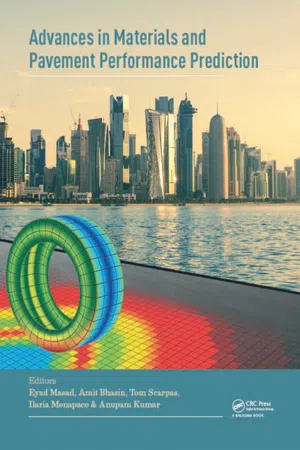
Advances in Materials and Pavement Prediction
Papers from the International Conference on Advances in Materials and Pavement Performance Prediction (AM3P 2018), April 16-18, 2018, Doha, Qatar
- 596 pages
- English
- ePUB (mobile friendly)
- Available on iOS & Android
Advances in Materials and Pavement Prediction
Papers from the International Conference on Advances in Materials and Pavement Performance Prediction (AM3P 2018), April 16-18, 2018, Doha, Qatar
About This Book
Advances in Materials and Pavement Performance Prediction contains the papers presented at the International Conference on Advances in Materials and Pavement Performance Prediction (AM3P, Doha, Qatar, 16- 18 April 2018).
There has been an increasing emphasis internationally in the design and construction of sustainable pavement systems. Advances in Materials and Pavement Prediction reflects this development highlighting various approaches to predict pavement performance. The contributions discuss links and interactions between material characterization methods, empirical predictions, mechanistic modeling, and statistically-sound calibration and validation methods. There is also emphasis on comparisons between modeling results and observed performance. The topics of the book include (but are not limited to):
• Experimental laboratory material characterization
• Field measurements and in situ material characterization
• Constitutive modeling and simulation
• Innovative pavement materials and interface systems
• Non-destructive measurement techniques
• Surface characterization, tire-surface interaction, pavement noise
• Pavement rehabilitation
• Case studies
Advances in Materials and Pavement Performance Prediction will be of interest to academics and engineers involved in pavement engineering.
Frequently asked questions
Information
Section 1: Modeling distress in flexible pavements
Modeling the relationships between pavement distress and performance
Yu Qiao & Sikai Chen
1 INTRODUCTION
2 METHODOLOGY
(1) |
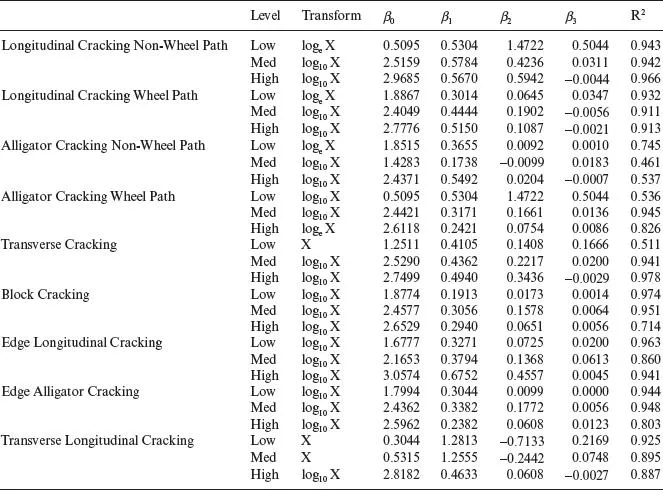
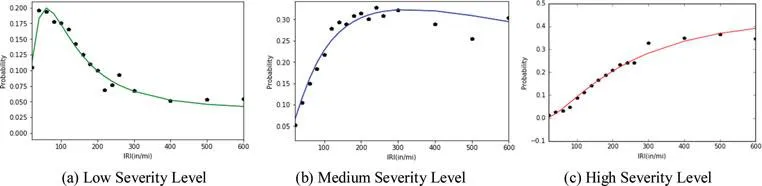
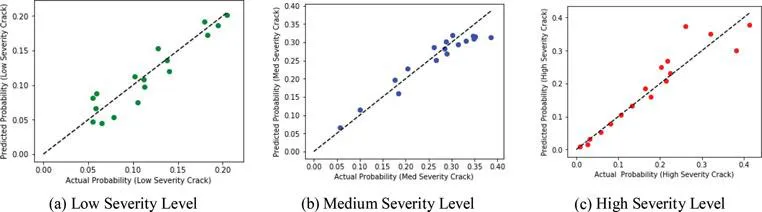
Table of contents
- Cover
- Half Title
- Title Page
- Copyright Page
- Table of Contents
- Preface
- Keynotes
- Session 1: Modeling distress in flexible pavements
- Session 2: Integrating material and structural response
- Session 3: Structural health assessment
- Session 4: Measuring and modeling mixture performance – 1
- Session 5: Measuring and modeling mixture performance – 2
- Session 6: Computational modeling to understand mixture production and behavior
- Session 7: Measuring and modeling performance of asphalt binders
- Session 8: Binders and emulsions: Workability, adhesion and rheology
- Session 9: Recycling in asphalt mixes
- Session 10: Modeling and measurement of noise and tire-pavement interaction
- Session 11: Pavement roughness: Measuring, modeling and implications – 1
- Session 12: Pavement roughness: Measuring, modeling and implications – 2
- Session 13: Developments in structural design of pavements
- Session 14: Variability in mixture and pavement design
- Session 15: Mixes and binders with additives and industrial waste
- Session 16: Chemomechanics and aging of binders – 1
- Session 17: Chemomechanics and aging of binders – 2
- Session 18: Rigid pavements 1
- Session 19: Rigid pavements 2
- Session 20: Pavement geotechnics 1
- Session 21: Pavement geotechnics 2
- Session 22: Innovations in pavement maintenance, analysis, and design
- Session 23: New asphalt mix paradigms
- Session 24: Modeling and measurement of chip seals
- Author index