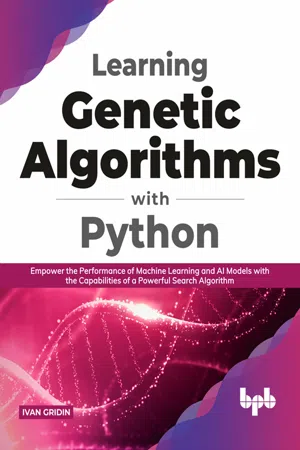
eBook - ePub
Learning Genetic Algorithms with Python
Empower the performance of Machine Learning and AI models with the capabilities of a powerful search algorithm (English Edition)
This is a test
- English
- ePUB (mobile friendly)
- Available on iOS & Android
eBook - ePub
Learning Genetic Algorithms with Python
Empower the performance of Machine Learning and AI models with the capabilities of a powerful search algorithm (English Edition)
Book details
Book preview
Table of contents
Citations
About This Book
Refuel your AI Models and ML applications with High-Quality Optimization and Search Solutions Key Features
- Complete coverage on practical implementation of genetic algorithms.
- Intuitive explanations and visualizations supply theoretical concepts.
- Added examples and use-cases on the performance of genetic algorithms.
- Use of Python libraries and a niche coverage on the performance optimization of genetic algorithms. Description
Genetic algorithms are one of the most straightforward and powerful techniques used in machine learning. This book 'Learning Genetic Algorithms with Python' guides the reader right from the basics of genetic algorithms to its real practical implementation in production environments.
Each of the chapters gives the reader an intuitive understanding of each concept. You will learn how to build a genetic algorithm from scratch and implement it in real-life problems. Covered with practical illustrated examples, you will learn to design and choose the best model architecture for the particular tasks. Cutting edge examples like radar and football manager problem statements, you will learn to solve high-dimensional big data challenges with ways of optimizing genetic algorithms. What you will learn
- Understand the mechanism of genetic algorithms using popular python libraries.
- Learn the principles and architecture of genetic algorithms.
- Apply and Solve planning, scheduling and analytics problems in Enterprise applications.
- Expert learning on prime concepts like Selection, Mutation and Crossover. Who this book is for
The book is for Data Science team, Analytics team, AI Engineers, ML Professionals who want to integrate genetic algorithms to refuel their ML and AI applications. No special expertise about machine learning is required although a basic knowledge of Python is expected. Table of Contents
1. Introduction
2. Genetic Algorithm Flow
3. Selection
4. Crossover
5. Mutation
6. Effectiveness
7. Parameter Tuning
8. Black-box Function
9. Combinatorial Optimization: Binary Gene Encoding
10. Combinatorial Optimization: Ordered Gene Encoding
11. Other Common Problems
12. Adaptive Genetic Algorithm
13. Improving Performance About the Author
Ivan Gridin is a mathematician, fullstack developer, data scientist, and machine learning expert living in Moscow, Russia. Over the years, he worked on distributive high-load systems and implemented different machine learning approaches in practice. One of the key areas of his research is design and analysis of predictive time series models.Ivan has fundamental math skills in probability theory, random process theory, time series analysis, machine learning, deep learning, and optimization. He also has an in-depth knowledge and understanding of various programming languages such as Java, Python, PHP, and MATLAB.He is a loving father, husband, and collector of old math books. LinkedIn Profile: www.linkedin.com/in/survex
Blog links: https://www.facebook.com/ivan.gridin/
Frequently asked questions
At the moment all of our mobile-responsive ePub books are available to download via the app. Most of our PDFs are also available to download and we're working on making the final remaining ones downloadable now. Learn more here.
Both plans give you full access to the library and all of Perlegoās features. The only differences are the price and subscription period: With the annual plan youāll save around 30% compared to 12 months on the monthly plan.
We are an online textbook subscription service, where you can get access to an entire online library for less than the price of a single book per month. With over 1 million books across 1000+ topics, weāve got you covered! Learn more here.
Look out for the read-aloud symbol on your next book to see if you can listen to it. The read-aloud tool reads text aloud for you, highlighting the text as it is being read. You can pause it, speed it up and slow it down. Learn more here.
Yes, you can access Learning Genetic Algorithms with Python by Ivan Gridin in PDF and/or ePUB format, as well as other popular books in Computer Science & Information Technology. We have over one million books available in our catalogue for you to explore.
Information
CHAPTER 1
Introduction
As we know, evolution is one of the most perfect adaptation mechanisms. It is a way to achieve extraordinary and complex solutions. Understanding the principles of evolution gave us a new approach called genetic algorithms. We will now explore this rather beautiful, simple, and effective approach to problem-solving.
