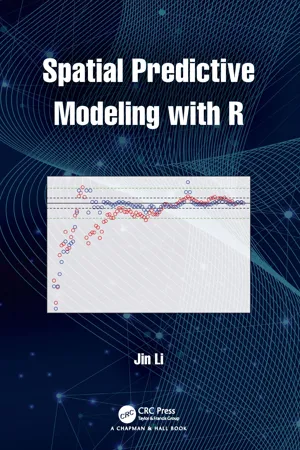
- 416 pages
- English
- ePUB (mobile friendly)
- Available on iOS & Android
Spatial Predictive Modeling with R
About This Book
Spatial predictive modeling (SPM) is an emerging discipline in applied sciences, playing a key role in the generation of spatial predictions in various disciplines. SPM refers to preparing relevant data, developing optimal predictive models based on point data, and then generating spatial predictions. This book aims to systematically introduce the entire process of SPM as a discipline. The process contains data acquisition, spatial predictive methods and variable selection, parameter optimization, accuracy assessment, and the generation and visualization of spatial predictions, where spatial predictive methods are from geostatistics, modern statistics, and machine learning.
The key features of this book are:
â˘Systematically introducing major components of SPM process.
â˘Novel hybrid methods (228 hybrids plus numerous variants) of modern statistical methods or machine learning methods with mathematical and/or univariate geostatistical methods.
â˘Novel predictive accuracy-based variable selection techniques for spatial predictive methods.
â˘Predictive accuracy-based parameter/model optimization.
â˘Reproducible examples for SPM of various data types in R.
This book provides guidelines, recommendations, and reproducible examples for developing optimal predictive models by considering various components and associated factors for quality-improved spatial predictions. It provides valuable tools for researchers, modelers, and university students not only in SPM field but also in other predictive modeling fields.
Dr Li has produced over 100 various publications in spatial predictive modelling, statistical computing, ecological and environmental modelling, and ecology, developed a number of hybrid methods for SPM, and published four R packages for variable selections as well as SPM.
Frequently asked questions
Information
Table of contents
- Cover
- Half-Title
- Title
- Copyright
- Contents
- Preface
- Author Bio
- 1 Data acquisition, data quality control, and spatial reference systems
- 2 Predictive variables and exploratory analysis
- 3 Model evaluation and validation
- 4 Mathematical spatial interpolation methods
- 5 Univariate geostatistical methods
- 6 Multivariate geostatistical methods
- 7 Modern statistical methods
- 8 Tree-based machine learning methods
- 9 Support vector machines
- 10 Hybrids of modern statistical methods with geostatistical methods
- 11 Hybrids of machine learning methods with geostatistical methods
- 12 Applications and comparisons of spatial predictive methods
- Appendix
- References
- Index