, and academics, to provide the first collection that focuses on the quantitative side of model validation. The book covers the three main areas of risk: Credit Risk and Market and Operational Risk.*Risk model validation is a requirement of Basel I and II *The first collection of papers in this new and developing area of research *International authors cover model validation in credit, market, and operational risk
Frequently asked questions
Information
Determinants of small business default*
ā Federal Reserve Bank of Chicago, Chicago, IL
ā” Office of the Comptroller of the Currency, Washington, DC
¶ College of Business Administration, University of Nevada, Reno, NV
Abstract
1 Introduction
Table of contents
- Cover image
- Title page
- Table of Contents
- Copyright page
- About the editors
- About the contributors
- Preface
- 1: Determinants of small business default
- 2: Validation of stress testing models
- 3: The validity of credit risk model validation methods
- 4: A moments-based procedure for evaluating risk forecasting models
- 5: Measuring concentration risk in credit portfolios
- 6: A Simple method for regulators to cross-check operational risk loss models for banks
- 7: Of the credibility of mapping and benchmarking credit risk estimates for internal rating systems
- 8: Analytic models of the ROC Curve: Applications to credit rating model validation
- 9: The validation of equity portfolio risk models
- 10: Dynamic risk analysis and risk model evaluation
- 11: Validation of internal rating systems and PD estimates
- Index