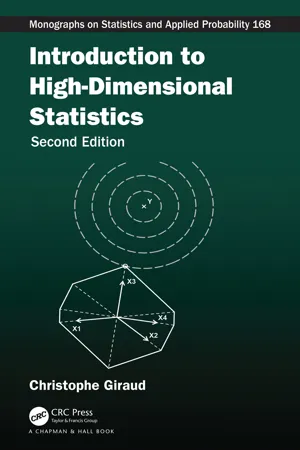
- 346 pages
- English
- ePUB (mobile friendly)
- Available on iOS & Android
Introduction to High-Dimensional Statistics
About This Book
Praise for the first edition:
"[This book] succeeds singularly at providing a structured introduction to this active field of research. … it is arguably the most accessible overview yet published of the mathematical ideas and principles that one needs to master to enter the field of high-dimensional statistics. … recommended to anyone interested in the main results of current research in high-dimensional statistics as well as anyone interested in acquiring the core mathematical skills to enter this area of research."
— Journal of the American Statistical Association
Introduction to High-Dimensional Statistics, Second Edition preserves the philosophy of the first edition: to be a concise guide for students and researchers discovering the area and interested in the mathematics involved. The main concepts and ideas are presented in simple settings, avoiding thereby unessential technicalities. High-dimensional statistics is a fast-evolving field, and much progress has been made on a large variety of topics, providing new insights and methods. Offering a succinct presentation of the mathematical foundations of high-dimensional statistics, this new edition:
-
- Offers revised chapters from the previous edition, with the inclusion of many additional materials on some important topics, including compress sensing, estimation with convex constraints, the slope estimator, simultaneously low-rank and row-sparse linear regression, or aggregation of a continuous set of estimators.
-
- Introduces three new chapters on iterative algorithms, clustering, and minimax lower bounds.
-
- Provides enhanced appendices, minimax lower-bounds mainly with the addition of the Davis-Kahan perturbation bound and of two simple versions of the Hanson-Wright concentration inequality.
-
- Covers cutting-edge statistical methods including model selection, sparsity and the Lasso, iterative hard thresholding, aggregation, support vector machines, and learning theory.
-
- Provides detailed exercises at the end of every chapter with collaborative solutions on a wiki site.
-
- Illustrates concepts with simple but clear practical examples.
Frequently asked questions
Information
Chapter 1
Introduction
1.1 High-Dimensional Data
- Biotech data: Recent biotechnologies enable to acquire high-dimensional data on single individuals. For example, DNA microarrays measure the transcription level1 of tens of thousands of genes simultaneously; see Figure 1.1. Next generation sequencing (NGS) devices improve on these microarrays by allowing to sense the “transcription level” of virtually any part of the genome. Similarly, in proteomics some technologies can gauge the abundance of thousands of proteins simultaneously. These data are crucial for investigating biological regulation mechanisms and creating new drugs. In such biotech data, the number p of “variables” that are sensed scales in thousands and is most of the time much larger than the number n of “individuals” involved in the experiment (number of repetitions, rarely exceeding a few hundreds).

- Images (and v...
Table of contents
- Cover
- Half Title
- Title Page
- Copyright Page
- Table of Contents
- Preface, second edition
- Preface
- Acknowledgments
- 1 Introduction
- 2 Model Selection
- 3 Minimax Lower Bounds
- 4 Aggregation of Estimators
- 5 Convex Criteria
- 6 Iterative Algorithms
- 7. Estimator Selection
- 8. Multivariate Regression
- 9. Graphical Models
- 10 Multiple Testing
- 11 Supervised Classification
- 12 Clustering
- Appendix A Gaussian Distribution
- Appendix B Probabilistic Inequalities
- Appendix C Linear Algebra
- Appendix D Subdifferentials of Convex Functions
- Appendix E Reproducing Kernel Hilbert Spaces
- Notations
- References
- Index