Structure
In this chapter, we will discuss the following topics:
- Nature of genetic algorithm
- Applicability of genetic algorithms
- Pros and cons of genetic algorithms
- Your first genetic algorithm
1.1 Nature of genetic algorithm
The rapid development in AI is made possible for humans to obtain solution to abstract problems. Complex computational problems that are very difficult to solve by classical methods can now be solved by AI.
One of the most powerful techniques to solve such complex problems is genetic algorithms (GA), which is based on the principle of an evolutionary approach.
In the late 60s, American researcher J. Holland proposed to find solutions to optimization problems using methods and evolution models of animal populations in nature. Since the evolutionās basic laws were investigated and described by genetics, the proposed approach was called genetic algorithms. GA is a randomly directed search algorithm based on mechanisms of natural selection and natural genetics. It implements the principle of survival of the fittest, forming and changing the search algorithm based on evolutionary modeling.
The basic steps in natural evolution are as follows:
- Selection: According to Charles Darwin, natural selection laws were formulated in the book On the Origin of Species. The central postulate is that individuals who can better solve problems, survive and reproduce more. In GAs, each individual is a solution to some problem. According to this principle, individuals who solve the problem better have a greater chance of surviving and leaving offsprings.
- Crossover: This means that the offspring chromosome is made up of parts that are derived from the parentsā chromosomes. This principle was discovered in 1865 by G. Mendel.
- Mutation: In 1900, H. de Vries discovered the principle of random change. Initially, this term was used to describe significant changes in descendantsā properties that were not present in their parents. By analogy, genetic algorithms use a similar mechanism to change offspringās properties, thereby increasing individualsā diversity in a population.
Genetic algorithms have the following characteristics:
- Easy to implement
- Used for a wide range of tasks
- They do not require any additional information about the nature of the problem
- Easy and convenient to parallelize
1.2 Applicability of genetic algorithms
As a solution, the GA tries to find the extremum of some function that characterizes the quality of the solution to the problem. Generally, the GA does not guarantee that the solution found is the best of all thatās possible. Usually, this is not required, but it is only important that the found solution satisfies the meaning of the problem being solved.
The areas of application of GAs include the following:
- Search for extremum of various functions
- Finding the shortest paths (traveling salesman problem)
- Combinatorial optimization
- Tasks of placement and scheduling
- Automatic programming tasks
- AI tasks (choosing the structure and parameters of artificial neural networks)
In real time scenarios, GAs are used to develop AI systems, like designing tasks for aircraft routes at airports, finding the optimal behavior of robots, problems of constructing investment portfolios, and so on.
1.3 Pros and cons of genetic algorithms
Like any other approach to problem-solving, GAs have their pros and cons as well. Understanding these features will allow you to solvе the practical problems in a better way.
The pros of genetic algorithms are as follows:
- A wide range of tasks to be solved: GA is successfully applied in the following areas ā combinatorial optimization, finance (portfolio optimization), machine learning (feature extraction, neural network hyper-parameter optimization), code-breaking, game theory, natural sciences, and so on.
- Ease of implementation: The algorithm implies the presence of steps ā natural selection, crossing, and mutation. This conceptual simplicity makes this method available to a wide range of developers.
- Resistance to dynamic changes in problem conditions: The GA is able to retrain if the conditions of the problem change when searching for a solution.
- The ability for self-adaptation: GAs are able, after a certain period of evolution, to adapt to the conditions of the problem being solved.
- Ease of scaling: Can easily be used on big data where the data is spread over the distributed systems. GAs, as a highly parallel process, can be easily parallelized, which makes it possible to proportionally accelerate the finding of a solution with an increase in computing power.
- Solving problems for which there is no solution experience: One of the biggest advantages of GAs is their ability to investigate problems for which there is no relevant solution experience. It should be noted that expert assessments are often used to solve difficult-to-formalize problems, but they sometimes give less acceptable solutions than automated methods.
The cons of genetic algorithms are as follows:
- The complexity of representing an individual in a population and determining the fitness function.
- For real problems, it is initially not-at-all obvious in what form it is necessary to present a set of individual genes for a successful solution to the problem, and also determine the assessment of the quality of a particular individual.
- The c...
Table of contents
- Cover Page
- Title Page
- Copyright Page
- Dedication Page
- About the Author
- About the Reviewer
- Acknowledgement
- Preface
- Errata
- Table of Contents
- 1. Introduction
- 2. Genetic Algorithm Flow
- 3. Selection
- 4. Crossover
- 5. Mutation
- 6. Effectiveness
- 7. Parameter Tuning
- 8. Black-Box Function
- 9. Combinatorial Optimization ā Binary Gene Encoding
- 10. Combinatorial Optimization ā Ordered Gene Encoding
- 11. Other Common Problems
- 12. Adaptive Genetic Algorithm
- 13. Improving Performance
- Index