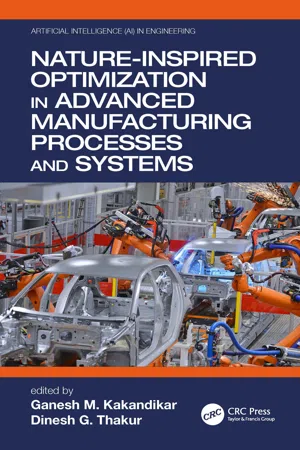
Nature-Inspired Optimization in Advanced Manufacturing Processes and Systems
- 262 pages
- English
- ePUB (mobile friendly)
- Available on iOS & Android
Nature-Inspired Optimization in Advanced Manufacturing Processes and Systems
About This Book
The manufacturing system is going through substantial changes and developments in light of Industry 4.0. Newer manufacturing technologies are being developed and applied. There is a need to optimize these techniques when applied in different circumstances with respect to materials, tools, product configurations, and process parameters.
This book covers computational intelligence applied to manufacturing. It discusses nature-inspired optimization of processes and their design and development in manufacturing systems. It explores all manufacturing processes, at both macro and micro levels, and offers manufacturing philosophies. Nonconventional manufacturing, real industry problems and case studies, research on generative processes, and relevance of all this to Industry 4.0 is also included.
Researchers, students, academicians, and industry professionals will find this reference title very useful.
Frequently asked questions
Information
1 Investigation on Process Parameters of EN-08 Steel by Using DoE and Multi-Objective Genetic Algorithm Approach
1.1 Introduction
Table of contents
- Cover
- Half Title
- Series Page
- Title Page
- Copyright Page
- Table of Contents
- Foreword
- Preface
- Editors
- Contributors
- Chapter 1 Investigation on Process Parameters of EN-08 Steel by Using DoE and Multi-Objective Genetic Algorithm Approach
- Chapter 2 Multi-Objective Optimization for Improving Performance Characteristics of Novel Curved EDM Process Using Jaya Algorithm
- Chapter 3 Artificial Neural Networks (ANNs) for Prediction and Optimization in Friction Stir Welding Process: An Overview and Future Trends
- Chapter 4 Energy-Efficient Cluster Head Selection for Manufacturing Processes Using Modified Honeybee Mating Optimization in Wireless Sensor Networks
- Chapter 5 Multiobjective Design Optimization of Power Take-Off (PTO) Gear Box Through NSGA II
- Chapter 6 Improving the Performance of Machining Processes Using Opposition-Based Learning Civilized Swarm Optimization
- Chapter 7 Application of Particle Swarm Optimization Method to Availability Optimization of Thermal Power Plants
- Chapter 8 Optimization of Incremental Sheet Forming Process Using Artificial Intelligence-Based Techniques
- Chapter 9 Development of Non-dominated Genetic Algorithm Interface for Parameter Optimization of Selected Electrochemical-Based Machining Processes
- Chapter 10 ANN Modeling of Surface Roughness and Thrust Force During Drilling of SiC Filler-Incorporated Glass/Epoxy Composites
- Chapter 11 Multi-objective Optimization of Laser-Assisted Micro-hole Drilling with Evolutionary Algorithms
- Chapter 12 Modeling and Pareto Optimization of Burnishing Process for Surface Roughness and Microhardness
- Chapter 13 Selection of Components and Their Optimum Manufacturing Tolerance for Selective Assembly Technique Using Intelligent Water Drops Algorithm to Minimize Manufacturing Cost
- Chapter 14 Enhancing the Surface Roughness Characteristics of Selective Inhibition Sintered HDPE Parts: An Integrated Approach of RSM and Krill Herd Algorithm
- Chapter 15 Optimization of Abrasive Water Jet Machining Parameters of Al/Tic Using Response Surface Methodology and Modified Artificial Bee Colony Algorithm
- Index