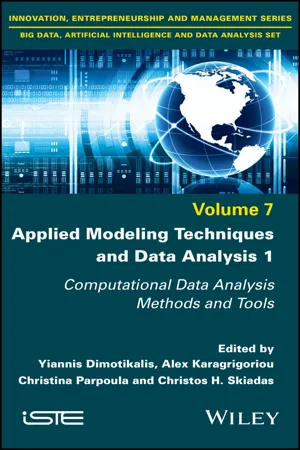
Applied Modeling Techniques and Data Analysis 1
Computational Data Analysis Methods and Tools
- English
- ePUB (mobile friendly)
- Available on iOS & Android
Applied Modeling Techniques and Data Analysis 1
Computational Data Analysis Methods and Tools
About This Book
BIG DATA, ARTIFICIAL INTELLIGENCE AND DATA ANALYSIS SET Coordinated by Jacques Janssen
Data analysis is a scientific field that continues to grow enormously, most notably over the last few decades, following rapid growth within the tech industry, as well as the wide applicability of computational techniques alongside new advances in analytic tools. Modeling enables data analysts to identify relationships, make predictions, and to understand, interpret and visualize the extracted information more strategically.
This book includes the most recent advances on this topic, meeting increasing demand from wide circles of the scientific community. Applied Modeling Techniques and Data Analysis 1 is a collective work by a number of leading scientists, analysts, engineers, mathematicians and statisticians, working on the front end of data analysis and modeling applications. The chapters cover a cross section of current concerns and research interests in the above scientific areas. The collected material is divided into appropriate sections to provide the reader with both theoretical and applied information on data analysis methods, models and techniques, along with appropriate applications.
Frequently asked questions
Information
PART 1
Computational Data Analysis
1
A Variant of Updating PageRank in Evolving Tree Graphs
1.1. Introduction
Table of contents
- Cover
- Table of Contents
- Title Page
- Copyright
- Preface
- PART 1: Computational Data Analysis
- PART 2: Data Analysis Methods and Tools
- List of Authors
- Index
- End User License Agreement