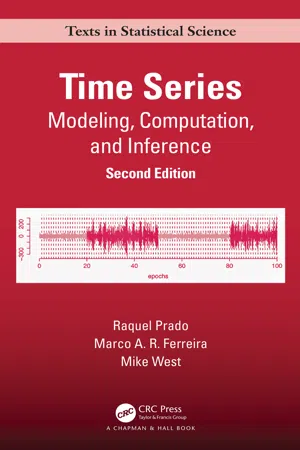
Time Series
Modeling, Computation, and Inference, Second Edition
- 472 pages
- English
- ePUB (mobile friendly)
- Available on iOS & Android
Time Series
Modeling, Computation, and Inference, Second Edition
About This Book
Focusing on Bayesian approaches and computations using analytic and simulation-based methods for inference, Time Series: Modeling, Computation, and Inference, Second Edition integrates mainstream approaches for time series modeling with significant recent developments in methodology and applications of time series analysis. It encompasses a graduate-level account of Bayesian time series modeling, analysis and forecasting, a broad range of references to state-of-the-art approaches to univariate and multivariate time series analysis, and contacts research frontiers in multivariate time series modeling and forecasting.
It presents overviews of several classes of models and related methodology for inference, statistical computation for model fitting and assessment, and forecasting. It explores the connections between time- and frequency-domain approaches and develop various models and analyses using Bayesian formulations and computation, including use of computations based on Markov chain Monte Carlo (MCMC) and sequential Monte Carlo (SMC) methods. It illustrates the models and methods with examples and case studies from a variety of fields, including signal processing, biomedicine, environmental science, and finance.
Along with core models and methods, the book represents state-of-the art approaches to analysis and forecasting in challenging time series problems. It also demonstrates the growth of time series analysis into new application areas in recent years, and contacts recent and relevant modeling developments and research challenges.
New in the second edition:
- Expanded on aspects of core model theory and methodology.
-
- Multiple new examples and exercises.
-
- Detailed development of dynamic factor models.
-
- Updated discussion and connections with recent and current research frontiers.
-
Frequently asked questions
Information
Chapter 1
Notation, definitions, and basic inference
1.1 Problem Areas and Objectives
Table of contents
- Cover
- Half Title
- Series Page
- Title Page
- Copyright Page
- Contents
- Preface
- Authors
- 1 Notation, definitions, and basic inference
- 2 Traditional time domain models
- 3 The frequency domain
- 4 Dynamic linear models
- 5 State-space TVAR models
- 6 SMC methods for state-space models
- 7 Mixture models in time series
- 8 Topics and examples in multiple time series
- 9 Vector AR and ARMA models
- 10 General classes of multivariate dynamic models
- 11 Latent factor models
- Bibliography
- Author Index
- Subject Index